From Cost Center to Value Creator
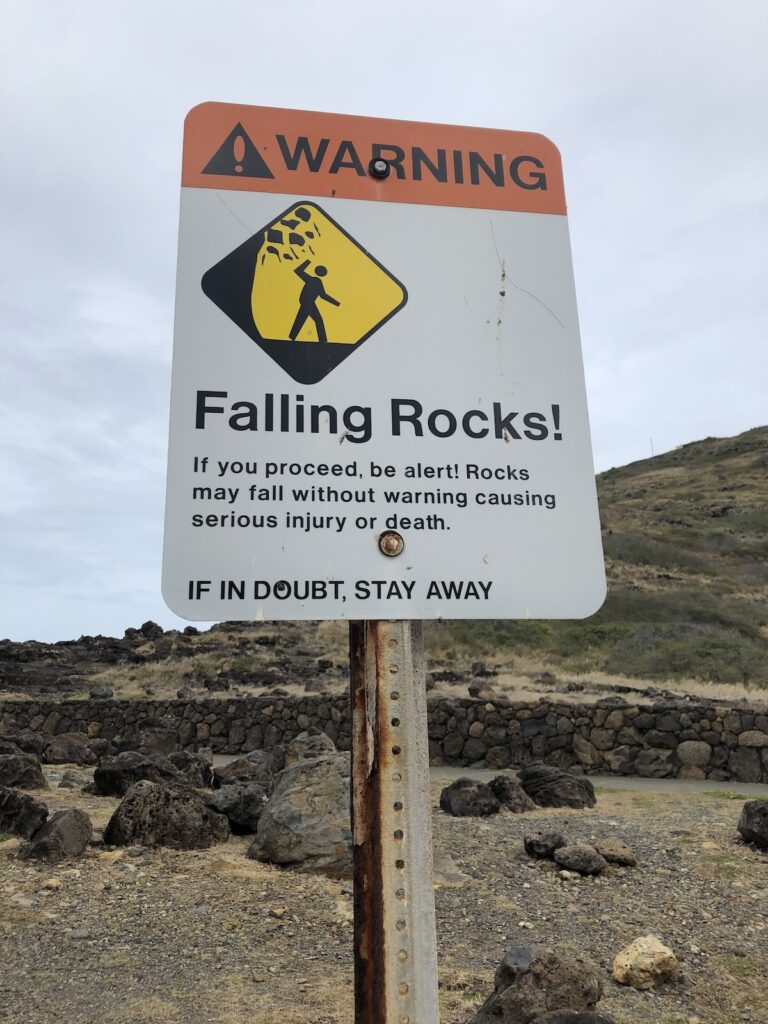
This is part 2 of a 3-part series on AI governance.
- Part 1: Introduces AI governance and reframes the conversation from cost center to value creator
- Part 2: Discusses implementing AI governance for competitive advantage
- Part 3: Outlines the strategic imperatives for successful AI governance
Introduction
In the first installment of my blog series on AI Governance, AI Oversight: Crafting Governance Policies for a Competitive Advantage, I began by reshaping the concept of AI governance–elevating it from a checkbox compliance requirement to a strategic advantage and value creator.[1] I shared real-world examples of inadequate governance frameworks, including the cautionary tale of Air Canada’s confabulating chatbot. I also established that AI governance is essential for maintaining consumer trust and organizational integrity in an age where technology is intricately woven into the fabric of business operations.
Can your AI inadvertently make decisions that expose your company to legal risks?
As we move on to Part 2 of my series, I’ll dive deeper into the operationalization of AI governance. I’ll discuss implementing these frameworks to comply with evolving regulations, drive competitive advantage, and foster innovation. Our focus will shift from defining and understanding the strategic need for AI governance to actionable insights on how to embed these principles into the very DNA of your organization. Join us as we navigate AI governance’s complex yet rewarding landscape, ensuring that your business remains at the forefront of responsible and innovative technology deployment.
AI Governance Journey
Companies gearing up for impending AI regulations often contemplate the ideal starting point. In the 1991 movie What About Bob? starring comedic legend Bill Murray, the story’s protagonist, Bob Wiley, was diagnosed with several phobias. To overcome his fears, his egotistical psychiatrist, Dr. Leo Marvin, prescribes Bob a copy of his book, “Baby Steps,” as a treatment method. When defining “baby steps,” Dr. Marvin states, “It means setting small, reasonable goals for yourself. One day at a time, one tiny step at a time.” Embrace this concept as an organizational journey that your company can navigate one step at a time, one day at a time.
Most organizations progress along the AI governance journey by moving through these steps: initially, starting with a needs-based approach, then moving towards an anticipatory approach, and eventually moving to an integrated strategy, as outlined below.
- Use case based on specific needs: initially, leaders might tackle AI challenges on a case-by-case basis, leading to temporary solutions and regulatory compliance efforts suitable for smaller setups but less effective as complexity increases.
- Strategic, anticipatory approach: the journey progresses towards a deliberate strategy that embraces structured evaluations of risk and reward, consciously choosing to confront more considerable risks directly.
- Evolving to an integrated strategy: ultimately, achieving the highest system benefits requires an interconnected strategy that balances present challenges with an eye on long-term advantages and potential future challenges.
In my article Regulating Generative AI, I outlined the three different risk levels discussed in the EU AI Act.[2] They were:
- Unacceptable risk: prohibits harmful technology that poses threats to humans, including cognitive manipulation, social ranking, facial recognition for surveillance, and autonomous weapons development by militaries globally.
- High risk: the EU classifies AI systems into two categories based on their potential impact on safety and rights. Category one covers AI in retail products like toys and medical equipment under product safety regulations. Category two requires registration in an EU database and includes biometrics, critical infrastructure, education, employment, policing, border control, and legal analysis.
- Limited risk: low-risk AI systems must adhere to transparency standards, notifying users of their interaction with AI according to EU regulations. Models must be law-abiding and disclose the use of copyrighted material in training.
Five Key Governance Questions
Thus, to begin the AI governance journey, the key questions organizations need to answer are:
- What level of risk are we comfortable with when utilizing AI?
- What are the recommended use cases and limitations to manage risks effectively?
- Do the use cases vary between publicly accessible applications like OpenAI’s ChatGPT and custom generative AI models designed for your specific business requirements?
- Who ultimately possesses the authority to decide on the utilization of generative AI?
- What information do we possess that we need to share, and who should we share it with?
Now that we understand the governance journey and critical questions, let’s look at a few real-world use cases.
AI Governance Examples
New York City
New York City’s (NYC) governance approach is as follows:[3]
Figure 1.1: NYC’s AI Governance Approach
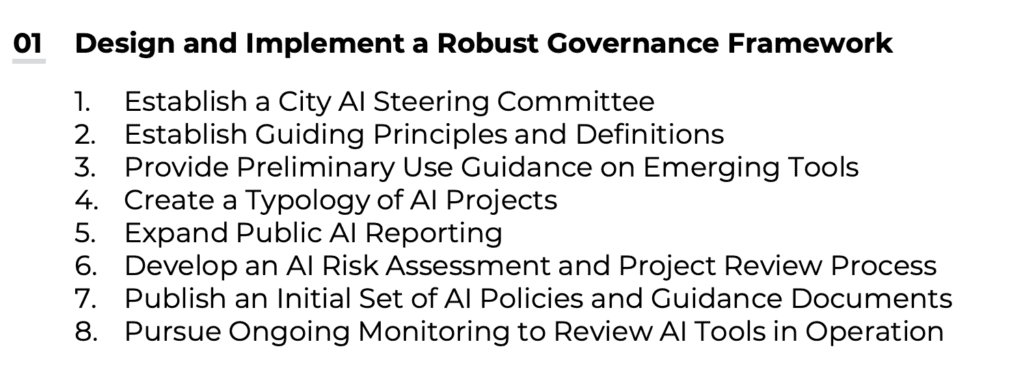
The NYC AI Action Plan outlines eight steps for designing and implementing a robust governance framework, which are also outlined below.
- Establish a city AI steering committee: establish a committee to oversee and provide input on AI initiatives. Within three months, the Steering Committee will draft a charter outlining its scope, guiding principles, membership, operational procedures, and collaboration with city AI projects.
- Establish guiding principles and definitions: define clear objectives and guiding principles to ensure responsible AI implementation across agencies within the city’s governance framework within three months. Leverage established national and international frameworks to inform these efforts.
- Provide preliminary use guidance on emerging tools: within three months, agencies will be given guidance on the uses and risks of emerging AI, starting with generative AI tools like large language models.
- Create a typology of AI projects: Within six months, create a typology of AI projects that reflects the variety of technologies and uses that may fall under the umbrella term “AI” for the city. The resulting typology can be used to inform governance efforts, clarify agency support needs, and enhance public engagement and understanding.
- Expand public AI reporting: within six months and thereafter, improve AI reporting guidelines for agencies to raise public awareness of AI initiatives citywide. This involves expanding the reporting scope, adding model and performance requirements, and ensuring explainability guidelines. Reports will be accessible to the public via the Open Data platform.
- Develop an AI risk assessment and project review process: within twelve months, develop a process for analyzing existing & proposed AI projects, covering key considerations like reliability, fairness, bias, accountability, transparency, data privacy, cybersecurity, & sustainability. Regularly update the assessment model & process, aligning with citywide policies for privacy & cybersecurity.
- Publish an initial set of AI policies and guidance documents: within one to two years, create initial policies and guidance for responsible AI use with input from the Steering Committee, drawing from established frameworks like NIST AI Risk Management and GAO AI Accountability. Prioritize and release policies and guidance incrementally.
- Pursue ongoing monitoring to review AI tools in operation: within one to two years, review AI tools at various project stages per the Project Review Process. Use metrics to gauge impact and ensure alignment with the city’s AI principles. Post-implementation reviews evaluate the tool’s effectiveness, goal achievement, and project enhancement.
Next, let’s look at how Boston is approaching AI.
City of Boston
Similar to NYC, the City of Boston also has a set of interim guidelines on the use of generative AI.[4] Both are based on the National Institute of Standards (NIST) Trustworthy and Responsible AI principles.[5]
Boston’s principles include:[6]
- Empowerment: encourage the effective use of AI to enhance city services and operations.
- Inclusion and respect: ensure AI technologies respect all individuals and communities, promoting inclusivity.
- Transparency and accountability: be open about AI use and accountable for its impact on public services.
- Innovation and risk management: balance innovation with cautious risk assessment and management.
- Privacy and security: protect sensitive information and uphold privacy standards in AI applications.
- Public purpose: align AI use with the public interest, focusing on the community’s welfare.
The City of Boston’s Guidelines for AI use are as follows:
- Fact-check and review AI-generated content: this is especially important for public communication or decision-making to ensure accuracy and avoid disseminating outdated or fabricated information. The responsibility lies in verifying AI-generated content through independent research.
- Disclose AI usage: Transparency about using AI to generate content, including the model version and type, is crucial. This practice builds trust and assists in identifying any potential errors in the AI-generated content.
- Protect sensitive Information: Avoid including sensitive or private information in AI prompts to prevent unintentional sharing through AI systems.
These guidelines encourage responsible AI experimentation while minimizing risks. They ensure that AI technologies are applied in a way that aligns with the city’s values of transparency, accuracy, and privacy protection.
NIST AI Risk Management Framework
As mentioned, NYC and Boston used the NIST AI Risk Management Framework (RMF) to shape their policies. The NIST AI RMF core is represented below.[7]
Figure 1.2: NIST AI Risk Management Framework for AI Governance
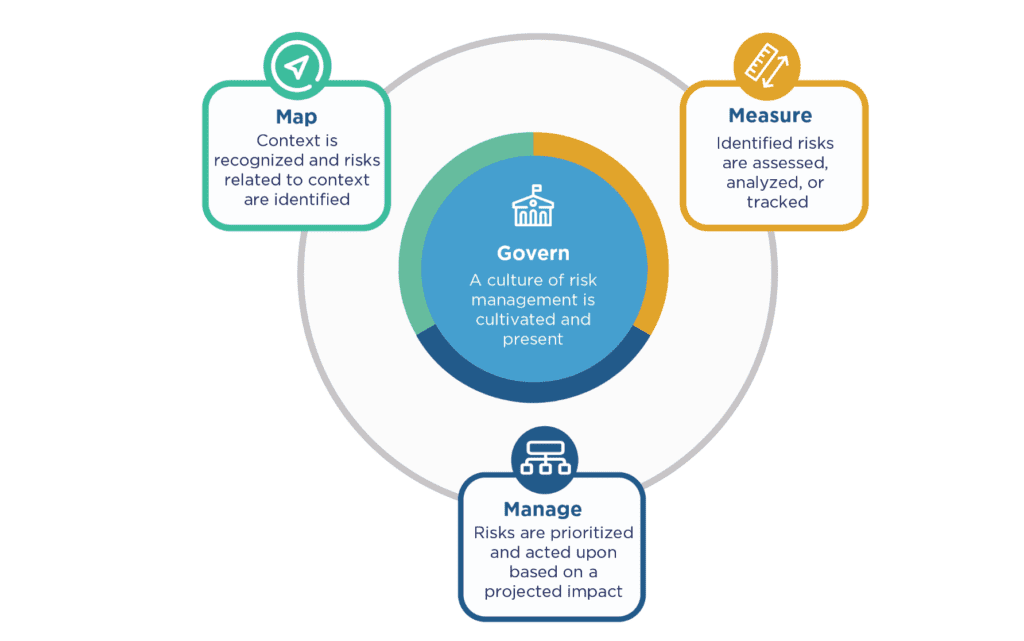
The NIST AI RMF Core is built on four key pillars: Govern, Map, Measure, and Manage. These pillars act as a blueprint for organizations to navigate AI risks and foster reliable AI systems with a systematic strategy. Within each pillar lie various categories and subcategories, offering a clear roadmap for organizations to adhere to. The Core underscores the importance of continual and thorough risk management throughout the AI system lifecycle, integrating diverse viewpoints in a comprehensive approach to AI risk mitigation.
The governance components within the NIST AI RMF core include:
- Cultivating a culture of risk management: developing a culture within organizations that focuses on managing risks throughout the lifecycle of designing, developing, deploying, evaluating, or acquiring AI systems.
- Outlining processes and documents: creating documents and organizational schemes to anticipate, identify, and manage the risks posed by AI systems to users and society, including detailed procedures for achieving these outcomes.
- Incorporating impact assessment processes: embedding processes within the organization to assess the potential impacts of AI systems.
- Aligning with organizational principles: providing a structure for AI risk management functions to align with the organization’s principles, policies, and strategic priorities.
- Connecting technical aspects to organizational values: ensuring that the technical aspects of AI system design and development are connected to the organization’s values and principles, supporting practices and competencies for individuals involved in the AI lifecycle.
- Addressing the Full Product Lifecycle: covering the entire product lifecycle and related processes, including legal and other issues concerning the use of third-party software or hardware systems and data
Within the NIST govern function, organizations must consider several categories and subcategories. They are as follows:
GOVERN 1: The organization’s policies, processes, procedures, and practices related to AI risks are established, transparent, and effectively implemented.
- Understanding, managing, and documenting legal and regulatory requirements.
- Integrating trustworthy AI characteristics into organizational standards.
- Establishing risk management activities based on organizational risk tolerance.
- Establishing transparent risk management processes and outcomes.
- Ongoing monitoring and review of the risk management process.
- Inventorying AI systems according to organizational risk priorities.
- Decommissioning AI systems safely without increasing risks.
GOVERN 2: Accountability structures ensure appropriate teams are empowered, responsible, and trained.
- Clear documentation and communication of roles and responsibilities
- Training personnel and partners in AI risk management.
- Executive leadership taking responsibility for AI-related decisions.
GOVERN 3: Prioritizing workforce diversity, equity, inclusion, and accessibility in managing AI risks.
- Decision-making is informed by a diverse team.
- Policies and procedures for human-AI configurations and oversight.
GOVERN 4: Commitment to a culture that critically assesses and communicates AI risks.
- Fostering a critical and safety-first mindset.
- Documenting and communicating potential impacts of AI.
- Enabling testing, incident identification, and information sharing.
GOVERN 5: Establishing robust engagement processes with relevant AI actors.
- Collecting and integrating feedback on AI’s societal impacts.
- Regularly incorporating feedback into system design and implementation.
GOVERN 6: Addressing risks and benefits associated with third-party software, data, and
- Policies for managing third-party risks, including intellectual property.
- Contingency processes for high-risk third-party failures.
These categories and subcategories provide a framework for implementing effective governance in AI risk management, emphasizing transparency, accountability, diversity, and engagement with relevant stakeholders.
Implementing AI Governance for Competitive Advantage
Building Trust Through Transparency and Ethics
For AI-driven companies, trust is the currency that drives customer loyalty and business success. How an organization approaches AI ethics and transparency can significantly influence public perception and market position. By being transparent and adopting ethical AI practices, companies can better align with regulatory frameworks and build a deeper level of trust with their customers.
Several organizations have demonstrated that prioritizing transparency and ethics in AI can lead to enhanced customer trust and loyalty. For example:
- Salesforce has made ethical AI a cornerstone of its business model, implementing comprehensive guidelines that govern the development and use of AI across its products. By prioritizing user-centric design and AI ethics, Salesforce has strengthened its reputation as a trustworthy software provider.[8]
- IBM has invested in transparency and explainability features for its Watson AI, offering tools that allow users to understand how AI models make decisions.[9] This commitment to open AI has enhanced IBM’s brand image and fostered greater customer engagement.
Recommended Actions for Building Trust
To replicate the success of these companies and build trust through transparency and ethics, business leaders can take the following steps:
- Develop transparent AI policies: create clear, comprehensive policies that outline how AI technologies are developed, deployed, and used within the organization. These policies should cover data privacy, algorithmic fairness, and accountability mechanisms.
- Engage customers in dialogue about AI ethics: Open communication channels with customers to discuss ethical considerations and the steps the organization is taking to ensure responsible AI use. This can include customer forums, feedback sessions, and educational content explaining AI technologies’ ethical dimensions.
By embracing transparency and ethics in AI governance, organizations can transform AI from a potential source of skepticism into trust and loyalty.
Differentiating Through Responsible Innovation
Responsible innovation has emerged as a critical factor for differentiating brands as AI becomes more integrated into products and services. Companies prioritizing responsible AI practices are seen as leaders in ethical business practices. This dual reputation can significantly enhance their appeal to consumers, partners, and investors who are increasingly conscious of the ethical implications of AI.
Responsible AI as a Catalyst for Innovation
Responsible AI refers to developing and using AI technologies in a way that is ethical, transparent, and aligned with human values. This approach to AI innovation focuses on creating solutions that are not only technologically advanced but also socially beneficial and environmentally sustainable. By embedding ethical considerations into the core of AI development processes, companies can unlock new opportunities for creating products and services that address unmet needs and emerging societal challenges.
Examples of Innovation Through Responsible AI
- Accenture has developed an AI fairness tool that helps businesses ensure their AI systems are free from bias, promoting fairness and inclusivity. This tool addresses a critical ethical issue in AI and serves as an innovative product that differentiates Accenture in the consulting and technology services market.
- DeepMind’s AlphaFold, an AI solution for predicting protein structures, is an exciting innovation with vast implications for medical research and drug discovery. AlphaFold’s development was guided by responsible AI principles, including research transparency and collaboration with the scientific community, setting a new standard for beneficial and open AI innovation.
Recommended Actions for Leaders
- Invest in ethical AI research: allocate resources to research and development initiatives focused on ethical AI. This includes funding projects that aim to solve technical challenges like bias and transparency in AI algorithms, as well as interdisciplinary research that explores the broader societal implications of AI. Investments in ethical AI research can lead to the development of innovative technologies that not only advance the field but also resonate with societal values.
- Develop products with AI ethics as a Unique Selling Proposition (USP): leverage the ethical aspects of AI as a key differentiator for products and services. This could mean marketing AI-driven solutions prioritizing privacy, fairness, and user agency, appealing to consumers and businesses looking for trustworthy technology partners. By positioning AI ethics as a USP, companies can tap into a growing market segment that values sustainability, social responsibility, and ethical innovation.
Through responsible innovation, businesses can lead with values that resonate deeply with contemporary consumers and stakeholders.
Achieving Regulatory Excellence Ahead of the Curve
AI regulatory landscapes are continuously adapting to address new technologies’ ethical, privacy, and security challenges. For companies operating in this dynamic environment, merely complying with existing regulations can be a moving target. However, organizations that aim to exceed these baseline requirements can safeguard their operations against future regulatory shifts and position themselves as industry leaders and influencers in the AI space. By progressing through the AI governance journey, companies can move from a reactionary approach to an integrated approach to stay ahead of the curve.
The Strategic Advantage of Regulatory Excellence
Regulatory excellence involves going beyond compliance to anticipate and address potential regulatory challenges before they become mandatory requirements. This approach demonstrates a company’s commitment to responsible AI practices and building trust with customers, regulators, and the public. Moreover, by setting higher standards for AI governance, companies can differentiate themselves in a crowded market, showcasing their leadership in ethical innovation and responsible business practices.
Examples of Proactive Regulatory Engagement
- IBM has advocated for the regulation of AI, emphasizing the importance of tailoring rules to specific AI applications rather than adopting a one-size-fits-all approach. By actively participating in policy discussions and contributing to developing industry standards, IBM ensures its AI practices are ahead of regulatory curves and influences how these regulations are shaped.
- Microsoft has established itself as a leader in AI ethics and regulation by calling for government action on facial recognition technology and implementing its own rigorous internal standards. This proactive stance prepares Microsoft for future regulatory changes and positions the company as a responsible innovator in the eyes of stakeholders.
Recommended Actions for Achieving Regulatory Excellence
- Engage with regulators: establish open lines of communication with regulatory bodies and government agencies overseeing AI technologies. By engaging in dialogue with regulators, companies can gain insights into upcoming legislative trends, contribute expertise to the regulatory process, and advocate for fair and effective policies. This engagement can also provide opportunities to pilot new regulatory frameworks in a controlled environment, demonstrating leadership and commitment to responsible AI.
- Participate in industry forums on AI governance: Join or form coalitions, working groups, and forums dedicated to AI governance and ethics. Participation in these industry-wide conversations allows companies to collaborate with peers, share best practices, and collectively influence the development of standards and regulations. By contributing to the discourse on AI governance, companies can help shape the ethical and regulatory frameworks that will govern the future of AI, ensuring these standards are both practical and beneficial for the industry and society at large.
By developing an integrated AI governance approach and actively engaging in shaping future regulations, companies can not only navigate the complexities of the AI landscape more effectively but also establish themselves as leaders in the responsible development and use of AI technologies.
Practical Advice and Next Steps
- Answer the five key governance questions: begin your journey by answering the five key governance questions identified in the first part of this article, which includes defining your risk tolerance.
- Form and fund a team: Effective AI governance can be a significant competitive advantage for your company, yet it does not materialize on its own. Your organization must empower a dedicated team to grasp the intricacies of the field and proactively implement strategies.
- Take baby steps: As previously mentioned, AI governance is a journey. It starts with taking baby steps to begin the process. Don’t wait, or your company may not achieve the competitive advantage—don’t let governance become another check-list compliance item.
Summary
In integrating generative AI into business operations, leaders must navigate the transformative potential of these technologies with strategic insight and ethical consideration. The journey begins with a thorough assessment of existing AI capabilities, emphasizing the need to establish a comprehensive ethical framework that addresses legal risks, data privacy, and bias. This approach mitigates potential legal exposures—echoing concerns about AI’s decision-making—and aligns AI applications with organizational values. Investing in team training ensures that employees are equipped to utilize AI technologies effectively, maximizing benefits while adhering to ethical standards. This holistic strategy positions businesses to leverage generative AI as a competitive advantage, ensuring innovation is both responsible and aligned with long-term objectives. Following the guidance outlined in this article will mitigate the risks associated with AI decisions and reduce the chance of being in legal hot water.
If you enjoyed this article, please like it, highlight interesting sections, and share comments. Consider following me on Medium and LinkedIn.
Please consider purchasing my latest TinyTechGuide:
Generative AI Business Applications: An Exec Guide with Life Examples and Case Studies.
If you’re interested in this topic, check out TinyTechGuides’ latest books, including The CIO’s Guide to Adopting Generative AI: Five Keys to Success, Mastering the Modern Data Stack, or Artificial Intelligence: An Executive Guide to Make AI Work for Your Business.
[1] Sweenor, David. 2024. “AI Oversight: Crafting Governance Policies for a Competitive Advantage.” Medium. February 25, 2024. https://medium.com/@davidsweenor/ai-oversight-crafting-governance-policies-for-a-competitive-advantage-80bd12a99293.
[2] Sweenor, David. 2023. “Regulating Generative AI.” Medium. August 8, 2023. https://medium.com/towards-data-science/regulating-generative-ai-e8b22525d71a.
[3] “The New York City Artificial Intelligence Action Plan.” 2023. https://www.nyc.gov/assets/oti/downloads/pdf/reports/artificial-intelligence-action-plan.pdf.
[4] Garces, Santiago. 2023. “City of Boston Interim Guidelines for Using Generative AI.” https://www.boston.gov/sites/default/files/file/2023/05/Guidelines-for-Using-Generative-AI-2023.pdf.
[5] “Trustworthy and Responsible AI.” 2022. NIST, July. https://www.nist.gov/trustworthy-and-responsible-ai.
[6] Garces, Santiago. 2023. “City of Boston Interim Guidelines for Using Generative AI.” https://www.boston.gov/sites/default/files/file/2023/05/Guidelines-for-Using-Generative-AI-2023.pdf.
[7] Tabassi, Elham. 2023. “AI Risk Management Framework.” Artificial Intelligence Risk Management Framework (AI RMF 1.0), January. https://doi.org/10.6028/nist.ai.100-1.
[8] Goldman, Paula. 2023. “How Salesforce Develops Ethical Generative AI from the Start.” Salesforce. September 20, 2023. https://www.salesforce.com/news/stories/developing-ethical-ai/.
[9] “What Is AI Governance? | IBM.” n.d. Www.ibm.com. https://www.ibm.com/topics/ai-governance.