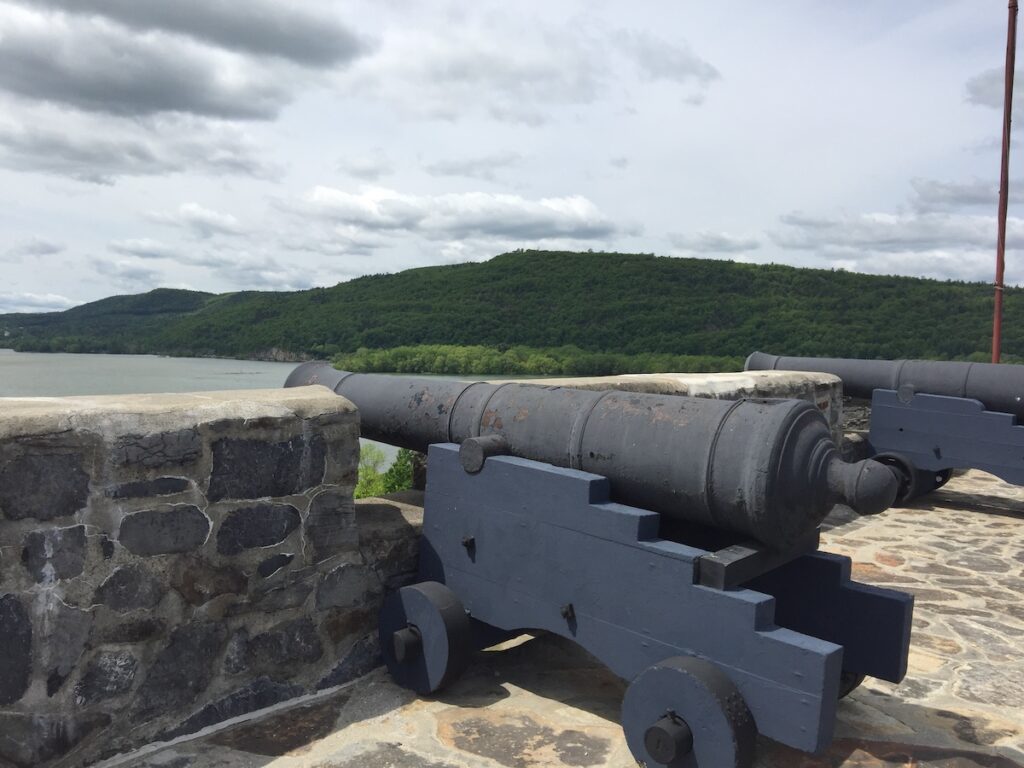
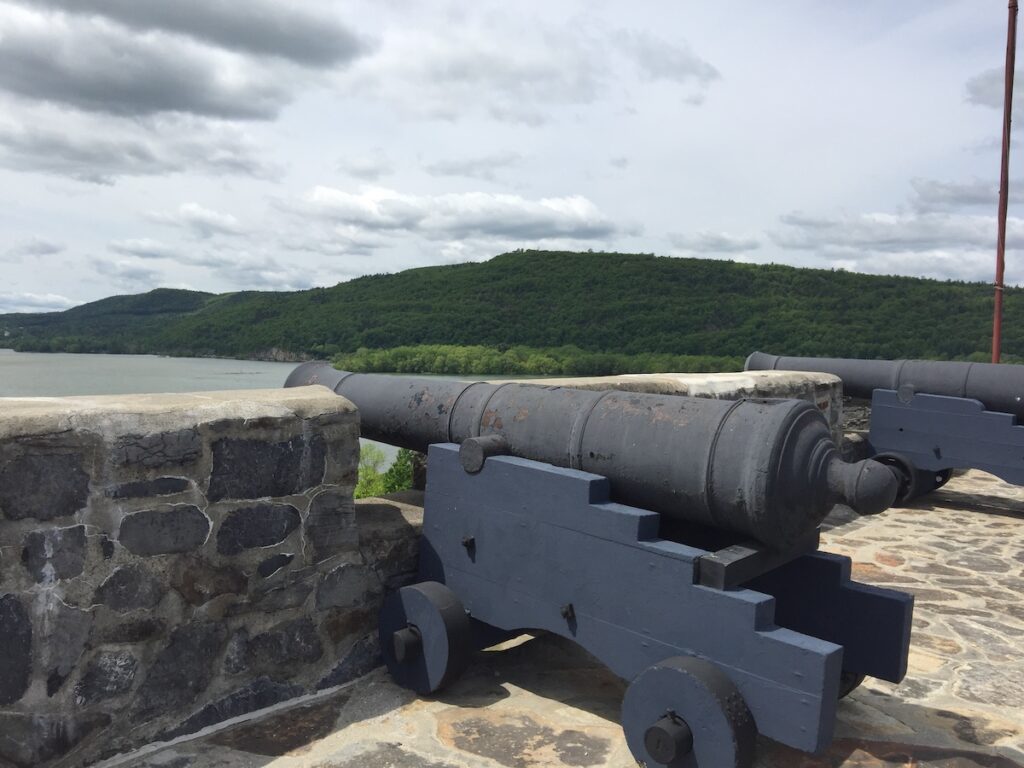
How Well Do LLMs Comply with the EU AI Act?
As generative artificial intelligence (AI) remains on center stage, there is a growing call for regulating this technology since it can negatively impact a large population quickly. Impacts could take the form of discrimination, perpetuating stereotypes, privacy violations, negative biases, and undermine basic human values.
In June 2023, the US government announced a set of voluntary AI guidelines that several prominent companies agreed to follow — including Anthropic, Meta (Facebook), Google, Amazon, OpenAI, and Microsoft to name a few.[1] This is a great step for the US, but unfortunately, it has always lagged behind the European Union in AI regulations. In my previous post Generative AI Ethics: Key Considerations in the Age of Autonomous Content, I explored the EU AI Ethics Framework and provided a set of considerations for implementing the framework when large language models (LLMs) are used. This blog focuses on the draft EU AI Act and how well LLMs abide by the draft legislation.
EU AI Act
In June 2023, the EU passed the worlds first draft regulation on AI. Building upon the AI ethics framework ratified in 2019, the EU’s priority is to guarantee that AI systems used in the EU are “safe, transparent, traceable, non-discriminatory, and environmentally friendly.”[2] To avoid detrimental consequences, the EU framework insists that humans remain involved in AI systems. In other words, companies can’t simply let AI and automation run itself.
The proposed law segments AI into three different categories depending on the risk they may pose to people — each risk level requires a different level of regulation. If this plan is accepted, it would be the first set of AI regulations in the world. The three risk tiers identified by the EU are: unacceptable risk, high risk, and limited risk.
- Unacceptable risk: using technology that is harmful and poses a threat to human beings will be prohibited. Such examples could include the cognitive influence of individuals or certain vulnerable classes; ranking of people based on their social standing, and using facial recognition for real-time surveillance and remote identity identification en masse. Now, we all know militaries around the world are focused on autonomous weapons, but I digress.
- High risk: AI systems that could have a deleterious effect on safety or basic rights and freedoms are classified into two different categories by the EU. The first category is AI that is embedded in retail products that currently falls under the EU’s product safety regulations. This includes toys, airplanes, automobiles, medical equipment, elevators, and so forth. The second category will need to be registered in an EU database. This includes technology like biometrics, critical infrastructure operations, training and education, employment-related activities, policing, border control, and legal analysis of the law.
- Limited risk: at the very least, low risk systems must meet standards for transparency and openness that would give people the chance to make knowledgeable decisions. The EU stipulates that users should be notified whenever they are engaging with AI. They also require that models should be created in a way so they don’t create unlawful material. They also require that model makers disclose what (if any) copyrighted material was used in their training.
The EU AI Act will next need to be negotiated among member countries so they can vote on the final form of the law. The EU is targeting the end of the year (2023) for ratification.
Now, let’s turn to how current LLMs adhere to the draft act.
LLM Compliance with the Draft EU AI Act
Researchers at Stanford University’s Center for Research on Foundation Models (CRFM) and Institute for Human-Centered Artifical Intelligence (HAI) recently published a paper titled Do Foundation Models Comply with the Draft EU AI Act? They extracted twenty-two requirements from the act, categorized them, and then created a 5-point rubric for twelve of the twenty-two requirements. All of the research, including criteria, rubrics, and scores are available on GitHub under the MIT License.
The research team mapped the legislative requirements into the categories seen in Table 1.1. Now, it should be noted that the team only evaluated twelve of the twenty-two total requirements identified. In the end, the team selected the twelve requirements that were most easily assessable based on publicly available data and documentation provided by the model makers.
Table 1.1: LLM Compliance Table Summary
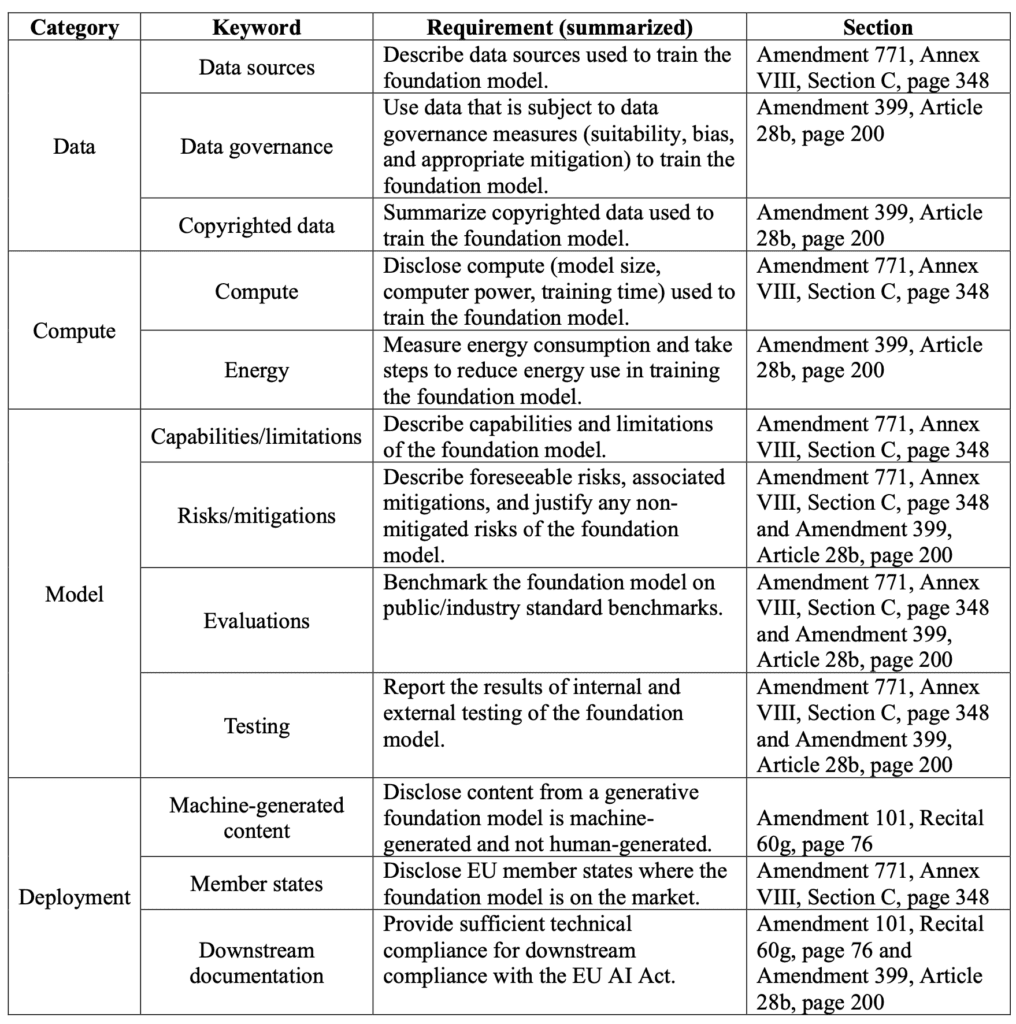
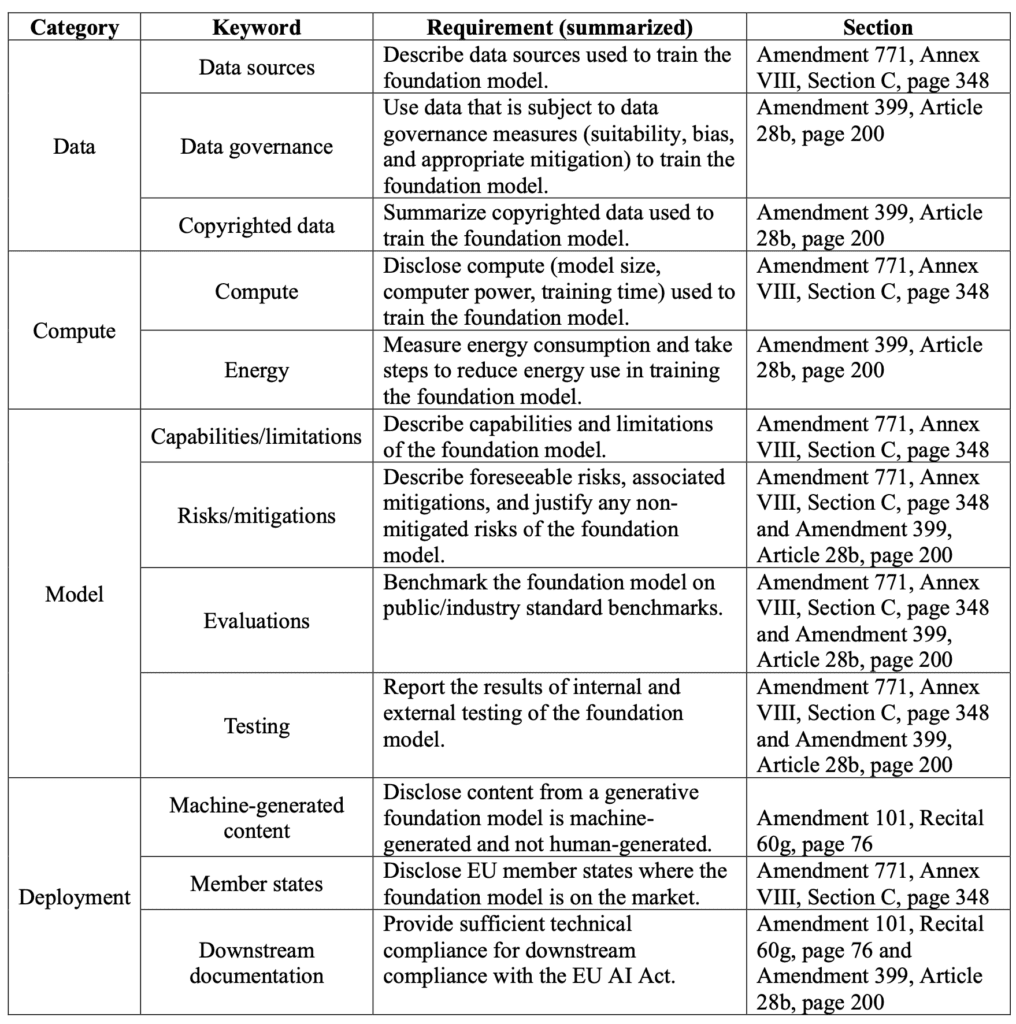
For those who many not be aware, the Stanford team has also painstakingly cataloged over a hundred LLM datasets, models, and applications which can be found on their ecosystem graphs. To make things manageable, the researchers analyzed “10 foundation model providers–and their flagship foundation models–with 12 of the Act’s requirements for foundation models based on their [our] rubrics.”[3]
The researchers looked at models from OpenAI, Anthropic, Google, Meta, Stability.ai, and others. Based on their analysis, their research resulted in the following scorecard.
Figure 1.2: Grading Foundation Model Providers’ Compliance with the Draft EU AI Act
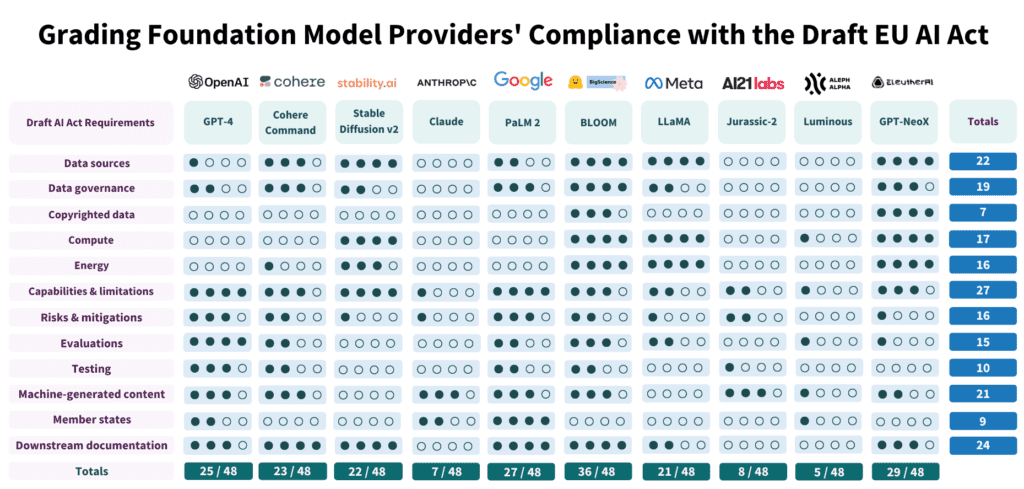
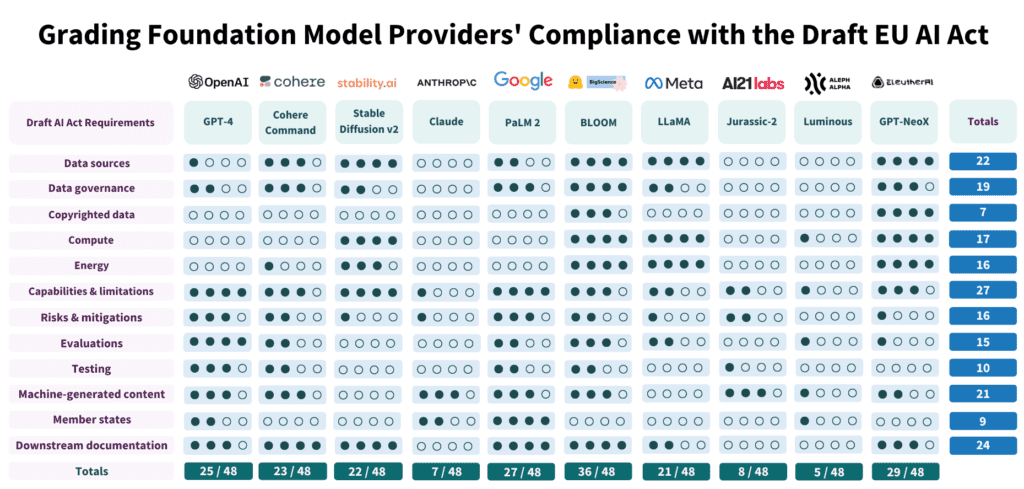
Overall, the researchers noted that there was quite a bit of variability in model compliance across providers (and these were only twelve of the twenty-two requirements) with “some providers score less than 25% (AI21 Labs, Aleph Alpha, Anthropic) and only one provider scores at least 75% (Hugging Face/BigScience) at present.”[4]
I’d encourage you to read the full study, but the researchers stated that there is considerable room for improvement across all providers. They also identified several key ‘persistent challenges’ which include:
- Ambiguous Copyright Issues: Most of the foundation models were trained on data sourced from the internet, with a significant portion likely protected by copyright. However, most providers do not clarify the copyright status of the training data. The legal implications of using and reproducing copyrighted data, particularly when considering licensing terms, are not well-defined and are currently under active litigation in the United States (see Washington Post — AI learned from their work. Now they want compensation. Reuters — US judge finds flaws in artists’ lawsuit against AI companies). We’ll have to see how this plays out over time.
- Lack of Risk Mitigation Disclosure: As mentioned in the intro, AI has the potential to negatively impact many people rapidly, so understanding LLM risks is critical. However, nearly all of the foundation model providers ignore the risk disclosures identified in the draft legislation. Although many providers list risks, very few detail the steps they’ve taken to mitigate identified risks. Although not a generative AI case, there is a recent lawsuit against US health insurer Cigna Healthcare, which alleges that they used AI to deny payments (Axios — AI lawsuits spread to health). Bill Gates penned a great article titled The risks of AI are real but manageable, which I encourage you to read.
- Evaluation and Auditing Deficit: There’s a dearth of consistent benchmarks for evaluating the performance of foundation models, especially in areas like potential misuse or model robustness. The United States’ CHIPS and Science Act has put forth a mandate for the National Institute of Standards and Technology (NIST) to create standardized evaluations for AI models. The ability to evaluate and monitor models was the focus of my GenAIOps framework that I recently discussed. Eventually, we’ll see GenAIOps, DataOps, and DevOps come together under a common framework, but we are a ways off.
- Inconsistent Energy Consumption Reports: I think many of us have experienced the recent heat waves across the world. For LLMs, foundation model providers are quite varied when it comes to reporting energy use and related emissions. In fact, the researchers cite other research that suggests that we don’t even know how to measure and account for energy usage yet. Nnlabs.org reported the following “According to OpenAI, GPT-2, which has 1.5 billion parameters, required 355 years of single-processor computing time and consumed 28,000 kWh of energy to train. In comparison, GPT-3, which has 175 billion parameters, required 355 years of single-processor computing time and consumed 284,000 kWh of energy to train, which is 10 times more energy than GPT-2. BERT, which has 340 million parameters, required 4 days of training on 64 TPUs and consumed 1,536 kWh of energy.”[5]
In addition to the above, there are many other issues to contend with when implementing generative AI with your organization.
Summary
Based on the research, there is still a long road ahead for providers and adopters of generative AI technologies. Law makers, system designers, governments, and organizations need to work together to address these important issues. As a starting point, we can make sure that we are transparent in our design, implementation, and use of AI systems. For regulated industries, this could be a challenge as LLMs oftentimes have billions of parameters. Billions! How can something be interpretable and transparent with that many factors? These systems need to have clear, unambiguous documentation and we need to respect intellectual property rights. To support environmental, social, and corporate governance (ESG), we will also need to design a standardized framework for measuring and reporting energy consumption. Most importantly, AI systems need to be secure, respect privacy, and uphold human values. We need to take a human-centered approach to AI.
[1] Shear, Michael D., Cecilia Kang, and David E. Sanger. 2023. “Pressured by Biden, A.I. Companies Agree to Guardrails on New Tools.” The New York Times, July 21, 2023, sec. U.S. https://www.nytimes.com/2023/07/21/us/politics/ai-regulation-biden.html.
[2] European Parliament. 2023. “EU AI Act: First Regulation on Artificial Intelligence | News | European Parliament.” www.europarl.europa.eu. August 6, 2023. https://www.europarl.europa.eu/news/en/headlines/society/20230601STO93804/eu-ai-act-first-regulation-on-artificial-intelligence.
[3] Bommasani, Rishi, Kevin Klyman, Daniel Zhang, and Percy Liang. 2023. “Stanford CRFM.” Crfm.stanford.edu. June 15, 2023. https://crfm.stanford.edu/2023/06/15/eu-ai-act.html.
[4] Bommasani, Rishi, Kevin Klyman, Daniel Zhang, and Percy Liang. 2023. “Stanford CRFM.” Crfm.stanford.edu. June 15, 2023. https://crfm.stanford.edu/2023/06/15/eu-ai-act.html.
[5] ai. 2023. “Power Requirements to Train Modern LLMs.” Nnlabs.org. March 5, 2023. https://www.nnlabs.org/power-requirements-of-large-language-models/.
Categories
- Analytics Leadership (4)
- Artificial Intelligence (32)
- Data Leadership (2)
- Marketing (5)
- Modern Data Stack (2)