John Thompson on the strategic value of AI agents, the unexpected risks of autonomous systems, and why intelligent governance matters more than ever
Listen now on YouTube | Spotify | Apple Podcasts
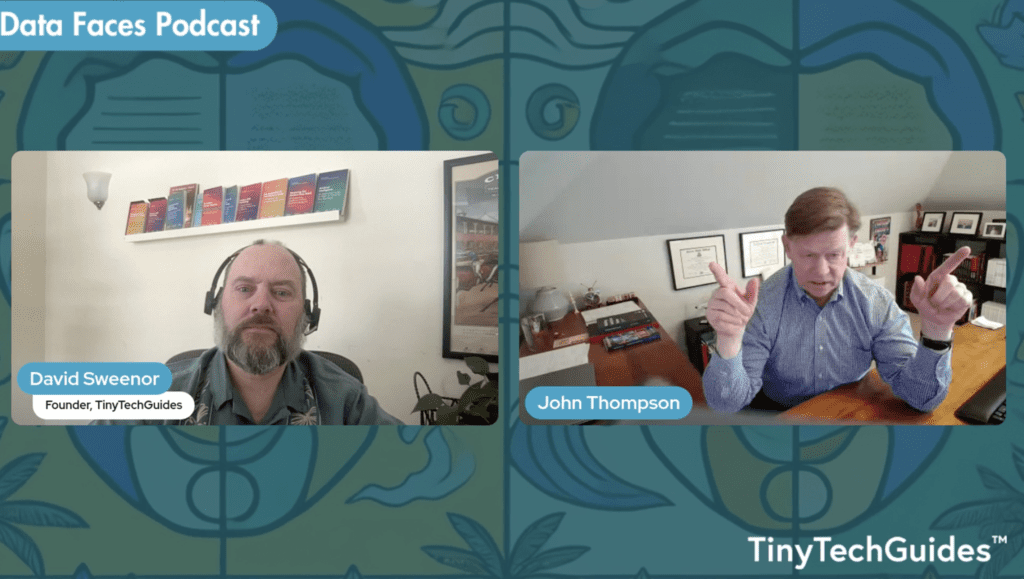
Generative AI is taking us back into the operations. It’s not so much that agents are about analyzing data. They’re about grabbing it, understanding it, doing something with it, transforming it, and then continuing the process. — John Thompson
The architectural leap from foundation models to autonomous agents represents one of the most significant transitions in enterprise AI strategy. While large language models (LLM) operate within a prompt-completion paradigm, agents function through persistent operation across multiple steps with variable outcomes and decision points. This marks a fundamental shift from analytical tools to operational automation that data and analytics leaders must recognize to properly position these technologies within their organization.
The transition introduces new challenges in system design, testing, and governance. While language models operate within the constraints of a single context window, agents maintain state across multiple operations, interact with external systems, and make decisions based on evolving information—creating cascading complexity that traditional AI governance frameworks struggle to address.
About the speaker
John Thompson serves as the Global AI Leader at EY, bringing 38 years of experience in data analytics and artificial intelligence. His career spans from early data warehousing projects to advanced neural networks at IBM, positioning him uniquely to address the strategic challenges of today’s AI agent landscape. Thompson routinely consults with technology leaders at companies including Microsoft and Google, gaining firsthand insight into the direction of autonomous AI systems.
Understanding the Dual Nature of Agent Systems
From an enterprise perspective, agent systems represent a significant departure from both traditional automation and standard LLM applications. While RPA systems follow explicitly coded execution paths, agents operate in probability spaces with emergent decision-making capabilities. This flexibility enables them to handle variable inputs and conditions, but introduces significant governance challenges.
The great thing about AI agents is that you can build them to do pretty much anything a person can do. The problem with AI agents is you can build them to do pretty much anything a person can do. — John Thompson
Thompson highlights a critical conceptual misconception: interpreting this probabilistic behavior as “thinking” rather than properly constraining it as goal-directed behavior with acceptable error boundaries. The strategic challenge lies in designing systems that converge toward optimal solutions while operating within defined parameters—essentially creating guardrails that prevent runaway operations while maintaining flexibility.
The most notorious implementation failures Thompson cites involve unbounded resource consumption—agents continuing execution without appropriate termination conditions, resulting in unexpected compute expenses. He references cases where “young people, very bright people, had built some early stage agents and let them loose… and in the next week or so, they found out that they had run up multi-hundred thousands of dollars compute bills.”
These represent failures of proper goal specification and constraint implementation rather than inherent limitations of agent technology. For data and analytics leaders, this underscores the need for operational guidelines that extend beyond technical parameters into business constraints and acceptable outcome boundaries.
Navigating the Fragmented Agent Framework Landscape
The current proliferation of agent frameworks presents CDAOs with a strategic dilemma: commit to specific technologies now and risk future obsolescence, or wait for market consolidation and potentially fall behind competitors.
There are 150 companies out there with Agent frameworks… that’s not going to last. It’s just like when at the turn of the century there were 100 car companies in America. Now, what are there? Like, seven. — John Thompson
Thompson identifies two dominant strategic approaches currently competing for enterprise adoption:
Microsoft’s Approach: Emphasizes an integrated framework with controlled tool access and structured planning. Thompson characterizes their perspective as “agents are all going to be built on our platform, and we’re going to do this, and it’s all going to be the Microsoft way.”
Google’s Approach: Promotes open interoperability standards with emphasis on framework-agnostic operation. In Thompson’s words, they envision “an open platform. Everybody’s going to be on the cloud, going to build agents in all these different frameworks, and they’re all going to interact… utopian and cool.”
Thompson believes “the reality is going to be somewhere in the middle,” with enterprises making pragmatic choices that often lead to internal fragmentation. He observes that in large organizations like EY, “you’re going to have one division building agents in crew.ai, and another building in AutoGen on Microsoft. They’re going to come together two years later and say, ‘Hey, we’d love our agents to work together.’ And the answer is going to be, ‘They can’t.'”
This organizational reality creates what Thompson calls “technology balkanization” and drives the need for an “agent management platform” that would function as an orchestration layer above individual agent frameworks. He emphasizes that without such coordination mechanisms, enterprises face significant future integration challenges as agent adoption scales across business units.
Implementing Effective Agent Governance
Effective agent governance requires approaches that go beyond simple policy statements. Thompson recommends a comprehensive strategy that surrounds agents with the same contextual knowledge that guides human employees: “You get all the policies and procedures that are relevant. You get all the training materials that are relevant. You get all the information that you would have given to an employee, and you wrap that agent with that information.”
If you build an agent and you wrap it with all the appropriate contextual regulations and policies, agents don’t forget things. Agents don’t willfully not follow the instructions. They will be more compliant than your human employees. — John Thompson
This can be implemented through retrieval-augmented generation systems that dynamically inject relevant policies into the agent’s operational context. Thompson specifically mentions that “you can do it in a standard retrieval-augmented generation, RAG environment. You can ingest it into the context window.”
Properly constrained agents offer a counterintuitive advantage: consistent compliance exceeds what even well-intentioned humans typically achieve. Unlike human employees, agents don’t selectively forget inconvenient policies or deliberately circumvent established procedures. This creates opportunities to reduce certain compliance risks while automating complex processes.
The central paradox of agent governance lies in the human element: the greatest risk factor remains the quality of human decision-making in agent development and deployment. As Thompson notes, “what I’m most concerned about is sloppy thinking by the people that are building” these systems.
For CDAOs, this suggests a governance focus that emphasizes design-time controls rather than solely monitoring agent outputs—addressing the quality of human decisions that shape agent capabilities rather than simply constraining agent behavior after deployment.
Assessing Organizational Maturity in Agent Implementation
Based on conversations with nearly 400 different companies, Thompson observes most organizations remain in experimental stages with AI agents. While generative AI has progressed further along the adoption curve, autonomous agents largely exist as proofs of concept, trials, and minimum viable products rather than production-scale deployments.
We’re in the early days, for sure. As far as generative AI, we’re probably past the knee in the curve, heading up the upward cycle there, and with agents we’re further down. We’re not even at the acceleration curve yet. — John Thompson
Governance approaches vary dramatically across organizations. Thompson describes a spectrum ranging from companies that “have no idea how to govern this stuff, and we haven’t even started to think about it. Therefore, we’re not doing anything” to those with “a well thought out governance process, and we understand how these models work, and we are only putting them in areas that we understand, where there’s low risk, and we’re keeping them under wraps in a way that their processing capabilities are limited.”
This experimental approach necessarily limits value realization. Thompson notes that pilot deployments among small user populations—often 50 people in organizations with hundreds of thousands of employees—represent statistical irrelevance from a value perspective. Significant returns require broader deployment beyond these initial test groups.
For CDAOs, this suggests a staged approach that moves from controlled experimentation to broader deployment as governance capabilities mature. The most effective implementation sequences prioritize internal processes before customer-facing applications, allowing organizations to refine governance approaches in controlled environments where failures have limited consequences.
Establishing Realistic Value Expectations
Thompson identifies a critical pattern in agent value assessment: the tendency toward “utopian automation thinking” that consistently delivers disappointment. Organizations frequently take productivity improvements observed in small pilot groups, multiply by their entire workforce, and project these figures across multiple years—creating projections that bear little resemblance to achievable outcomes.
You see these numbers that can be rather eye-watering in their size, but the value realization often comes down to like 10% of that number. You have to have a very clear-eyed view of what is the utopian possible automation scenario, and then bring it down to value realized. — John Thompson
Reality proves more nuanced. Thompson observes that productivity improvements follow a bell curve distribution across user populations: “Some people are going to get no improvement. Some people are going to get a lot of improvement. And when you bring it towards the middle and actually work it out… the value realization often comes down to, like, 10% of that number.”
This doesn’t diminish the genuine productivity enhancements agents can deliver, but it emphasizes the need for disciplined ROI assessment. Organizations should establish metrics focused on realized value rather than theoretical capability, incorporating adoption rates and usage patterns into their calculations.
For CDAOs, this suggests implementing comprehensive measurement approaches that capture actual usage patterns and business outcomes rather than relying on theoretical productivity calculations. Building these measurement systems into agent deployments from the beginning creates the foundation for data-driven scaling decisions rather than aspirational projections.
Maintaining Transparency in Customer-Facing Implementations
Thompson takes an unambiguous position on transparency in customer-facing agent deployments: “You should let people know that they’re interfacing with an AI.” This represents more than regulatory compliance—it acknowledges fundamental customer expectations around authentic interaction.
There’s no question at all that if you’re injecting AI into your call center operations or customer service processes, or putting it out on the web, you should let people know they’re interfacing with an AI. — John Thompson
He observes that most consumers lack specialized knowledge about AI systems and their capabilities: “A lot of people are not like us. They don’t spend their waking hours thinking about AI and what’s good and what’s bad and what should happen… Most people are just trying to get through their day.” This creates an ethical obligation for transparency, particularly as these technologies become more sophisticated and harder to distinguish from human interactions.
A promising approach involves using AI systems to monitor AI-generated content. Thompson suggests that organizations can deploy verification systems that review outputs from customer-facing agents, ensuring adherence to brand guidelines, appropriate language, and suitable content: “You can set up a model that actually generates this stuff, and then you have another model that ingests it and reads it and listens to it and watches it, then comes back and says, ‘This doesn’t fit with the guidelines.'”
For CDAOs, this creates a strategic imperative: define appropriate boundaries for agent autonomy in customer interactions and implement robust monitoring systems that prevent reputation-damaging failures. This approach balances innovation with appropriate safeguards, enabling progressive expansion of agent capabilities while maintaining organizational control.
Preparing for the Agent-Driven Future
Thompson dismisses notions of a potential “AI winter” or post-AI era—”AI is going to be here forever,” creating an imperative for proactive adaptation rather than wishful thinking about reverting to previous paradigms. When asked about the next AI winter, he responds definitively: “There’s not another AI winter coming. It’s widely adopted now, people are using this stuff.”
There is no post-AI world. If there’s a post-AI world, then the world has ended. AI is going to be here forever. — John Thompson
Workforce implications vary substantially by career stage. Thompson observes that adaptation challenges prove greatest for experienced professionals: “The people who have more experience or have been longer in their career, those are the folks that are going to have challenges with AI.” This creates both a development challenge and a strategic opportunity for organizations that effectively support this transition.
For CDAOs leading agent adoption initiatives, Thompson recommends several resources for organizational learning: “Connect with me on LinkedIn. If you’re in analytics and data and AI field, I’ll connect with you. I post on this stuff on a daily basis.” He also suggests online learning platforms like Coursera for building foundational knowledge, and references his upcoming book “The Path to AGI” (March 10) for deeper understanding of the evolutionary trajectory from current AI systems toward more sophisticated capabilities.
Organizations should approach agent adoption with strategic patience—recognizing the persistent relevance of these technologies while taking measured steps to implement them effectively. As Thompson emphasizes, “AI is going to be here forever,” making thoughtful adoption an organizational necessity rather than a discretionary initiative.
- Check out John’s latest book The Path to AGI: Artificial General Intelligence: Past, Present, and Future on Amazon: https://www.amazon.com/Path-AGI-Artificial-General-Intelligence/dp/1634627016/
- Connect with him on LinkedIn: https://www.linkedin.com/in/johnkthompson/
Transcript Highlights
This edited transcript has been condensed for clarity and readability.
Here’s a condensed, edited version of the transcript with key timestamps and insights that would work well as a supplementary section at the end of your blog:
Key Insights: AI Agents in the Enterprise
Edited transcript highlights from John Thompson’s interview
[00:33] “I’ve been doing this for 38 years now… it just struck me one day that everything that we did and do had something to do with data. And people were always trying to make sense of data and trying to analyze and trying to put it together in different ways.”
[02:40] “AI agents is a big deal… The great thing about AI agents is that you can build them to do pretty much anything a person can do. The problem with AI agents is you can build them to do pretty much anything a person can do.”
[03:45] “We had this happen a couple times over the last couple years where it turned out to be two young people, very bright people, had built some early stage agents and let them loose. And in the next week or so, they found out that they had run up multi-hundred thousands of dollars compute bills.”
[04:58] “We’re in the early days, for sure. As far as generative AI, we’re probably past the knee in the curve, heading up the upward cycle there, and with agents we’re further down. We’re not even at the acceleration curve yet.”
[05:35] “I saw the other day, there are 150 companies out there with Agent frameworks… that’s not going to last. It’s just like when at the turn of the century there were 100 car companies in America. Now, what are there? Like, seven?”
[06:22] “You talk to the people at Microsoft, and it’s like, ‘Agents are all going to be built on our platform’ … Talk to the people at Google like, ‘It’s going to be an open platform. Everybody’s going to be on the cloud, going to build agents in all these different frameworks, and they’re all going to interact.'”
[10:13] “Agents are, you know, intelligent process automation.”
[12:07] “What I’m most concerned about is the people that build it. A lot of the developers and the people that are building these things, the analysts, they’re good folks, but they really have never been let loose on a problem that could have this level of variability in it, this level of complexity.”
[14:28] “We’ve started to design something we’re calling agent management platform.”
[16:54] “If you don’t control these things, and if you don’t think about them in a structured way, your risk is that they’re going to run out of control, or they’re going to interface with companies that you don’t want them to interface, or they’re going to bind you to things that you don’t want to do.”
[18:46] “When you look at an agent, you wrap around that agent everything that you would wrap around an employee… all the policies and procedures that are relevant. You get all the training materials that are relevant.”
[19:25] “Agents are nothing more than software. They’re not people, they don’t have emotions, they don’t have 401Ks, they don’t take time off. The whole idea that we treat software as some kind of sentient being is truly misguided.”
[23:44] “Any use of AI that comes in contact with another person should have some kind of disclaimer or announcement.”
[25:48] “The challenge that I see in most corporations is that people get an idea of a utopian automation scenario… we’re going to extrapolate out against our entire workforce for the next five years. That’s just bad math. That’s just sloppy thinking.”
[27:39] “The fact of the matter is it’s in the hands of 50 people. If you’ve got 200,000 employees, that’s not even a drop in the bucket. So you’re not really going to see this value realization happening until you get it in the hands of a substantial number of the population of your employees.”
[30:53] “The problem that’s created by AI is solved by AI.”
[34:13] “There is no post-AI world. If there’s a post-AI world, then the world has ended.”
[34:33] “If you really feel like you’re not understanding what’s going on, I would start with the tiny tech guides. Another way, self-servingly, is connect with me on LinkedIn. If you’re in analytics and data and AI field, I’ll connect with you.”