Creating a KPI Game Plan
Not everything that can be counted counts and not everything that counts can be counted.
-Albert Einstein
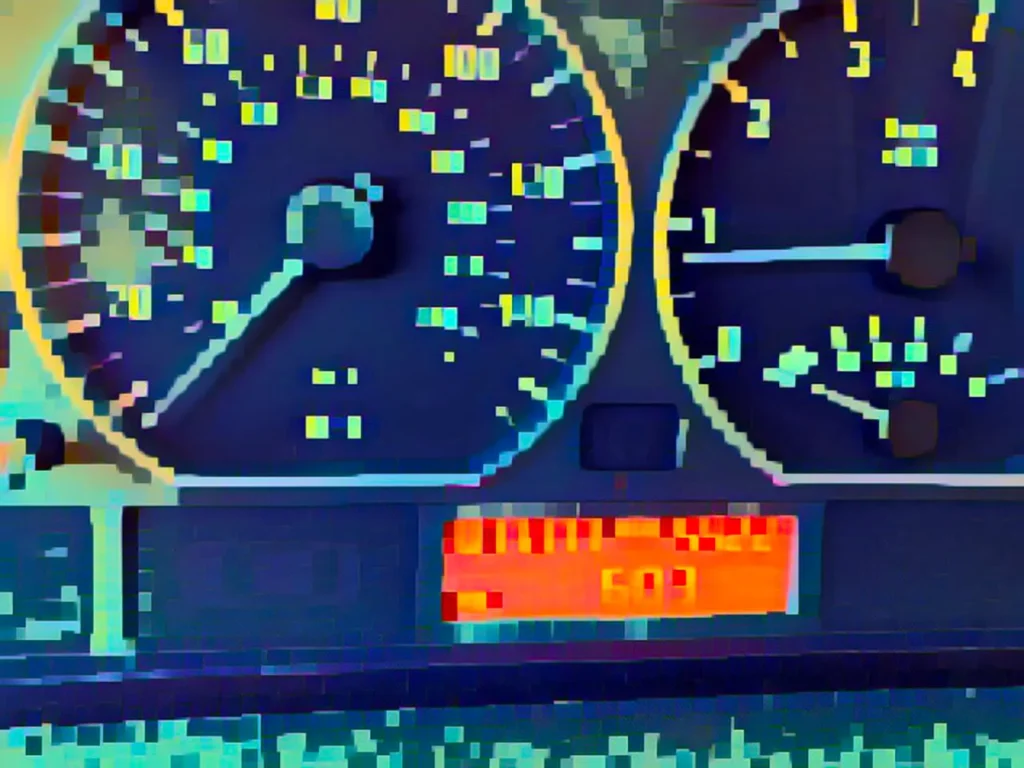
Some say data is the new oil. It’s not, as the World Economic Forum pointed out in 2018. Petroleum is a finite resource, and it takes eons to make more of it. Once the crude is extracted, refined, and consumed, that’s the end of it.
Contrast that with data. The amount of data being created is beyond our wildest dreams — it comes in all shapes and sizes and some firms estimate that it is growing at an exponential rate. In fact, the latest IDC Global DataSphere Forecast suggests that the amount will more than double in size by 2026. The fact of the matter is that the sheer amount of data available is incomprehensible to most — how big is a zettabyte, really? To put this into some perspective, it is estimated that “more than 5 billion customers interact with data every day — by 2025, that number will be 6 billion, or 75% of the world’s population. In 2025, each connected person will have at least one data interaction every 18 seconds.”[1] It is anticipated that there will be 175 ZB of data by 2025. “If you were able to store the entire Global DataSphere on DVDs, then you would have a stack of DVDs that could get you to the moon 23 times or circle the Earth 222 times.” [2] Now that’s a lot of data — but also speaks to the inefficiency of DVDs as a storage mechanism. In fact, a single gram of DNA can store about 215 petabytes (0.000215 zettabytes).[3]
That’s why I prefer to think of data as a renewable resource. Of course, renewable energy involves the costs of installing solar farms, geothermal plants, biomass facilities, hydroelectric dams, and wind turbines. But once they’re in place, the energy released will be available for a long time. Even with ongoing maintenance, the up-front investment will provide continuing value.
That renewability ties into the concepts behind Bill Schmarzo’s book, The Economics of Data, Analytics, and Digital Transformation. Once data is transformed into higher value through analytics, your organization will receive that value in perpetuity.
There’s no limit on how many times and ways you can transform the data — its value will continue to increase.
Here’s why.
Before Thinking About KPIs, Understand Your Game Plan
Before energy companies invest in developing a site, they generally gauge the fit with their corporate strategy. They conduct significant research to ensure that the infrastructure and facilities will have a positive, sustainable, significant return on investment. In the case of wind turbines, important factors include understanding the wind resource (minimum average annual wind speed), access to land and capital, proximity to transmission lines, and zoning regulations.
Similarly, before organizations embark on any analytic and AI initiative, business leaders need a clearly defined business strategy. Good business strategies often give rise to objectives and key results (OKRs). To achieve these OKRs, organizations will have strategic initiatives comprising one or more projects, each of which often requires AI and analytics.
“AI should fuel business strategy.”[4]
For any given project, I always encourage business leaders to understand the business decision to be made, and then work backwards from there. After all, we don’t build wind farms for the sake of it. We construct them to provide value to society and returns to our company and municipality.
Failure to have a clear strategy can be disastrous. When your data isn’t as good as you think it is, you draw the wrong conclusions and waste effort. Or, you can spend so much time collecting data that you miss the window of opportunity. And some companies over invest in their data science team and underinvest in spreading data literacy among their workers.
The conversations we have with our customers start with the end goal. And our customers continually show us the value in conducting due diligence on business processes to see how data and analytics can be used to achieve their business goals. Everything starts with an objective.
Key Performance Indicators for Analytics
With the current economic uncertainty that surrounds us, Chief Data and Analytics Officers are under increasing pressure to track the business impact of data, analytics, and AI initiatives on business outcomes. In most organizations, that leads to talk of key performance indicators (KPIs).
But why should we care about KPIs at all? According to Michael Schrage, a visiting research fellow at the MIT Initiative on the Digital Economy, “When applied to digital transformation efforts, KPIs can drive business strategy and provide a distinct competitive advantage.” [5] Furthermore, Schrage has identified seven fundamental characteristics of enterprises boosting their return on KPIs. They are:
1. KPIs help to lead and manage.
2. KPIs help to align the entire organization.
3. KPIs provide a holistic view of the customer.
4. KPI factors inform decision making.
5. KPI data is shared across business units.
6. KPIs don’t proliferate. Leaders should focus on 3 to 6 KPIs that will drive growth.
7. KPIs serve as data sets for machine learning (ML).
So, which are the best KPIs for your organization to track? Before you can answer that, your business leaders need to answer these questions:
· What do you want to know?
· How much do you need to know?
· Why do you want to know this?
· What is the value or impact between not knowing and knowing?
Once you know the answers to these questions, you can start establishing KPIs to track your success.
Independent of data, analytics and AI, we find that KPIs generally fall across the following dimensions:
· Financial — Revenue growth, cash flow, burn rate, gross profit
· Customer — Engagement rates, net promoter scores, acquisition costs, conversion rates
· Support & Service — Turnaround time, mean time to resolve, SLA compliance, quality
· Employee — Attrition and retention, satisfaction, engagement
· Governance, Risk, & Compliance — Percent compliance to process, audit compliance, non-security incidents
Data & Analytics KPIs
Looking closer, are there any specific KPIs that organizations should track when data, analytics, and AI are used to deliver upon the strategic plan? Perhaps there are.
The analyst firm Gartner recently published a research note titled “5 Data and Analytics KPIs Every Executive Should Track”, [6] describing key performance indicators you can apply to your data, analytics, and AI programs. They include:
· Business impact of data, analytics, and AI
· Time from data creation to insight, and ultimately action
· Data quality
· Data literacy levels
· Data, analytics, and AI risks
The indicators will help you get more out of your AI programs and avoid wasting your data as you try to use it.
1. Track business impact of data and analytics
This is the great defining indicator because it answers the question, “How much has the needle moved because of our efforts in data and analytics?” Almost everybody in the organization wants to know whether the investment in data, analytics, and AI is paying off, and this is the shorthand answer.
But projects can make an impact without making the cash register ring right away. Think about a few different shades of business impact:
· Indirect — You can’t directly link all of the decisions you’re basing on data analytics to bottom-line results, but you may still feel you’re moving in the right direction. Or maybe you haven’t yet discovered the right metrics to link your data-driven decisions to business outcomes; when you discover those metrics, the business impact won’t seem indirect anymore.
· Leading — Some outcomes predict other outcomes. Suppose a low net promoter score among your employees two years ago convinced you to add headcount in Human Resources last year, and productivity is rising this year. A concrete outcome leading another concrete outcome is tangible business impact.
· Anecdotal — You can’t be certain that working from home is the reason your employees are more productive, but many of them are telling you that it is the reason. It’s easy to measure time spent in the office before and during the pandemic. But with few recognized ways of measuring all the variables, you must rely on the anecdotal evidence that working from home is linked to the productivity boost.
· Direct — How close to the scientific method — hypothesize, measure, change variables, measure, conclude — can you get? Online advertising, for example, has vast potential for A/B testing and refining with thousands or millions of data points.
· Touched — When multiple teams, factors, and AI efforts are at work, it becomes difficult to attribute a given outcome to any single element. I like to think about this: How do I measure the value of electricity in my house? Without electricity, I may not see a wall in the dark, not be able to charge my devices, and not be able to binge my favorite shows. But quantifying the ROI of that electricity is quite difficult. Still, both the efforts and the impact are measurable, so, like electricity, you can say confidently that data, analytics, and AI have touched certain outcomes.
Given those different gradations, how do you report on the business impact of data, analytics, and AI? Consider this structure as an example:
· Business impact
· Target total for this fiscal year: $25M vs. $2M actualized YTD
· Progress
· Use case 1: 80% underway, on track
· Use case 2: 20% underway, 3 months’ delay
· Use case 3: 100% complete
2. Monitor time from insight to action
Over time, data can go stale for several reasons: competitors get the drop on you, customer tastes turn on a dime, uncontrollable economic factors come into play.
That’s why the second indicator looks at how quickly your organization can derive insights from data and turn them into action. That agility — where preparation meets opportunity — is your competitive differentiator, whether your point of departure is a clear business question or an unguided hunt for insights hidden in your data.
Here’s guidance for tracking and reporting on the time that elapses between insight and action in your organization:
· Time from insight to action:
· Currently: 45 days
· Target for end of year: 7 days
· Process improvement
· Speeding up approval process: 80% complete
· Improving data literacy: 55% staff trained
· Accelerating IT involvement: 10% complete
3. Measure data quality
“How do we know the data’s any good?”
That’s the logical question to ask anytime you arrive at a new insight through data, analytics, and AI, especially if the insight is unexpected. It can be only as valid as the data on which it is based.
The key to reporting this indicator is not to simply name the data source but to quantify the current trustworthiness of the data and to show the path to improvement:
· Data quality
· Currently: 70% of sales forecast based on unverified data
· Target for EOY: 10%
· Process improvement
· Remove manual reconciliation process (prospect data): 100% complete
· Train data entry associates (customer data): 25% complete
4. Track data literacy levels
When most companies hear “data-driven,” they usually think “technology.” But success in data, analytics, and AI depends more on skills and people than on technology.
That’s why it’s important to track and report on your company’s progress in data literacy across all functions of the enterprise. It’s no secret that professionally trained data scientists are in short supply, so smart companies instead upskill their current workforce to make better-informed decisions more quickly. Training in data literacy shows people how to retrieve, analyze, and derive insights from data, then communicate those insights and convert them to action. Data literacy extends from advanced data scientists to citizen data scientists, and it’s at the heart of digital transformation.
Consider this structure for your reporting of this indicator:
· Data literacy rate
· Currently: 35% of staff have completed the program
· Target for EOY: 100%
· Progress
· Uptake in self-service reporting and dashboards
· Staff survey results on usefulness of their new skills
5. Quantify data, analytics, and AI risk
What’s the chance that one or more of your data, analytics, and AI projects will be misused? What’s your company’s exposure if your work becomes a mess, goes negative in value, and turns into waste?
Sensitive data usually rubs up against regulations and industry standards. If you’re maintaining a warehouse of customer data and somebody forgets to enable encryption, how much time, money, and effort could it cost you in remedies and reputation?
Here’s one perspective on quantifying your risk of exposure:
· Data, analytics, and AI risk
· Currently: $17,000,000 data risk
· Target for EOY: $5,000,000
Progress
· Projects undergoing ethics review: 30%
· Projects undergoing privacy impact assessment: 80%
· Projects undergoing data protection impact assessment: 55%
Conclusion
Data, analytics, and AI are part of your competitive advantage, part of the promise you make to your customers and part of your perceived value to investors and prospects.
Smart leaders focus on managing their company’s data as a strategic resource. The indicators outlined above are designed to keep leaders’ fingers on the pulse of their data and keep it from ever getting messy.
You can use this self-assessment from Harvard Business Review to evaluate your organization’s analytic maturity to help you start planning KPIs for your next project.
Editors Note: A version of this originally appeared on Medium.
References
[1] Reinsel, David, John Gantz, and John Rydning. 2018. “The Digitization of the World from Edge to Core.” https://www.seagate.com/files/www-content/our-story/trends/files/idc-seagate-dataage-whitepaper.pdf.
[2] Ibid.
[3] Service, Robert F. 2017. “DNA Could Store All of the World’s Data in One Room.” www.science.org. March 2, 2017. https://www.science.org/content/article/dna-could-store-all-worlds-data-one-room.
[4] Sweenor, David. 2022. Artificial Intelligence: An Executive Guide to Make AI Work for Your Business TinyTechMedia LLC. https://www.amazon.com/Artificial-Intelligence-Executive-Guide-Business/dp/B09X4KTYS4/.
[5] Eastwood, Brian. 2021. “Digital Business Needs New KPIs. Here’s Why They Matter.” MIT Sloan. May 11, 2021. https://mitsloan.mit.edu/ideas-made-to-matter/digital-business-needs-new-kpis-heres-why-they-matter.
[6] Buytendijk, Frank, and Alan D. Duncan. 2022. “5 Data and Analytics KPIs Every Executive Should Track.” Gartner.com. Gartner Inc. https://www.gartner.com/document/4014751.
Categories
- AI Trends (8)
- Analytics Leadership (9)
- Artificial Intelligence (52)
- Data Faces Podcast (4)
- Data Leadership (2)
- Marketing (17)
- Modern Data Stack (3)
- News (8)
- Tech News (1)
Latest Posts
- Team Dynamics Over Technology: The Human Elements that Drive AI Success
- The Customer Hero Principle: Why Your B2B Messaging Falls Flat
- The Grandmother Test: Building AI Trust Beyond Technology
- Don’t Trust Your AI With Dates: The Calendar Blindspot
- The Gen AI Shift: How Product Marketing Managers Are Adapting