Kamal Maheshwari on solving the data trust crisis, aligning teams, and building AI-ready data ecosystems. View on YouTube: https://youtu.be/U9pq1D2iVRY
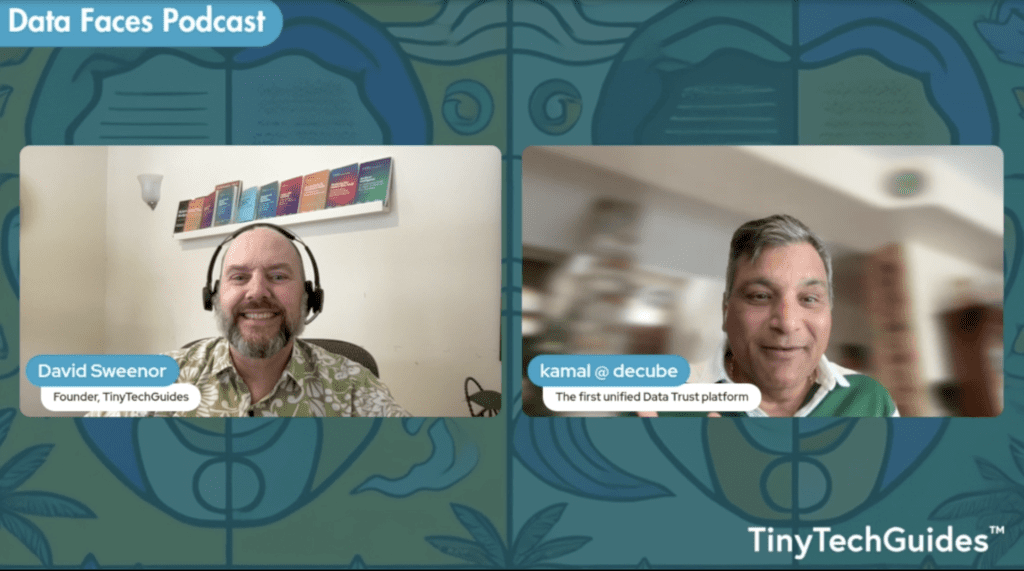
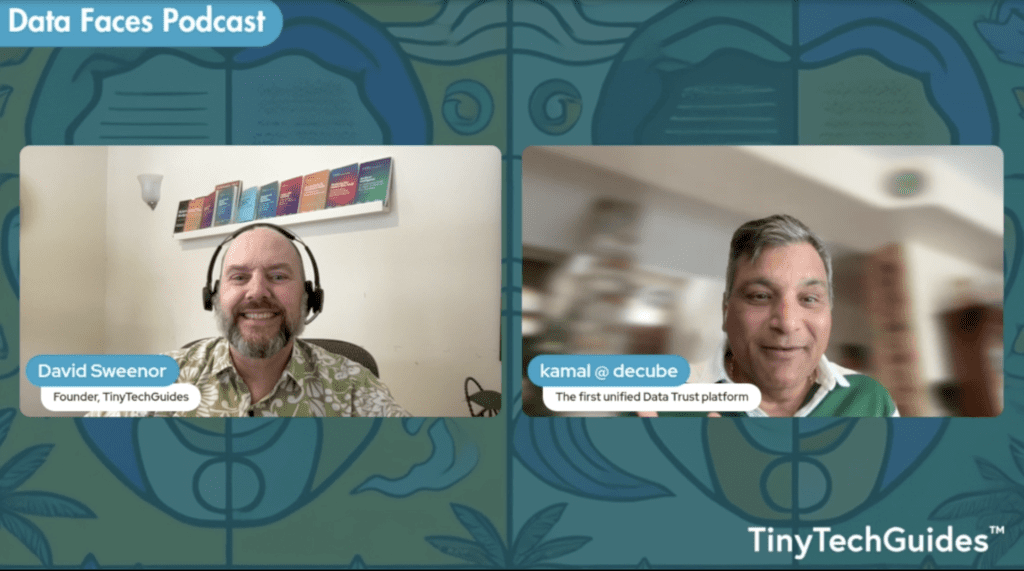
How can you trust the decisions of your AI if you can’t trust the data that powers it?
In Episode 4 of the Data Faces Podcast, host David Sweenor speaks with Kamal Maheshwari, Co-Founder of Decube, about the growing need for trusted data in today’s AI-driven world. Kamal shares actionable insights into how organizations can tackle the data trust crisis and build robust foundations for AI success.
About the Speaker
Kamal Maheshwari is a seasoned entrepreneur and technologist with a deep background in data management. He has worked with leading organizations like Oracle, Cloudera, and Sun Microsystems before co-founding Decube, where he helps businesses unify their data ecosystems to improve trust and drive smarter decisions.
In our conversation, we discuss:
- Why data trust is a cornerstone for AI success
- The biggest challenges organizations face in building trusted data systems
- Practical solutions for business leaders to improve data integrity
- How to quantify trust using data metrics
- Emerging trends in data trust for the AI era
Listen now on YouTube
What is Data Trust?
Data trust refers to the confidence an organization has in its data’s accuracy, consistency, and reliability. Without this trust, even the most sophisticated AI systems are bound to fail, producing unreliable or even harmful outputs.
Kamal Maheshwari explains data trust using a powerful analogy: it’s like the foundation of a building. If the foundation has cracks, the structure above becomes unstable, jeopardizing the entire project. Similarly, without trusted data, AI outputs can’t be relied upon, which risks poor decision-making at every level of the business.
For CEOs and business leaders, data trust isn’t just a technical challenge—it’s the difference between confident decisions and costly mistakes. Trusted data reduces operational risks, accelerates decision-making, and ensures the company can fully leverage AI for competitive advantage.
Challenges in Building Trusted Data Systems
Organizations understand the value of data trust, but achieving it is far from simple. Kamal Maheshwari identifies three primary challenges that hinder progress:
- Legacy Systems and Silos: Outdated infrastructure and fragmented systems result in data inconsistencies, making it difficult to ensure data integrity.
- Disjointed Teams: Governance, quality, and warehousing teams often work in isolation, creating misalignment in data strategies.
- Data Quality Failures: In the AI era, poor data inputs don’t just lead to bad decisions—they can magnify risks and cause significant business disruptions.
These challenges require business leaders to rethink their data ecosystems, prioritize cross-functional collaboration, and address technical gaps that weaken data reliability.
Solutions for Business Leaders
To solve the data trust crisis, organizations must take a dual approach, addressing both technology and leadership:
- Technological Solutions:
Unified data platforms, such as Decube, integrate governance, quality, and cataloging to create a single source of truth. Cloud-native tools offer the scalability and flexibility needed for modern data environments. - Leadership and Organizational Solutions:
Leaders play a critical role in fostering data trust by aligning teams and setting clear accountability. Establishing cross-functional governance committees ensures that data quality and reliability remain top priorities.
Practical Steps for Business Leaders:
- Conduct a thorough data trust audit to identify weaknesses in your current ecosystem.
- Implement data lineage tracking to increase transparency across data pipelines.
- Develop key performance indicators (KPIs) to measure and improve data trust over time.
With these solutions, businesses can turn data trust from an abstract concept into a measurable advantage that supports smarter decision-making and sustained growth.
Quantifying Data Trust
Quantifying data trust allows organizations to monitor the health of their data ecosystems and take proactive measures to address weaknesses. Kamal Maheshwari introduces the concept of a trust score—a composite metric that evaluates data accuracy, freshness, and lineage.
For example, a trust score might drop when inconsistencies in data pipelines arise, signaling a need for immediate investigation. By tracking this score over time, businesses can identify trends, detect potential risks early, and take corrective actions before they affect decision-making.
A measurable trust framework not only enhances data reliability but also builds confidence in AI-driven processes, enabling leaders to make informed decisions with less uncertainty.
Future of Data Trust in the AI Era
As businesses increasingly rely on AI, the importance of trusted data will continue to grow. Kamal Maheshwari highlights three key trends shaping the future of data trust:
- Real-Time Data Validation: Emerging tools enable organizations to verify data accuracy and consistency as it moves through pipelines, reducing delays and errors.
- AI-Driven Governance: Machine learning is automating routine governance tasks, allowing teams to focus on higher-value activities.
- Embedding Trust Metrics into Business KPIs: Companies are beginning to treat data trust as a core performance indicator, aligning it with broader business goals.
For business leaders, keeping pace with these trends is essential. Those who adopt forward-thinking data strategies will gain a competitive edge, while those who don’t risk being left behind in an increasingly AI-driven marketplace.
Conclusion
Trusted data is the bedrock of AI success. Without it, even the most advanced algorithms can lead to flawed insights and costly decisions. Kamal Maheshwari’s insights from Episode 4 of Data Faces Podcast highlight the urgent need for organizations to prioritize data trust.
For business leaders, the path forward is clear:
- Assess your current data environment and identify gaps.
- Invest in modern, unified data platforms to streamline governance and quality.
- Build a culture of accountability and transparency around data usage.
By taking these steps, companies can unlock AI’s full potential, mitigate risks, and make confident, data-driven decisions.
Listen now on YouTube: https://youtu.be/U9pq1D2iVRY
Transcript Summary
0:00 – Introduction
David Sweenor introduces Kamal Maheshwari, Co-Founder of Decube, and sets the stage for a discussion on the importance of trusted data as the foundation for AI success.
0:44 – Kamal’s Background and Vision
Kamal shares his journey in the data industry, from working at Oracle and Cloudera to co-founding Decube. He emphasizes his passion for solving the data trust crisis and highlights the role of trusted data in AI-driven decision-making.
2:13 – Why Data Trust Matters
Kamal explains that data trust ensures confidence in data at every stage of its lifecycle. He compares trusted data to a building’s foundation, arguing that poor data quality can compromise the integrity of AI systems, leading to catastrophic business decisions.
5:37 – The Impact of Poor Data Quality
The discussion shifts to real-world scenarios where flawed data leads to significant delays and decision-making errors. Kamal introduces the concept of a “data trust score” to proactively monitor and address data issues.
9:26 – Challenges in Building Trusted Data Systems
Kamal identifies three key challenges: legacy systems, siloed teams, and data quality failures. He highlights how these obstacles prevent organizations from achieving reliable AI outcomes.
14:39 – The Role of Leadership
Leadership plays a pivotal role in fostering a culture of data integrity. Kamal stresses the importance of aligning teams and breaking down silos to ensure data strategies are cohesive and effective.
21:08 – Unified Data Platforms as a Solution
Kamal discusses how unified data platforms, like Decube, can help businesses overcome data trust challenges by integrating governance, quality, and cataloging into a single system.
27:37 – Quantifying Data Trust with Metrics
The importance of quantifying data trust through measurable metrics, such as trust scores, is explored. Kamal explains how businesses can use these scores to track progress, detect risks, and improve decision-making.
36:34 – Future Trends in Data Trust
Kamal identifies emerging trends, such as real-time data validation and AI-driven governance, which will shape the future of data trust in an AI-driven economy.
37:33 – Final Advice for Business Leaders
Kamal offers practical advice: assess your current data environment, adopt modern tools, and embrace innovative solutions to build trust in your data and drive AI success.