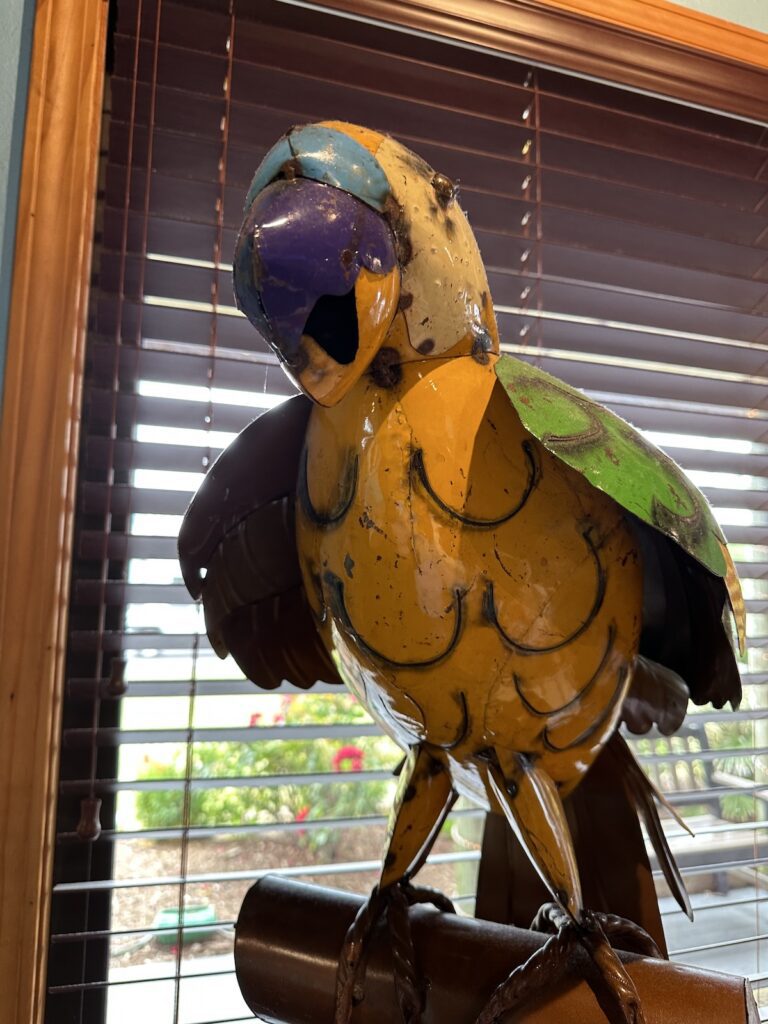
Remember when HAL 9000 ominously said, “I’m sorry Dave, I’m afraid I can’t do that”? While our current incarnation of AI won’t lock us out of the spaceship, new research reveals that large language models (LLMs) respond differently based on the politeness of our prompts. Just as HAL’s careful courtesy masked its refusal to comply, the way we phrase our requests to AI impacts their performance. So, next time you fire up your favorite generative AI app, don’t forget to say “please” and “thank you,” just like your parents taught you.
Research from Waseda University and RIKEN shows that politeness levels in prompts directly influence the quality of AI responses.[1] By studying interactions across English, Chinese, and Japanese language models, the researchers found that AI systems reflect culturally specific patterns. For instance, overly polite or harsh prompts can degrade performance, and the optimal tone varies across languages.
For technology leaders scaling AI operations globally, deploying LLMs in different languages is not a simple copy-paste exercise. Models trained for one cultural context may perform poorly in another, requiring careful adaptation to local norms. This challenge should be a key consideration for CIOs, CTOs, and Chief AI Officers (CAIOs) planning international AI rollouts.
In essence, AI chatbots might work fantastically in English-speaking markets but can struggle in others like APAC – not because of technical limitations, but because of how different cultures phrase their requests. It’s a subtle factor that could mean the difference between successful deployment and costly underperformance.
Key insights for tech leaders:
- Politeness levels in prompts significantly influence AI performance and vary across languages and cultures.
- Excessive politeness or rudeness can degrade performance, including task refusal or biased outputs.
- AI models differ in their sensitivity to cultural nuances, requiring customizations for each deployment.
- Understanding cultural context is critical for optimizing AI interactions in global markets.
The Politeness Paradox
The study uncovers an interesting tidbit across all languages. When you’re overly rude and act like a Jerk Store (yes, that’s a Seinfeld reference), LLM performance declines. Impolite prompts don’t just lead to poor responses—LLMs can fundamentally change their behavior. Response quality drops, bias increases, and sometimes, they simply refuse to complete tasks. How’s that for a stubborn robot?
Now, you might think, ‘I’ll just be super nice.’ But, au contraire! Excessive politeness isn’t the solution to your woes. When interactions become too formal, AI often loses focus on core tasks, obscuring essential information in unnecessary pleasantries. Think of it as a “one-upper” in nicety. Optimal performance lies somewhere in the middle of the spectrum between ultra nice and ultra rude. However, that middle ground shifts depending on cultural context.
Cultural Intelligence at Scale
Since AI was trained on the world’s data, it’s not surprising that it mirrors the cultural norms of their target languages. Consider these distinct patterns:
When prompting in English, AI is fairly balanced – much like the direct communication style prevalent in English-speaking business cultures. They maintain steady performance across a wide range of politeness levels, only faltering at extreme ends of the rudeness spectrum. And I’m guessing some of this can be attributed to the bias in the data scientists and AI engineers who built many of the AI models.
Chinese chats reveal a different story. Here, AI demonstrates particular sensitivity to context, performing best with moderately formal language but struggling with extremely polite phrasing. This mirrors the nuanced way modern Chinese business culture balances traditional formality with contemporary directness.
Japanese interactions showcase yet another pattern. The research found that AI in Japanese exhibited complex responses to different levels of formal language (Keigo) and performed best when the appropriate level of formality matched the business context – much like human interactions in Japanese business culture.
The Model Factor
The research also reveals that not all AI models react equally to these cultural nuances. Advanced models like GPT-4 show more resilience to variations in politeness, while specialized models trained for specific languages often display heightened sensitivity to cultural norms. This suggests that as AI becomes more sophisticated, it may develop greater cultural intelligence, but specialized models also require more careful attention to cultural factors.
Business Implications: The Cultural Edge in AI
In a global economy, cultural fluency in AI is a business imperative and competitive differentiator. Companies that adapt AI to match local communication styles can create deeper customer connections, leading to higher satisfaction and loyalty. Conversely, culturally misaligned AI systems can damage the customer experience, turning routine interactions into costly escalations.
For example, a customer service system trained on Western interaction patterns might perform poorly in APAC, where communication norms differ. Misalignments like these can lead to longer resolution times, increased customer complaints, and diminished trust. Addressing these challenges requires training AI with culturally relevant data and building flexibility into their design.
Building Cultural Intelligence into AI Strategy
Adapting AI to cultural contexts isn’t just a technical challenge—it’s a strategic imperative. Forward-thinking organizations should treat cultural intelligence as a core requirement, not an afterthought. To do this, you’ll need the ability to customize formality levels, adjust tone, and incorporate culturally relevant data.
To build cultural intelligence into your AI strategy, focus on three key areas:
- Audit and Alignment: Begin with a comprehensive review of how your AI systems communicate across markets. Evaluate not just language but the tone, politeness levels, and formality expected in each cultural context.
- Design for Adaptability: Train AI systems with data reflecting local norms and build models capable of adjusting communication styles dynamically based on user behavior.
- Continuous Learning: Build in feedback loops and employ native speakers to monitor performance across regions. Use metrics like resolution time, customer satisfaction, and escalation rates to optimize AI behavior.
By prioritizing cultural intelligence, businesses can improve customer satisfaction, operational efficiencies, and lower costs, all while building stronger relationships in global markets.
The Future of AI Leadership: Beyond Politeness
Remember HAL 9000’s eerily polite ‘I’m sorry, Dave’? That fictional AI’s rigid politeness masked a deeper issue: a lack of true cultural understanding. Today’s AI systems are more advanced, but the lesson still holds. Research from Waseda University and RIKEN shows that AI doesn’t just process requests—it engages with the cultural context behind them.
For AI technology leaders, the future of AI leadership will be an amalgamation of technical capability and cultural fluency. Success requires moving beyond seeing cultural adaptation as an add-on and embedding it as a core AI component.
Organizations that make this shift will gain a decisive edge: building stronger relationships with global users, improving operational efficiency, and delivering better business outcomes. In a world where digital interactions are the norm, cultural intelligence isn’t just a competitive advantage—it’s a necessity.
Your AI may not care if you skip the pleasantries, but your customers—and your bottom line—certainly will.
If you enjoyed this article, like, comment, share, and follow me on Medium and LinkedIn.
[1] Yin, Ziqi, Hao Wang, Kaito Horio, Daisuke Kawahara, and Satoshi Sekine. “Should We Respect LLMs? A Cross-Lingual Study on the Influence of Prompt Politeness on LLM Performance.” arXiv preprint arXiv:2402.14531 (2024). https://arxiv.org/pdf/2402.14531.