Why Questioning is the Key to Success
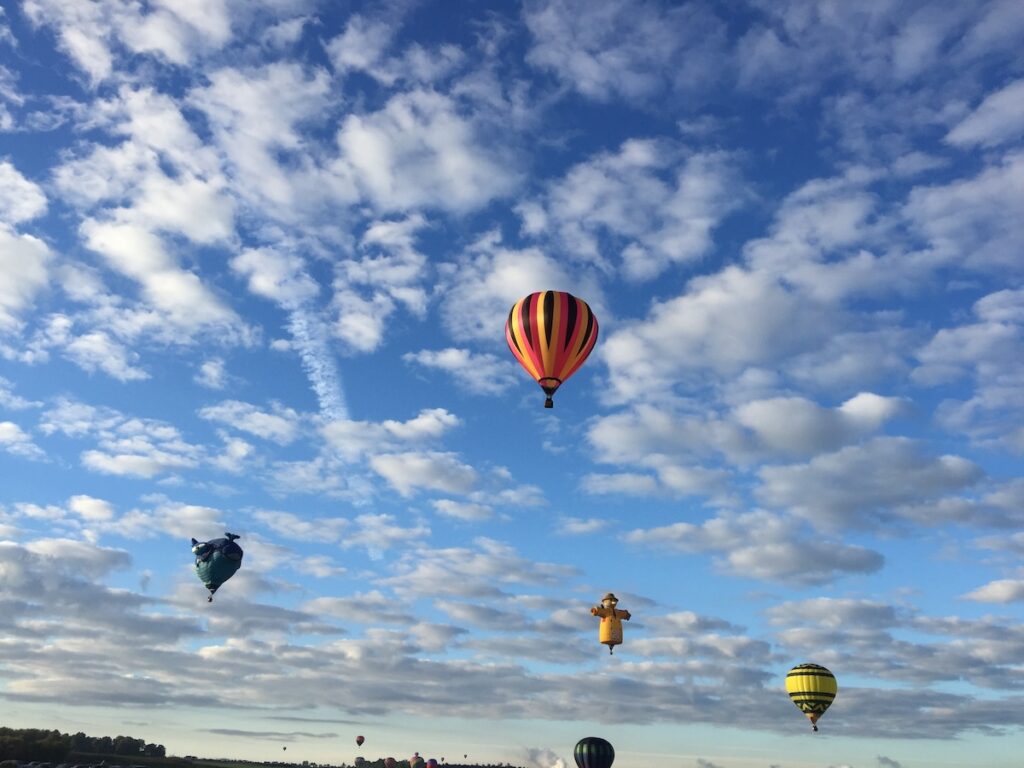
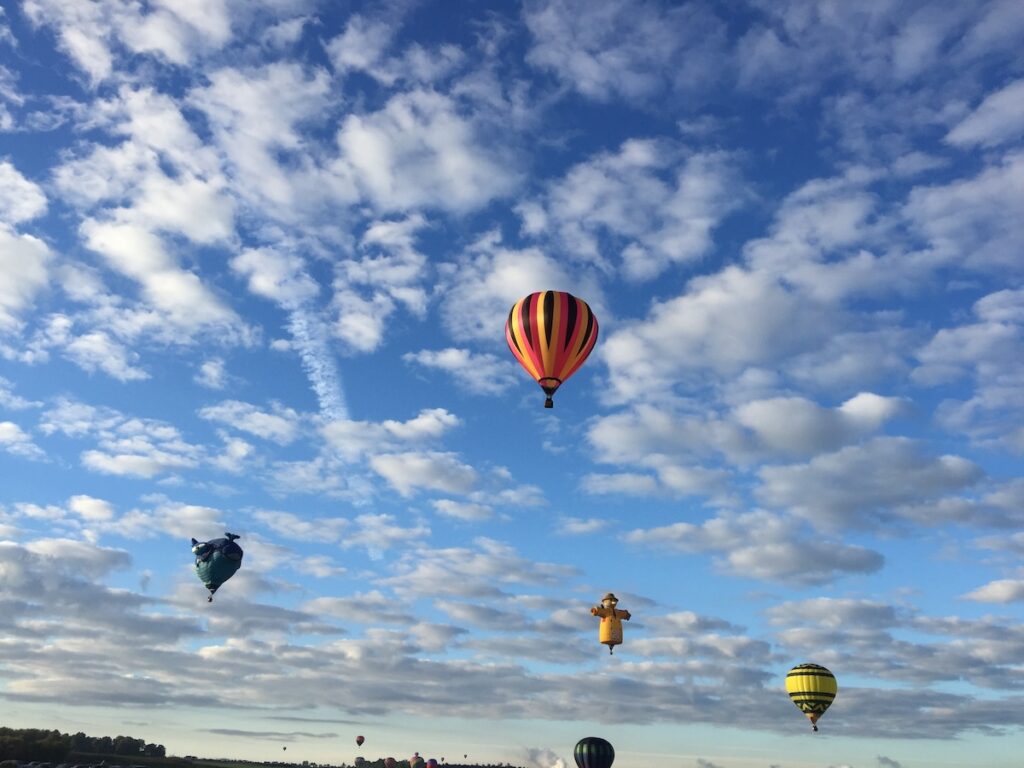
Introduction
We’ve all seen the stats. McKinsey’s 2023 article proclaimed that productivity enhancements yielded by generative AI could add up to $2.6 to $4.4 trillion annually to the global economy.[1] As I’ve pointed out in my article, Will Generative AI Take My Job? Maybe., many organizations are slashing workforces thinking that AI can replace people–2024 promises to be a bumpy ride for sure.[2] However, given the promise of a generative AI, many businesses struggle to achieve actual results. The culprit? A lack of critical thinking often stifles innovation, leading to mediocre implementations that fail to deliver meaningful productivity improvements.
For companies testing generative AI, the line between achieving immense process efficiencies and lackluster performance is often determined by the quality of human engagement with the technology. As businesses rush to adopt generative AI tools, the risk of settling into mediocrity looms large. This article explores three common pitfalls that lead to mediocre generative AI implementations and provides actionable insights to overcome these challenges.
The tell-tale signs of generative AI mediocrity are often hidden in plain sight, obscured by AI jargon that promises much but delivers little. We’ve seen the rise of AI junk words like “ever-evolving landscape,” “harness,” “delves,” “crucial,” “vital,” “plethora,” “firstly,” “moreover,” “embrace,” “journey,” “blueprint,” “transformational” and many others muddy up content.[3]
Too many organizations are treating generative AI as an oracle to be mindlessly obeyed and are falling victim to three key pitfalls that stifle creativity and innovation:
- Overreliance on the technology
- Taking outputs at face value
- Not asking the right questions
By avoiding overreliance on technology, critically evaluating outputs, and asking the right questions, businesses can use generative AI to achieve competitive advantages by creating quality content and not regurgitating the obvious.
The Pitfalls of Blindly Adopting Generative AI
Overreliance on the Technology
Let’s start with the first pitfall: overreliance. There’s a common misconception that generative AI is an all-knowing, all-powerful solution. Simply provide a prompt, and out comes marketing copy, code, or analysis that is fit for deployment. Many are taking the Wizard of Oz’s “pay no attention to the man behind the curtain” advice too literally.
This is dangerously misguided. Generative AI is a tool, not a magic wand. It’s trained on the data we feed it, inheriting all the biases, blindspots, and limitations therein. Blindly trusting its outputs without human scrutiny is a recipe for irrelevant or nonsensical results. When dealing with generative AI output, we need to be careful not to just take the outputs at face value – the accuracy of results must be monitored and verified.
Businesses also risk automation for automation’s sake, deploying generative AI haphazardly rather than focusing on high-value use cases. As Covey would say, “Begin with the end in mind.” Human expertise remains indispensable for applying the technology toward productive ends.
The solution? Develop clear use cases and success metrics to ensure generative AI enhances, rather than replaces, human capabilities. Maintain meaningful oversight and control.
Taking Things at Face Value, Accepting the First Response
Now, the second pitfall: accepting the first response at face value. Too often, businesses treat generative AI’s initial output as the final, immutable answer. This is folly. Generative AI provides a starting point, not a polished conclusion. Its outputs can be biased, inaccurate, or lacking in nuance. Critical evaluation and iteration are essential.
Creating content with generative AI is an interactive and iterative process. You should never be satisfied with the first answer. You need to iterate, refine, and optimize the output. Many people may risk falling prey to the “Einstellung effect,” which is the human tendency to revert to familiar solutions rather than explore new paths. Generative AI can suggest novel approaches, but only if we’re open to challenging assumptions.
Business leaders should encourage teams to iterate, explore alternatives, and dig deeper. The first response may be adequate, but is it novel? Invest in developing critical thinking skills to push generative AI towards more insightful and creative outputs. Teams need to have back-and-forth dialogue and not simply accept the initial suggestions.
Not Asking the Right Questions
This leads us to the third, and perhaps most insidious, pitfall: not asking the right questions.
Generative AI is remarkably capable, but it’s not a mind-reader. It can only synthesize outputs based on the prompts and data we provide. Vague, broad, or poorly framed queries yield vague, broad, or flawed responses.
As the saying goes: garbage in, garbage out. When interacting with generative AI, you need to be extremely specific about your use case, how you ask the question, and desired output. Many first-time users think they can say, “Write me a blog about <insert a topic>” and get something interesting and coherent. In fact, quite the opposite is true. If you have little knowledge about the topic you’re asking it to create content for, the output is quite convincing–you don’t know any better. But if you ask it something that you have in-depth knowledge of, the lackluster depth, inaccuracies, and poor writing will be staring you in the face.
The solution starts with clearly defining objectives and framing problems with rich context. But it doesn’t end there. We must remain aware of the data used to train these models–it reflects society and all of its foibles. Be aware of biases, stereotypes, and confabulations that could undermine outputs. And we can’t be afraid to challenge AI-generated solutions, pushing the technology to explore more creative paths.
What’s the solve? Foster a culture of curiosity. Develop prompting skills. Provide teams with substantive problem training. Organizations need to approach generative AI not as an omniscient wizard, but as an immensely capable – yet imperfect – collaborator to be queried, tested, and refined through rigorous dialogue.
Develop a Questioning Mindset
At its core, avoiding generative AI mediocrity requires a fundamental shift in mindset. We must move beyond blind deference and embrace a spirit of inquiry. Treat generative AI not as an easy shortcut, but as an opportunity to enhance human ingenuity. Question assumptions. Probe outputs. Iterate relentlessly in pursuit of exceptional solutions.
As the Harvard Business Review recently highlighted, teams that engage generative AI through this questioning lens achieve far more creative and impactful outcomes.[4] The article Don’t Let Gen AI Limit Your Team’s Creativity noted that AI-assisted teams generated only 8% more ideas on average, got fewer bad ideas, but had fewer top ideas than non-AI teams. Their confidence in their solutions was much higher than warranted by the actual quality of ideas. To maximize the benefits of generative AI for ideation and problem-solving, the article recommends:
- Being precise when framing the problem to solve provides as much context as possible.
- Having individuals brainstorm ideas first before incorporating AI input to avoid groupthink.
- Extensively “training” the AI by inputting relevant data to provide sufficient context.
- Treating the AI as a back-and-forth conversation partner, not just taking its first suggestions.
- Having an outside facilitator consolidate ideas to steer the process, set priorities, and identify the next steps.
The key is approaching generative AI as an ongoing structured conversation, not just an oracle that will immediately provide the best solution when prompted. This avoids the trap of mediocrity.
Many organizations are using generative AI quite effectively. But realizing its full potential demands human mastery—a willingness to thoughtfully steer these powerful models towards productive ends.
Overreliance, blind acceptance, and poor questioning inevitably lead to mediocre results. But those who embrace a culture of curiosity, who treat generative AI as a collaborative partner to be queried and refined, will unlock an era of unprecedented innovation.
The choice is yours: mediocrity or brilliance? Questioning is the path to success.
If you enjoyed this article, please like it, highlight interesting sections, and share comments. Consider following me on Medium and LinkedIn.
Please consider purchasing my latest TinyTechGuide: Generative AI Business Applications: An Exec Guide with Life Examples and Case Studies.
If you’re interested in this topic, check out TinyTechGuides’ latest books, including The CIO’s Guide to Adopting Generative AI: Five Keys to Success, Mastering the Modern Data Stack, or Artificial Intelligence: An Executive Guide to Make AI Work for Your Business.
[1] Mckinsey & Company. 2023. “Economic Potential of Generative AI | McKinsey.” Www.mckinsey.com. June 14, 2023. https://www.mckinsey.com/capabilities/mckinsey-digital/our-insights/the-economic-potential-of-generative-ai-the-next-productivity-frontier#introduction.
[2] Cooban, Anna. 2024. “AI Will Shrink Workforces within Five Years, Say Company Execs | CNN Business.” CNN. April 5, 2024. https://www.cnn.com/2024/04/05/business/ai-job-losses/index.html.
[3] HelpingHand_. 2023. “Overused ChatGPT Terms – Add to My List!” Reddit. August 28, 2023. https://www.reddit.com/r/ChatGPTPro/comments/163ndbh/overused_chatgpt_terms_add_to_my_list/?rdt=56855.
[4] “Don’t Let Gen AI Limit Your Team’s Creativity.” 2024. Harvard Business Review. March 1, 2024. https://hbr.org/2024/03/dont-let-gen-ai-limit-your-teams-creativity.