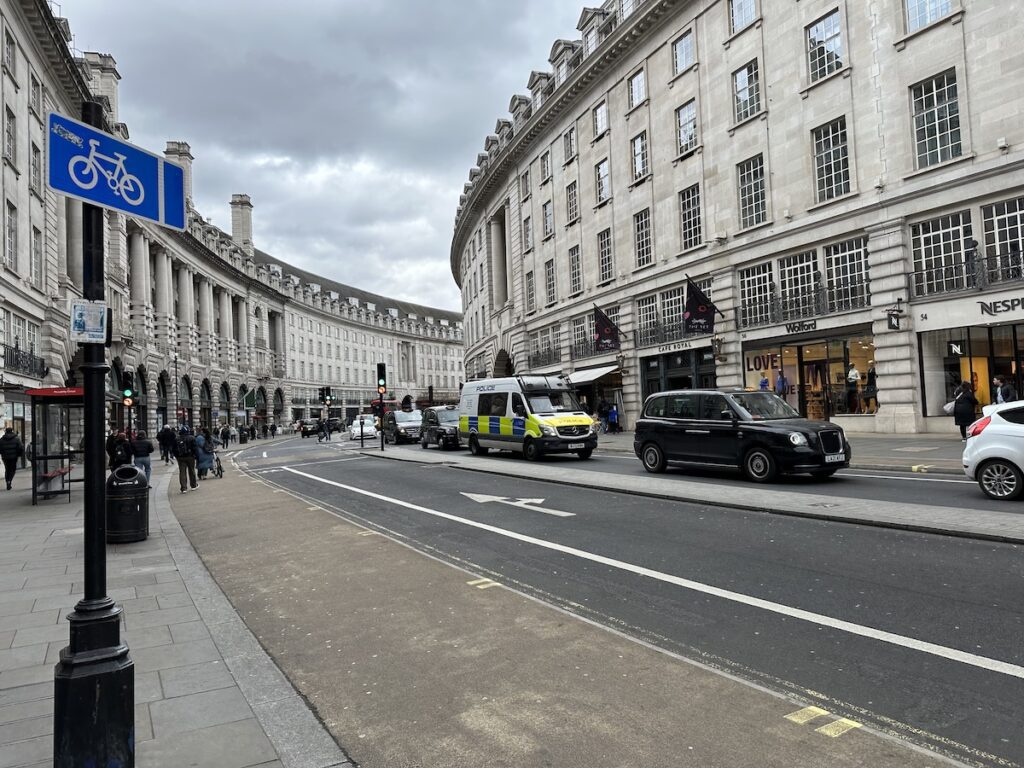
Have you started using artificial intelligence (AI) and machine learning (ML) in your analytics initiatives? What kind of results are you seeing?
Generative AI is all the rage – since November 2022, the popularity of OpenAI’s ChatGPT (a chatbot on steroids) and DALL·E 2 (art and images) has certainly generated a lot of buzz. Naturally, that buzz is echoing in the halls of data science and analytics, with people asking how they can apply AI to make better business decisions with less effort. Decision makers and business leaders want the latest technology at work for them, and AI is no exception.
Having spent my career in data and analytics, there are a number of AI myths that are often repeated. My colleagues Mellisa Burroughs, director of technology solutions marketing, and Monica Cisneros, manager of product marketing and I decided to bust the most common of these myths in a webcast called Myth Busters: Data Science + Analytics Edition on The Ravit Show. This post summarizes some of the mythbusting from that webcast and sets you on the path to getting started with AI in analytics.
Myth #1: AI is like The Terminator or Westworld.
Many people associate AI with renegade computers, robots and android characters they’ve seen in entertainment. That’s understandable given movies like 2001: A Space Odyssey, The Terminator, and Westworld. But even with high-concept, high-functioning prototypes like those, the reality today is that robots are accomplishing tasks like making pizzas and shuttling parts around warehouses. Some of them are on wheels but few of them can negotiate stairs smoothly. Autonomy is still some way off, since most are connected by cables.
Instead of envisioning AI in the context of human intelligence, think about a pragmatic definition of artificial intelligence: Data + Analytic Insights + Automation = AI.
- Data: All the points of information you collect from sources like equipment, machines, satellites, users, points of sale and the internet.
- Analytic Insights: These are what you apply to specific business problems to take action. For example, discovering a likely 20% increase in COVID-19 hospitalizations next month is a fine insight. But you have to act on it to order more medical supplies, increase staffing levels or add more beds.
- Automation: This allows you to take smart actions repeatedly, without repeated human intervention. Think business rules, decision management, chatbots, virtual agents and robotics.
Myth #2: AI is going to take over the world.
This myth is popular, especially with the competition between Google’s Bard and Microsoft’s Bing. With AI-search chatbots on the march, most human endeavors could soon be taken over.
But if you consider the decades and tens of billions of dollars that General Motors, Ford and Tesla have spent, self-driving cars are not yet ready for the mass market. In the U.S., they tend to be confined to the Sun Belt, because they still can’t handle weather patterns trickier than partly cloudy. State-of-the-art robot cars also struggle with construction, animals, traffic cones and crossing guards.
Myth #3: AI is going to take our jobs. You won’t have to think; the computer will do it.
Successive waves of technology innovation have mostly disproved this.
The industrial revolution brought about mechanization through water and steam, and labor markets adapted to it. Motor vehicles displaced the horse and buggy, without the predicted downfall of civilization. The digital revolution has brought miniaturization and huge multiplier effects — up to and including AI — without calamitous job loss. AI can make our lives better and it has the power to help humanity.
There’s no denying that AI will displace workers in sectors such as health care, customer service and logistics. After all, the day that Level-5, fully autonomous cars are safe enough for mass adoption, the transportation industry will surely rely less on human drivers. But many experts believe that AI and robotics will complement and augment most jobs, creating even more jobs in new markets.
Keep in mind that ChatGPT and DALL·E 2 are very impressive, but they can only return what they are trained to return. Their accuracy is questionable and their lack of understanding about what is factual holds potential for misinformation. We see NLP technologies integrated in the context of augmenting the creation of analytic workflows, dashboards and predictive models. We think of it as opening up new conversational interfaces beyond data and analytics, making interaction with machines less formulaic.
Myth #4: AI is for experts only.
While it’s true that it takes experts to create AI models, it’s not true that they alone are qualified to take advantage of them. Even without budget and staffing for experts, small organizations are successfully applying machine learning in areas like these:
- Analyzing PDFs: This saves time by opening and scanning the content of PDFs, then summarizing the text or distilling the main points.
- Forecasting revenue — Every business tries to estimate future income; customers use time-series forecasting to analyze specific time segments and estimate increases and decreases in revenue.
- Classifying: Customers invariably gravitate to different profiles, and AI can help segment them into groups. Do high-value customers buy a little from you every week or a lot once a year? Knowing that can help you tighten your relationship with them.
- Customizing offers: After classifying your customers, it becomes easy to tailor marketing campaigns and discounts to each profile and spending pattern.
Those and similar opportunities are well within the realm of AI possibility for small-to-medium businesses (SMBs).
Myth #5: AI is only for enterprises.
Every day we see the effects of predictive analytics in products from tech behemoths like Netflix, Amazon and Walmart. Small wonder, then, that many people associate AI with the enterprise.
The fact, though, is that SMBs without large budgets or well-staffed teams are wielding low-code/no-code and open-source tools fruitfully, even with just a single license of those tools. They’re discovering the same advantages in data visualization or machine learning tools that enterprises enjoy, commensurate with the size of their business.
The technology has advanced enough to become accessible and useful to people without deep data science or coding expertise. For that matter, it’s not hard to find YouTube or TikTok content showing how people are analyzing fashion or television trends using these tools.
Further refuting this myth is the strong trend toward making analytics accessible to everyone — enabling a larger share of the workforce to access and benefit from analytics either independently or with help from internal experts. It ensures that data drives more of the decisions in the business. It can also help free up your highly skilled analytics professionals like data scientists to focus on the company’s big challenges, not on recurring, business-as-usual questions.
Mind you, myths circulate about democratization too.
Myth #6: Democratizing analytics is not good strategy.
This spans a few sub-myths:
Just give everybody a dashboard.
Dashboards do not equal democratization, because data is not helpful unless it has been collected and analyzed to answer a particular question. Visualization tools are useful, but if their users don’t understand the data behind them — how it was transformed, the phenomena being measured — they risk drawing incorrect conclusions.
That’s why data literacy is important. You can’t just distribute new tools to people; you have to ensure that they’re properly trained and that they can interpret results accurately.
Democratizing analytics ruins governance.
Some IT leaders fret that, giving more people access to sensitive data is an invitation to analytics chaos. But part of allowing more people to participate in the analytics process is to ensure that your analytics processes remain compliant with security protocols for the data being accessed. That’s data governance, and democratization and governance are allies rather than adversaries.
IT should be involved in democratization, but your business users should be empowered to help. After all, you can’t expect IT to do everything for you when it comes to data.
Democratizing analytics is a feel-good activity with no real impact on the business.
Putting analytics into the hands of business teams on the front lines of your organization will help them do their job much more effectively. It tightens feedback cycles and makes the organization more agile because people who spot opportunities can take action on their own, without waiting for support from a data scientist.
The International Institute for Analytics says that doing analytics across multiple business units increases your organization’s analytics maturity. They’ve correlated analytics maturity to dozens of important metrics, like three-year revenue and 10-year operating income. They’ve demonstrated that businesses with high analytics maturity can earn up to four times the revenue of competitors lacking that maturity.
Myth #7: AI is unapproachable and there is no creativity.
Some people find AI unappealing because they think it can’t create anything. Or they think that data science is staffed by statistical zealots, gearheads and python jocks.
True data scientists know it’s not that simple. Being creative in data science is a matter of understanding the overall goal of the model you’re trying to build and of the variables you’re using. Just picking out and generating the right variables to train the model on is an art. Choosing the right test, the right algorithm and the right method to solve the problem at hand is a creative endeavor.
As a creative exercise, data science is about framing the problem. Are you asking the right question to achieve that goal? Visualizations are delightful, and human interaction with results is important. But framing the problem and selecting the analytic method are demanding tasks that require expertise and creativity.
Myth #8: I don’t need AI.
Maybe you don’t. But any simplicity in your life depends on things that AI powers; for example, predictive shipping, purchase recommendations, news, internet uptime, fraud detection, identity protection and spam filtering.
In short, we’re already immersed in AI, and the genie is not going back into the lamp. If that doesn’t set your mind at ease, then maybe you have an exaggerated concept of what AI entails. Refer to the pragmatic definition of AI — Data + Analytic Insights + Automation — described in Myth #1 above.
How to get started with AI
To return to the original question, if you haven’t yet started using artificial intelligence (AI) and machine learning in your analytics initiatives, here is a framework for getting started.
- People — In upskilling data literacy, your goal is not to turn all of your employees into data scientists, but rather to cultivate a class of citizen data scientists. These are front-line workers who understand the power of data to improve the outcome of their work.
- Process — Start by tying AI and analytics to business outcomes and issues. Think big, start small and act fast.
- Technology — At the same time that you’re developing people and process, you’ll find you need technology. In most cases, it consists of software products that help you ensure quality in the data you’re analyzing. It includes self-service tools that empower front-line workers, and analytics cloud and automation to accelerate processing.
Before you begin implementing #ML models, you should think about the following::
- Understand what ML can and can’t do.
- Translate business problems into the language of ML predictions.
- Choose and act on predictions that can capture business value.
- Identify the data ML models require (and don’t require).
- Communicate the success of ML pilots in business terms.
If you are interested in starting your AI journey, I have developed a handy template with seven to help you get started.
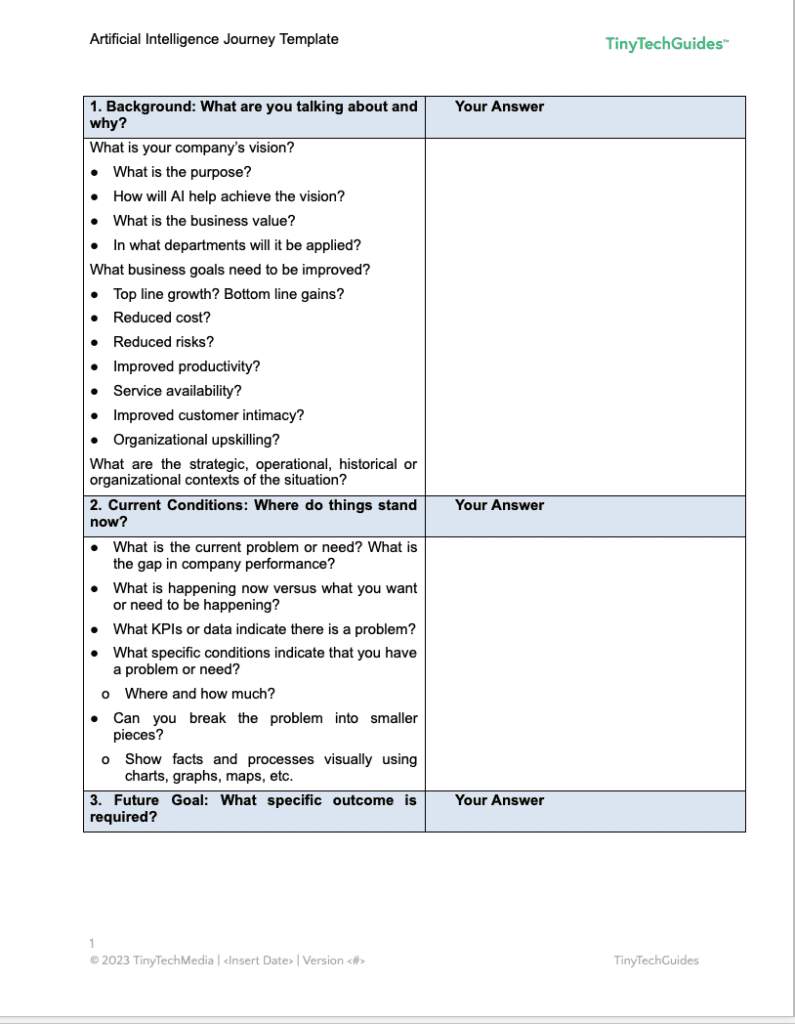
Next steps
Watch the podcast from which this blog post was adapted: Myth Buster: Data Science + Analytics Edition.Grab a copy of Artificial Intelligence: An Executive Guide to Make AI Work for Your Business.
Categories
- AI Trends (8)
- Analytics Leadership (8)
- Artificial Intelligence (52)
- Data Faces Podcast (4)
- Data Leadership (2)
- Marketing (16)
- Modern Data Stack (3)
- News (8)
- Tech News (1)
Latest Posts
- The Grandmother Test: Building AI Trust Beyond Technology
- Don’t Trust Your AI With Dates: The Calendar Blindspot
- The Gen AI Shift: How Product Marketing Managers Are Adapting
- Your LLM’s So Smart It’s Missing the Pepperoni
- There Is No Post-AI World: Preparing Your Organization for the Agent Revolution