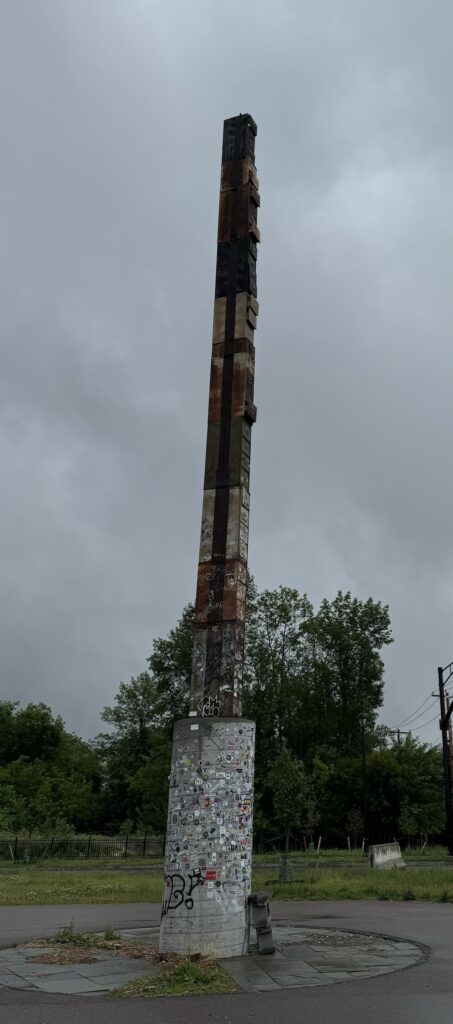
As the world turns, data is created at a pace that is incomprehensible to most. In fact, in the 2030s, it’s estimated that we will be generating a Yottabyte (1024 bytes) of data per year. To put that into perspective, if you were to put that data on a stack of DVDs, it would reach all the way to Mars—which, on average, is 140 million miles away from Earth![1] For the past few decades, organizations have done a decent job capturing and storing important structured data for their business. Data-rich businesses within financial services, high-tech manufacturing, consumer packaged goods (CPG), retail, and others have learned to find hidden patterns of insight within their data. Rather than relying on gut feeling and intuition, they have used those insights to help make faster and better business decisions. However, other sectors like healthcare providers, legal, insurance, construction, and education, which are also awash in data, have lagged in adopting data, analytics, and artificial intelligence (AI). Why do some industries lag in adopting data, analytics, and AI while others thrive?
While some argue that security, privacy, and regulatory concerns are the primary cause, there is probably a more fundamental reason for this difference. For the most part, industries leading in adopting analytics and AI rely on data for their day-to-day operations and business decisions. The faster these organizations can process and analyze data, the faster they can make decisions and, thus, generate greater profits. So, it comes down to the fundamental economics of the situation. Organizations leading in adopting AI have lower costs, higher bottom-line returns, greater efficiency, and reduced risks. Essentially, data is the fuel on which their business runs. Now, security, privacy, and regulations are undoubtedly important, but that should not be an excuse for any company’s inaction. As exemplified below, Analytics and AI can be used in non-regulated and highly regulated environments.
For example, consider UBS, the giant Swiss banking behemoth with revenue totaling USD 96.798 billion (for 2023). Swiss banks are known for their privacy and security. They must adhere to some of the strictest banking regulations on the planet. In 2016, UBS embarked on an AI and analytic journey. They partnered with an analytics software provider to deliver self-service data prep and analytics at scale. Fast forward to 2021; UBS has over 3000 analytics users worldwide on every continent. UBS runs over 6,000 analytic workflows each day and uses analytics for fraud detection, capital planning, and revenue forecasting.[2] This is a prime example of being able to balance stringent regulations and self-service analytics![3]
In another example, the fast food giant McDonald’s has spent hundreds of millions of dollars transforming its business operations with artificial intelligence. They use digital order boards that can dynamically promote different food items depending on the time of day and weather conditions. For example, if the temperature is hot outside, they may recommend more cool drinks like shakes and iced coffees. At drive-thrus, some stores have license plate readers that can recommend orders based on prior order history. The net is that they are using analytics and AI to increase drive-through throughput and increase ticket sizes on orders by upselling additional items.[4]
As you can see from the above examples, data, analytics, and AI are used to improve efficiency, increase revenues, lower costs, and reduce risks. The companies that succeed at applying data, analytics, and AI to improve decisions are the ones who win in the marketplace. However, there’s a wrinkle. Organizations keenly understand the importance of data, analytics, and AI, but relatively few have been able to transform their organizations into AI-driven organizations. A survey by NewVantage Partners suggests that companies are making progress in creating a data-driven culture, which improved from 23.9% to 48.15% from 2023 to 2024.[5] That means more than half still haven’t unblocked AI’s true potential. What’s holding them back? What is preventing companies from crossing the AI chasm and achieving analytics nirvana?
Ninety-two percent of executives claimed that cultural change was the biggest impediment to establishing a data-driven culture.[6]
Culture—not data, tools, technology, and skills—is the number one impediment preventing organizations from reaching their AI potential.
Data vs. Insight vs. AI Driven
In the parlance of our times, the term data-driven has become a popular buzzword. Many business leaders and analytics practitioners I interact with have different definitions of being data-driven. Is a data-driven organization different from an insight-driven organization? How are those different from AI-driven organizations?
Before answering those questions, let’s start with a few definitions.
We will define a few essential terms according to the Merriam-Webster dictionary:[7]
- Automation: automatically controlled operation of an apparatus, process, or system by mechanical or electronic devices that take the place of human labor
- Data: factual information (such as measurements or statistics) used as a basis for reasoning, discussion, or calculation.
- Decision: the act or process of deciding
- Decide: to select as a course of action
- Digital: composed of data in the form of especially binary digits
- Insight: the power or act of seeing into a situation
Additionally, the Gartner IT Glossary provides the following definitions as a helpful starting point:[8]
- Artificial intelligence (AI): applies advanced analysis and logic-based techniques, including machine learning, to interpret events, support and automate decisions, and take actions.
- Digitization: the process of changing from analog to digital form, also known as digital enablement. Said another way, digitization takes an analog process and changes it to a digital form without any different-in-kind changes to the process itself.
- Digitalization: the use of digital technologies to change a business model and provide new revenue and value-producing opportunities; it is the process of moving to a digital business.
Now that we have a clear understanding and precise definitions of these key terms, let’s discuss the differences between data-driven, insight-driven, and decision-driven.
What Does it Mean to Be Data Driven?
First, let’s start with data. What is data? Besides being a famous Star Trek character named Data, data is a fact. For our purposes, let’s think of it as a measurement. All data begins as an event–a keyboard stroke or mouse click, a credit card swipe, a social media check-in, the movement of your smartphone, a pressure measurement in a factory, a medical image, a cell phone picture, or a simple drive down the street. Fundamentally, in the modern era, for just about every interaction with the external environment, if there is a paper & pencil to record something or an electronic device nearby, the interaction generates data. If the data is not captured digitally, it will need to be digitized (translated to something understandable by a computer) before your organization can use it effectively. Typically, this data is ingested, harvested, and stored for safekeeping–whether in memory on a device, hidden within a log file, data lake, data swamp, spreadsheet, or some other persistent storage mechanism.
Now, before we can be data-driven, we must digitalize our business. If you refer to the previous definitions, digitization is about taking measurements or facts and putting them into a form that a computer can understand. But simply having data in a digital format doesn’t really change your business–it certainly can have dramatic impacts, but it doesn’t have to. For example, many organizations have digitized contracts and expense receipts, which means they can access these contracts in a PDF file storage system somewhere. But, it doesn’t mean they can easily find or analyze the data within the contract or receipt! Digitalizing your business means you will need to change your manual process to a digital one. This means you will use some business rules and automation to make the process more efficient. In other words, some parts of the process can happen without human intervention. You can automate inefficient processes just as well as efficient ones. Most companies that digitalize their processes re-evaluate their manual, inefficient processes, but it’s not a requirement.
Synonymous with digitalization, data-driven simply means using data and automation to operate business processes within your business digitally, without human intervention.
For example, consider placing an order with your favorite online store or app. This business process is generally called Order-to-Cash (O2C). The steps of this business process typically follow something like this, as illustrated in Figure 1.1:[9]
Figure 1.1: Typical Order to Cash Process
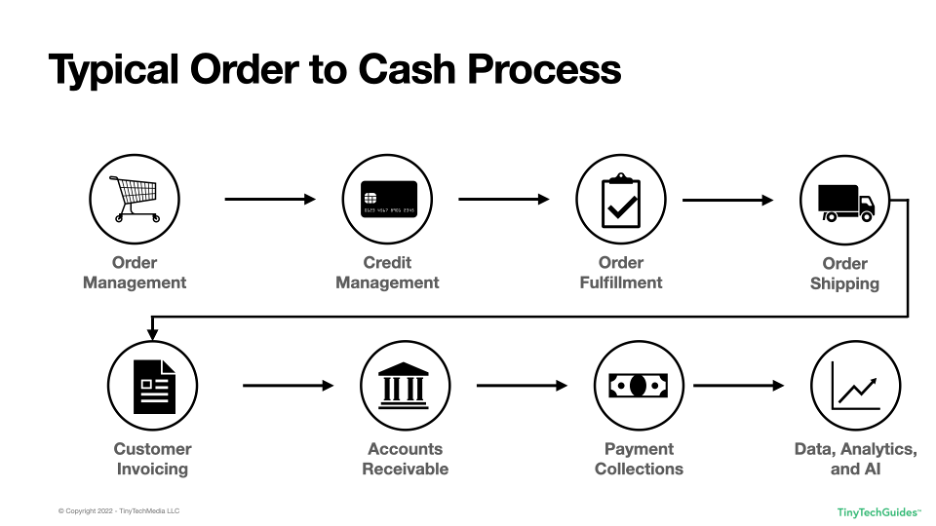
Each step generates data, and it can occur digitally or manually. Most organizations have digitalized and automated these processes, but some still occur manually.
What Does it Mean to Be Insight Driven?
Now that we understand that data-driven is simply applying digitized data and automation to run some parts of your business processes automatically, let us discuss what it means to be insight-driven. If we recall our definition of insight earlier, it is “the power or act of seeing into a situation.”
Data doesn’t do anything on its own. It’s inert. Analytics and AI are the catalysts that transform data into something useful and actionable.
What insights can we add to our O2C process?
Order Management
- Customer Order History: What is the customer’s historical order frequency and volume? This can help predict future orders and tailor marketing efforts.
- Product Affinity: Which products are frequently ordered together? This insight can inform cross-selling and upselling strategies.
Credit Management
- Payment Behavior: What is the customer’s track record for on-time payments? This can inform credit limit decisions.
Order Fulfillment
- Inventory Turnover: Which products have the highest and lowest turnover rates? This can optimize inventory management.
Order Shipping
- Carrier Performance: Which shipping carriers have the best on-time delivery rates? This can inform carrier selection.
Customer Invoicing
- Invoice Accuracy: What is the error rate in invoicing? This can help improve the invoicing process.
Accounts Receivable
- Days Sales Outstanding (DSO): What is the average time to collect payment? This can help improve cash flow management.
Payments Collection
- Preferred Payment Methods: Which payment methods do customers use most frequently? This can streamline the payment process.
Great, we have analyzed our data and have insights about them. But have we changed how our business operates? No, we simply have insights. This is where many organizations are in their analytic maturity today. They have insights about different aspects of their business, but they haven’t fundamentally acted upon those insights in any meaningful way. Next, let’s turn to AI.
What is Artificial Intelligence?
Before we can understand what it means to be AI-driven, we need to understand what AI is and isn’t. In the first TinyTechGuide, Artificial Intelligence: An Executive Guide to Make AI Work for Your Business, we defined AI as a fusion of data, analytics, and automation technology.
In other words,
Artificial Intelligence = Data + Analytic Insights + Automation Technology
What Does it Mean to Be AI-Driven?
Now that we have a pragmatic definition of AI and understand that a data-driven enterprise is focused on digitalization and an insight-driven enterprise is fundamentally looking to create insights, let’s compare it to an AI-driven enterprise looking to automate digital decisions.
Recall our AI definition from earlier? “Applies advanced analysis and logic-based techniques, including machine learning, to interpret events, support and automate decisions, and take actions.”
So, artificial intelligence is about making business decisions. It uses data, analytics, and automation to do this. Here are the significant differences between the different types of companies.
Data-Driven Companies
- Goal: Digitize data and digitalize processes
- Delivery Mechanism: Automation of some business process elements
- Decision-Making Style: Primarily manual, human-centric decision-making along with business rules to automate some decisions
- Decision Types: Mostly used for operational decisions
Insight-Driven Companies
- Goal: Deliver insights that inform business decisions
- Delivery Mechanism: Reports and dashboards which need to be interpreted by a human
- Decision-Making Style: Used in combination with subject matter expertise, intuition, and other support systems
- Decision Types: Used for a wide variety of decision types, strategic, tactical, and operational
AI-Driven Companies
- Goal: Deliver digital decisions that automate business actions
- Delivery Mechanism: Embedded in business processes, humans may be informed and can override if necessary
- Decision-Making Style: Depending on the use case, usually more automated, business outcomes are monitored
- Decision Types: Primarily used for high volume, automated, operational decisions
Now, with the above, these are different progressions along an AI maturity curve that companies progress through. They are similar, and more analytically mature companies have become AI-driven as they increase automation.
Why is it Important to be AI-driven?
Before an organization can become AI-driven, it needs a compelling vision and strong commitment from all stakeholders—especially the executive leadership team. For many companies, this is a multi-year journey, not a simple flip of the switch. It needs to be a core element of their business strategy. If they do not embark on this journey, they risk becoming irrelevant.
This irrelevancy will come from not being able to sense and respond to changing market conditions, a lethargic response to customer needs, a paralyzation of not being able to work well with your suppliers, and, unfortunately, the net result—you will not be able to deliver on your company’s promise to your customers. Thus, the time to act is now!
When the COVID-19 pandemic swept in in early 2020, everything changed overnight. Norms, habits, and ways of doing business changed. Individuals were locked down and required to work from home. Supply chains ground to a halt; the new normal was disruption. Today, economic uncertainty looms on the horizon; persistent inflation weighs heavily on minds; supply chains are strained, and geopolitical unrest are familiar headlines. With this ever-evolving dynamism, your company has probably accelerated digital transformation initiatives.
Companies were forced to go all in on AI, and many moved faster than they ever thought possible.
“During the pandemic, for example, Frito-Lay ramped up its digital and data-driven initiatives, compressing five years’ worth of digital plans into six months. “Launching a direct-to-consumer business was always on our roadmap, but we certainly hadn’t planned on launching it in 30 days in the middle of a pandemic,” says Michael Lindsey, chief growth officer at Frito-Lay. “The pandemic inspired our teams to move faster than we would have dreamed possible.”[10]
How has AI helped businesses? An article in HBR highlighted the following:[11]
- The pandemic has accelerated the adoption of AI and analytics
- Business leaders understand firsthand the power and potential of analytics and AI in their business
- AI is instrumental in alleviating skills shortages
- AI and analytics are boosting productivity
- AI and analytics are delivering new products and services
- AI accentuates corporate values
- AI and analytics are addressing supply chain issues
- AI is fueling startups while helping companies manage disruption
As time marches on, AI will only become more pervasive and embedded in everything a company does. Business leaders cannot do it alone, though. They need to involve everyone within their organization, partners, suppliers, customers, and the broader ecosystem.
Furthermore, if the gloom and doom don’t scare you, data-driven companies outperform non-data-driven companies across all dimensions—financial, customer, and people. For example, Harvard Business Review (HBR) studied leaders vs. laggards and found that leaders had 4.7 points higher revenue growth and 7.8 points higher CAGR compared to laggards.[12]
Figure 1.2: Revenue Growth and Growth Rates for Leaders vs. Laggards
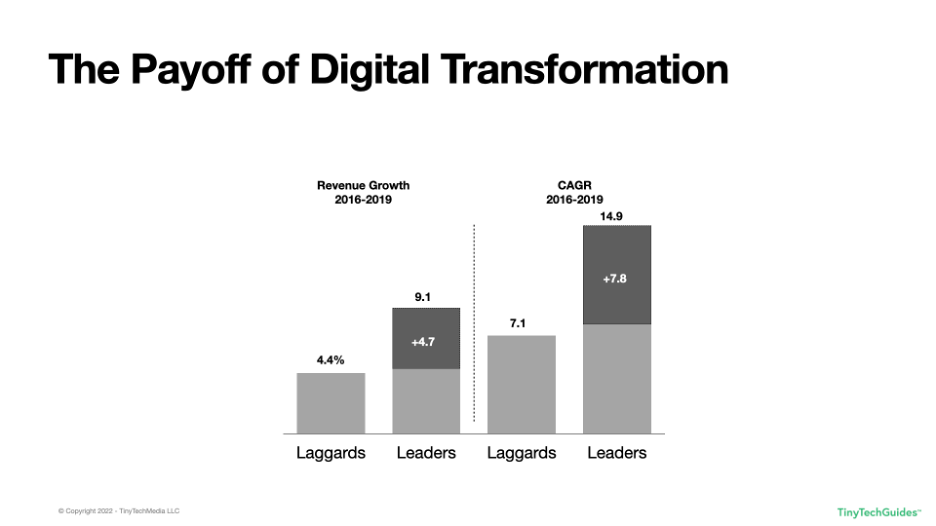
Conclusion
The journey to becoming an AI-driven organization is not just a technological shift, but a fundamental transformation in how businesses operate, compete, and create value. As we’ve explored, this evolution progresses from data-driven to insight-driven, and ultimately to AI-driven operations–each stage building upon the last to unlock new capabilities and opportunities.
The imperative for this transformation is clear. AI-driven organizations have a distinct advantage in today’s rapidly changing business landscape, characterized by economic uncertainty, supply chain disruptions, and evolving customer expectations. They can sense and respond to market changes quickly, make more informed decisions at scale, and deliver personalized experiences that drive customer loyalty and revenue growth.
UBS and McDonald’s examples demonstrate that AI adoption is possible and beneficial across diverse industries, even in highly regulated environments. These success stories underscore the versatility and power of AI in enhancing operational efficiency, improving decision-making, and driving innovation.
However, the path to becoming AI-driven is not without challenges. As highlighted by the NewVantage Partners survey, cultural change remains the biggest hurdle for many organizations. This underscores the need for strong leadership, a clear vision, and a commitment to fostering a data-centric culture throughout the organization.
The rewards for overcoming these challenges are substantial. AI-driven companies consistently outperform their peers in revenue growth, profitability, and adaptability. They are better positioned to navigate disruptions, capitalize on new opportunities, and create innovative products and services that meet evolving market needs.
As we look to the future, the pervasiveness of AI in business operations will only increase. Organizations that fail to embrace this shift risk falling behind more agile, AI-enabled competitors. The time to act is now. You can begin the journey towards becoming an AI-driven organization by assessing your current AI maturity, developing a clear roadmap, and focusing on high-impact use cases.
Becoming AI-driven is not just about adopting new technologies; it’s about rejiggering how your business operates in a data-rich, algorithmically optimized world. This journey promises to enhance decision-making, drive efficiency, and unlock new sources of value. As the business landscape continues to evolve, embracing AI is not just an option—it’s an imperative for sustained success and competitiveness in the digital age.
If you enjoyed this article, please like it, highlight interesting sections, and share comments. Consider following me on Medium and LinkedIn.
Please consider supporting TinyTechGuides by purchasing any of the following books.
- Generative AI Business Applications: An Exec Guide with Life Examples and Case Studies
- Artificial Intelligence: An Executive Guide to Make AI Work for Your Business
- The CIO’s Guide to Adopting Generative AI: Five Keys to Success
- Mastering the Modern Data Stack
- Modern B2B Marketing: A Practioner’s Guide for Marketing Excellence
[1] Gibney, Elizabeth. 2022. “How Many Yottabytes in a Quettabyte? Extreme Numbers Get New Names.” Nature, November. https://doi.org/10.1038/d41586-022-03747-9.
[2] Transcribing, Motley Fool. 2021. “Alteryx (AYX) Q2 2021 Earnings Call Transcript.” The Motley Fool. August 4, 2021. https://www.fool.com/earnings/call-transcripts/2021/08/04/alteryx-ayx-q2-2021-earnings-call-transcript/.
[3] Sweenor, David. 2022. “Analytics Democratization vs. Governance: Are They at Odds?” Medium. September 27, 2022. https://medium.com/@davidsweenor/analytics-democratization-vs-governance-are-they-at-odds-378c50258261.
[4] Yaffe-Bellany, David. 2019. “Would You like Fries with That? McDonald’s Already Knows the Answer.” New York Times, October 23, 2019. https://www.nytimes.com/2019/10/22/business/mcdonalds-tech-artificial-intelligence-machine-learning-fast-food.html.
[5] Bean, Randy. 2024. “2024 Leadership and AI Survey Analysis: What Data Leaders Need to Know.” Data Leadership Collaborative. February 6, 2024. https://www.dataleadershipcollaborative.com/data-leadership/2024-leadership-and-ai-survey-analysis-what-data-leaders-need-know.
[6] Davenport, Thomas, and Randy Bean. 2022. “Data and AI Leadership Executive Survey 2022: Executive Summary of Findings.” NewVantage Partners A Wave Stone Company. https://www.newvantage.com/_files/ugd/e5361a_ad5a8b3da8254a71807d2dccdb0844be.pdf.
[7] Merriam-Webster. 2019. “Definition of DATA.” Merriam-Webster.com. 2019. https://www.merriam-webster.com/dictionary/data.
[8] Gartner. 2012. “Digitalization.” Gartner. 2012. https://www.gartner.com/en/information-technology/glossary/digitalization.
[9] Wong, Danny. n.d. “What You Should Know about the Order-To-Cash Process.” Salesforce.com. Accessed December 17, 2022. https://www.salesforce.com/products/cpq/resources/what-to-know-about-order-to-cash-process/.
[10] McKendrick, Joe. 2021. “AI Adoption Skyrocketed over the Last 18 Months.” Harvard Business Review, September 27, 2021. https://hbr.org/2021/09/ai-adoption-skyrocketed-over-the-last-18-months.
[11] McKendrick, Joe. 2021. “AI Adoption Skyrocketed over the Last 18 Months.” Harvard Business Review, September 27, 2021. https://hbr.org/2021/09/ai-adoption-skyrocketed-over-the-last-18-months.
[12] Iansiti, Marco, and Satya Nadella. 2022. “Democratizing Transformation.” Harvard Business Review. May 1, 2022. https://hbr.org/2022/05/democratizing-transformation.