Robert Lake on asking the three essential business questions, managing the human tendency to anthropomorphize, and leading effective AI change management
Listen now on YouTube | Spotify | Apple Podcasts
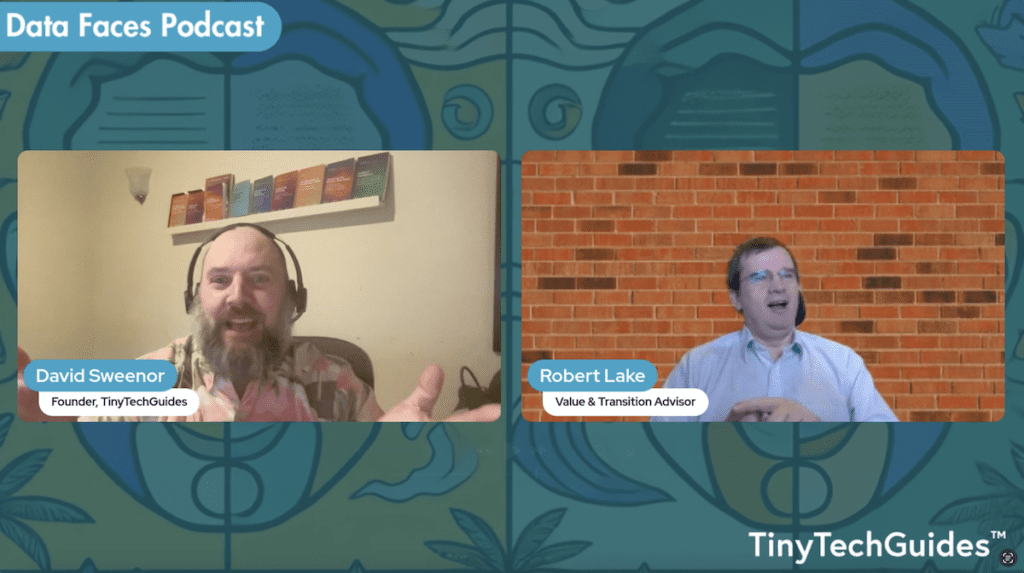
Be sure to sign up for prompts.tinytechguides.com to get the latest stories delivered directly to your Inbox.
Trust in AI isn’t just a technical challenge; it’s a human one. As businesses rush to implement AI, they face a disconnect between the probabilistic nature of these technologies and the certainty demanded by executives making million-dollar decisions. This tension creates the perfect environment for misaligned expectations, failed implementations, and eroded confidence.
About Robert Lake
Robert Lake brings over 30 years of data science experience to this challenge. As owner of Trebor Strategic Advisors, he coaches businesses on implementing AI effectively while preparing for successful exits. Previously leading data science organizations and guiding multiple companies through exits, Lake combines technical expertise with business pragmatism.
AI trust is one of the worst understood areas of what we do… when you build an algorithm to [connect with people], it’s a manipulation, because what we’re doing is we’re deliberately forcing that connection. – Robert Lake
In our conversation, Lake weighs in on why businesses struggle with AI’s probabilistic nature, our tendency to anthropomorphize technology, and why leadership must anchor AI implementation in core values rather than technological capabilities.
The Probabilistic Nature of AI vs. Business Expectation of Certainty
One of the greatest disconnects in AI implementation happens when business leaders expect definitive answers from inherently probabilistic systems. Most executives want fixed outcomes they can rely on, but AI delivers probabilities and ranges instead.
“Even though he wanted that sensitivity, that variation being shown, he just could not get past the point of having fixed outcomes coming out of algorithms and not having a range.” – Robert Lake
Lake recalls a senior vice president he worked with who called him an “Excel model expert” but couldn’t grasp that models produce ranges, not certainties. This executive wanted Lake to “predict anything you want to be able to do, you just need to hire a priest.”
This disconnect becomes especially problematic with consumer-facing AI systems that hide their uncertainty levels. When using ChatGPT or similar tools, users receive definitive-sounding answers with no indication of confidence levels for accuracy, which might be as low as 30%.
For product teams implementing AI, Lake recommends a verification approach: frame questions where you already have a general sense of what the answer should be, then evaluate if the AI’s response falls within that expected range. This creates a practical reality check on AI outputs that teams can implement immediately.
The Human Element: Why We Anthropomorphize AI
Throughout history, humans have displayed two consistent tendencies: seeking efficiency and humanizing technology. “We’ve always tried to find the easiest, fastest way of doing something,” Lake explains. This drive has produced technologies from water wheels to modern AI.
Simultaneously, we anthropomorphize our tools. This tendency creates dangerous misunderstandings about AI’s capabilities, especially in customer-facing applications.
Lake uses image recognition as a concrete example: “It has no idea it’s you on the screen right now, we have no idea that it’s your face. It’s just a guess… The image doesn’t know that. It just knows colors, three dimensions of color, and shapes.”
We humanize everything, right? Because we’re such a social animal… it’s actually natural to expect people to start to adopt AI that way. – Robert Lake
For product and marketing leaders, this creates an important ethical consideration: how do you present AI capabilities honestly while managing user expectations? Lake warns that exaggerating AI’s “humanness” can have severe consequences, citing a case of a teenager who took his life after developing an emotional dependency on an AI chatbot.
Product teams should consider implementing explicit “this is not human” reminders in AI interfaces where emotional connection might develop, while marketing should avoid anthropomorphic language that could mislead users about system capabilities.
Starting with Core Values: The Foundation of AI Accountability
Accountability for AI outcomes must trace back to leadership and core values, not to technical teams working in isolation.
“It always has to come back to the owner, or the CEO, the board, whoever runs the company,” Lake explains. Without this leadership direction, technical teams might build impressive systems that don’t align with business needs.
It has to start with leadership. And ultimately, the top of the leadership pyramid of what it is we’re trying to do from a business perspective. How do we believe this is going to help us? – Robert Lake
Organizations implementing AI need explicit strategic direction connecting AI initiatives to specific business objectives: “Here’s where we’re going. We are going to be working in this market space with this client avatar, and here are our products that we’re serving. Here’s the problems where we’re trying to solve.”
For leadership teams, Lake offers a practical test to determine if stated values are actually driving decisions: “Show me where you’ve hired or fired someone based on the core value. Show me how you made a business decision on that. If you haven’t, it’s not a core value.”
This accountability framework gives teams clear parameters for AI development, prevents scope creep, and ensures alignment with organizational objectives before any technical work begins.
Creating AI Systems That Build Long-Term Confidence
Building trustworthy AI systems requires applying established business principles rather than inventing entirely new frameworks. Lake emphasizes that continuous testing, improvement, and customer feedback cycles apply directly to AI development.
For product teams, this means implementing specific monitoring metrics for AI performance, creating feedback loops for continual refinement, and, most importantly, focusing relentlessly on customer needs rather than technological capabilities.
AI is nothing different from anything else you do as a business. You need to monitor it. You need to track it, you need to improve it, and you need to get feedback… customer is king. – Robert Lake
Lake recommends that teams identify “three to five pain points” their customers face, then evaluate AI implementations based on how effectively they address these specific problems.
This disciplined approach prevents the common pitfall of rapidly escalating costs without clear ROI. Lake points to Microsoft’s Copilot as an example where per-user costs can increase “from 15 to 20 bucks a month to 40 to 50 to 60 to 70 to 80 to 100 bucks a month per user” without necessarily delivering proportional value.
IT and data science leaders should establish clear cost-benefit metrics before implementation and regularly reassess based on actual performance data rather than theoretical capabilities.
Executive AI Literacy: What Leaders Actually Need to Know
How much should executives understand about AI technologies? Lake takes a pragmatic approach, focusing on business outcomes rather than technical minutiae.
Lake begins leadership interviews with a surprising statement: “I am going to be the first person to tell you not to use AI… I am going to tell you when you don’t need it.” This contrarian stance emphasizes that AI should solve specific business problems, not simply showcase technological capabilities.
I am going to be the first person to tell you not to use AI… I am going to tell you when you don’t need it, and we need more people to do that. – Robert Lake
Rather than deep technical knowledge, executives should focus on three fundamental questions when evaluating AI implementations:
- Does it help us make money?
- Does it help us save money?
- Does it keep us out of legal trouble?
Lake warns leaders against “shiny object syndrome,” where impressive demonstrations lead to implementations without clear business justification. He recommends establishing formal challenger roles within AI evaluation processes to prevent echo chambers of enthusiasm.
For organizations building AI capabilities, this means creating evaluation frameworks that begin with business outcomes rather than technical specifications, and establishing governance processes that can confidently reject misaligned proposals despite their technological impressiveness.
Trust Through Change Management: Leading AI Implementation
Successfully implementing AI requires effective change management focused on building trust through clear communication of purpose.
If you don’t start with the why, you don’t build trust. If people don’t understand why they’re doing something, they don’t have trust. – Robert Lake
Without clear explanations of purpose, employees affected by AI implementations may develop anxiety about job security or other negative outcomes. Lake recommends explicit communication about how AI will impact roles, workflows, and objectives before technical implementation begins.
For evaluating potential applications, Lake suggests using a “creepy meter” to identify implementations that might violate social norms or privacy expectations. He cites an example of an inappropriate application: “The moment you walk into the store, the video monitor in front of you will show your face and say, ‘Hey, Robert, how you doing? By the way, the condoms are this direction.'”
For a simpler everyday evaluation framework, Lake offers what might be called the “grandmother test”:
My grandma used to say, when you’re out there in the world, ask yourself, what would your nana say?… Think about that when you want to do AI. – Robert Lake
This simple heuristic helps teams evaluate potential implementations against broader social and ethical considerations beyond pure technical capability or business advantage.
Balancing Innovation with Responsibility
Building trustworthy AI systems requires balancing innovation with responsibility. Organizations must establish clear ethical boundaries based on core values while focusing implementation on specific business problems rather than technological capabilities.
For data science, IT, and business leaders looking to build trustworthy AI, focus on these top priorities:
- Start with your business strategy and specific customer needs
- Establish explicit accountability from leadership downward
- Apply the “grandmother test” to potential ethical questions
Don’t allow people to do this shiny object thing… you’ve got to come back to: Does it help me make money? Does it help me save money? Does it keep me out of jail? – Robert Lake
Take a moment to assess your current AI implementations against these criteria. Are your systems addressing specific customer pain points? Can you trace accountability clearly back to leadership? Would your applications pass both the “creepy meter” and “grandmother” tests?
By following these principles, organizations can build AI systems that earn trust through demonstrable value, ethical implementation, and alignment with core business objectives rather than chasing technological trends without clear purpose.
If you’re interested in learning more about AI or how you can use generative AI, check out these TinyTechGuides.
Generative AI Business Applications: An Executive Guide with Real-Life Examples and Case Studies
Artificial Intelligence: An Executive Guide to Make AI Work for Your Business
Or the full series:
TinyTechGuides (7 book series) Paperback Edition
Edited Transcript Highlights: Building Trust in AI with Robert Lake
00:05 – Introduction David Sweenor: “Welcome to the Data Faces podcast that brings together the human stories behind data analytics and AI to the forefront. In today’s episode, we’re going to talk about one of the most important topics in AI: trust. What does it mean to trust a machine or a system? How do organizations build confidence in systems that are complex, evolving and sometimes opaque?”
01:04 – Robert’s Background Robert Lake: “I’ve been doing data science for over 30 years, way before the actual term data scientist came out. I’ve transitioned now from corporate world into business coaching, particularly helping people exit. One thing I’ve always found in the advanced analytics arena and AI arena is people tend to paper over their business problems, hoping that AI or advanced analytics will fix the problems magically.”
02:54 – The Challenge of Probabilistic AI Robert Lake: “This is one of the worst understood areas of what we do. I used to make models in Excel for a senior vice president. He used to introduce me as his Excel model expert, which used to drive me crazy. He also said, ‘He can build a model for anything you want and predict anything, you just need to hire a priest.’ He could not get past the point of having fixed outcomes coming out of algorithms and not having a range.”
04:38 – Verification Approach Robert Lake: “One of the things I try to help people understand is you can’t trust everything you see there. You need to verify it. Think of it as: I have a question. I think I know what the answer should look like. I’m going to propose a question, and does it give me an answer in the area I think it is?”
11:53 – Anthropomorphizing AI Robert Lake: “We’ve always tried to find the easiest, fastest way of doing something. We humanize everything because we’re such a social animal. It’s actually natural to expect people to start to adopt AI that way. But what you have to understand is, when we as humans are talking to each other, we’re engaging, connecting, and constantly sensing all the time. The trouble is, when you build an algorithm to do that, it’s a manipulation.”
16:52 – Business Accountability David Sweenor: “Explainability and accountability. Where does it begin in an enterprise system, and sort of who owns this? Is it the designer of the system? The designer of the models? The company running the system?”
17:33 – Leadership Responsibility Robert Lake: “It always has to come back to the owner, or the CEO, the board, whoever runs the company. It’s really easy to give someone who’s really excited about something and they build something, and it’s like, ‘ta-da, I built something.’ Like, ‘yeah, that looks great, but how we’re going to use it?’ It has to start with leadership.”
20:38 – Building Trust Systems David Sweenor: “How do you build this sort of repeatable production system that builds long-term confidence in the AI systems being built?”
21:12 – Continuous Improvement Robert Lake: “Let’s go back to the basics. Lean Six Sigma – constant, continuous improvement. You need to be constantly testing, constantly improving, constantly gaining feedback. Customer is king, not your AI engineers, not your developers, not your sales team or your marketing team. If your customers don’t need this or don’t want this, why are we doing it?”
29:52 – Executive Understanding Robert Lake: “When I go interview for leadership roles and talk to CEOs, I always make them laugh with this comment. They’ll say, ‘Why should I hire you as my AI leader?’ And I say, ‘Real simple, I am going to be the first person to tell you not to use AI.’ They look at you—’What?’ Yeah, I am going to tell you when you don’t need it.”
34:15 – Leading Organizational Change Robert Lake: “Simon Sinek says it the best: start with the why. If you don’t start with the why, you don’t build trust. If people don’t understand why they’re doing something, they don’t have trust. The challenging of the why is not a challenge of your authority or your leadership. It’s allowing someone to understand.”
38:30 – Final Thoughts Robert Lake: “My grandma used to say, when you’re out there in the world, ask yourself, what would your nana say? Think about that when you want to do AI. It sounds cool for me right now, but what would someone else say? Does that meet my core values? Am I there to manipulate people, to force people into doing something?”
About the David Sweenor
David Sweenor is an AI, Generative AI, and Product Marketing Expert. He brings this expertise to the forefront as founder of TinyTechGuides and host of the Data Faces podcast. A recognized top 25 analytics thought leader and international speaker, David specializes in practical business applications of artificial intelligence and advanced analytics.
Books
- Artificial Intelligence: An Executive Guide to Make AI Work for Your Business
- Generative AI Business Applications: An Executive Guide with Real-Life Examples and Case Studies
- The Generative AI Practitioner’s Guide: How to Apply LLM Patterns for Enterprise Applications
- The CIO’s Guide to Adopting Generative AI: Five Keys to Success
- Modern B2B Marketing: A Practitioner’s Guide to Marketing Excellence
- The PMM’s Prompt Playbook: Mastering Generative AI for B2B Marketing Success
With over 25 years of hands-on experience implementing AI and analytics solutions, David has supported organizations including Alation, Alteryx, TIBCO, SAS, IBM, Dell, and Quest. His work spans marketing leadership, analytics implementation, and specialized expertise in AI, machine learning, data science, IoT, and business intelligence.
David holds several patents and consistently delivers insights that bridge technical capabilities with business value.Follow David on Twitter @DavidSweenor and connect with him on LinkedIn.