Why AI Can’t Read Your Messaging On a Page (and the Simple Fix Every PMM Needs)
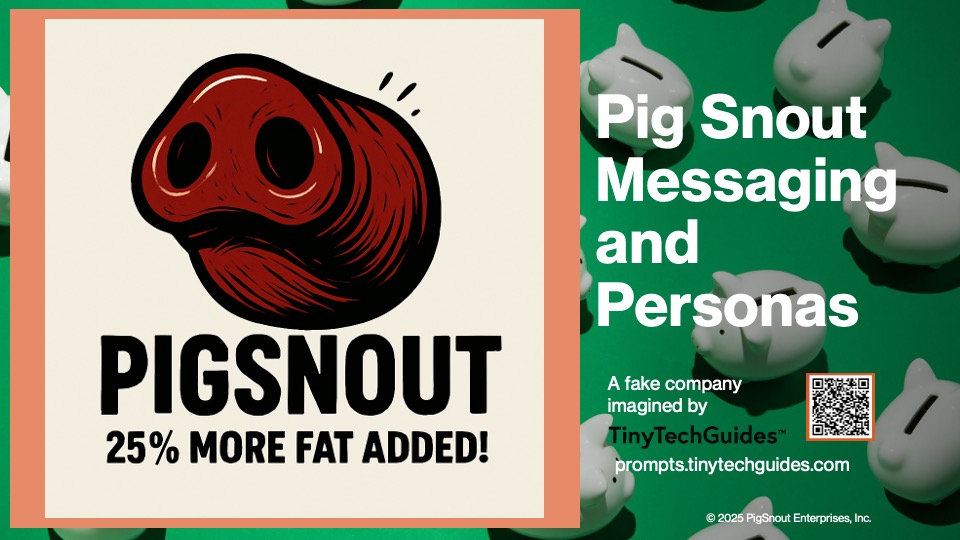
Last week, I worked with a client, using my AI superpower to augment competitor research and create content based on their messaging framework. To ensure alignment, I had made a custom GPT loaded with their public case studies, key whitepapers, custom research, and messaging framework. But something seemed off about the content it generated.
It wasn’t on message, so I started asking direct questions about key value propositions, differentiators, and proof points in their messaging on a page framework. For some reason, the LLM couldn’t answer these fundamental questions. What of that? When I finally got to the root of the problem, I discovered it wasn’t using the messaging framework that was definitely in its knowledge base. Why? Well, like most good marketers, they had created a messaging-on-a-page that was in a table in PowerPoint. Despite multiple attempts with different formats and prompts, the LLM couldn’t accurately process what was plainly visible to human readers.
It’s like the LLM was missing the pepperoni on a pizza – those savory slices that stand out as the most flavorful, valuable bits. In my house, someone always tries to take one of the crunchy slices right off the pizza! Similarly, in your messaging frameworks, the points of distinction (value pillars) form the essential context needed to create unique, distinctive, and on-message content targeted towards the personas you’re after.
Despite all the hubbub surrounding LLMs and their supposed ability to ‘reason,’ these ‘intelligent’ systems can’t even read a basic table correctly—it’s a real problem for PMMs.
To illustrate this with a concrete example, I’ve created a fictional company called PigSnout Enterprises, Inc., makers of the world-famous PigSnout Pepperoni Chips. Why did I choose this? Well, several years ago, when the latest health craze was at its apogee, I told my friends and family that I would start the PigSnout Pepperoni Chips company with an “extra fat added.”
When LLMs can’t see or interpret your tables, they create completely off-message content, introduce misalignment, make up case study quotes that don’t exist, and deliberately waste your time as you repeatedly correct their output. If you’re using AI tools to help scale your content creation, you need to understand this limitation before it undermines your carefully crafted messaging.
The Challenge of Tables for LLMs
Humans love tables. They help us organize complex information, show relationships between concepts, and quickly compare data points. That’s precisely why PMMs use them for messaging frameworks and competitive comparisons. Tables let us pack a lot of structured information into a compact, easily consumable, snackable format.
LLMs, however, have a fundamental mismatch with how they process information. While humans easily understand the two-dimensional nature of tables, LLMs process text sequentially, essentially flattening your carefully structured information.
Your carefully structured messaging table might be invisible ink to LLMs – technically present in the document but entirely imperceptible to the AI’s understanding.
According to a 2024 study by Liu et al. in “Rethinking Tabular Data Understanding with Large Language Models,” LLMs often struggle with tables. The authors note, “we discover that structural variance of tables presenting the same content reveals a notable performance decline, particularly in symbolic reasoning tasks.[1]” In plain English: while these AI systems can write poetry and code, they can’t read tables worth a boo.
Here’s the information that ChatGPT read from the above slide on page 2 of the PPTX file. Notice the fact that it doesn’t “see anything”? Also, if the page has an image, it doesn’t see that. Claude does, though.
The problem is even more basic than you might think. Some LLMs have difficulty with tasks as simple as accurately identifying the number of columns and rows in a table. They literally can’t count the cells correctly.
Claude refused to read the PPTX file.
However, Gemini could not see the table either.
Format matters significantly here. Microsoft Research’s 2024 work on “Improving LLM understanding of structured data and exploring advanced prompting methods” found that HTML and Markdown formats typically perform better than CSV or plain text[2]. But even with the best formats, there’s still a significant gap in how well LLMs interpret tabular data compared to human readers.
Here are the results of my little experiment:
ChatGPT | Gemini | Claude | |
PPTX | Did not see the table | Did not see the table | Refused to read PPTX |
Google Slides | Confused page numbers, but extracted content. Cell content is not associated with one another. | Confused page numbers, extracted content. Cell content is not associated with one another. | Did not see the file in Google Drive |
Similar to Google Slides | Extracted content, put a bunch of “ and, in the output. Cell content is not associated with one another. | Extracted some content on the first pass, did a better job on the second pass, and associated the value pillars with the differentiators. |
Claude did the best job of extracting the PDF.
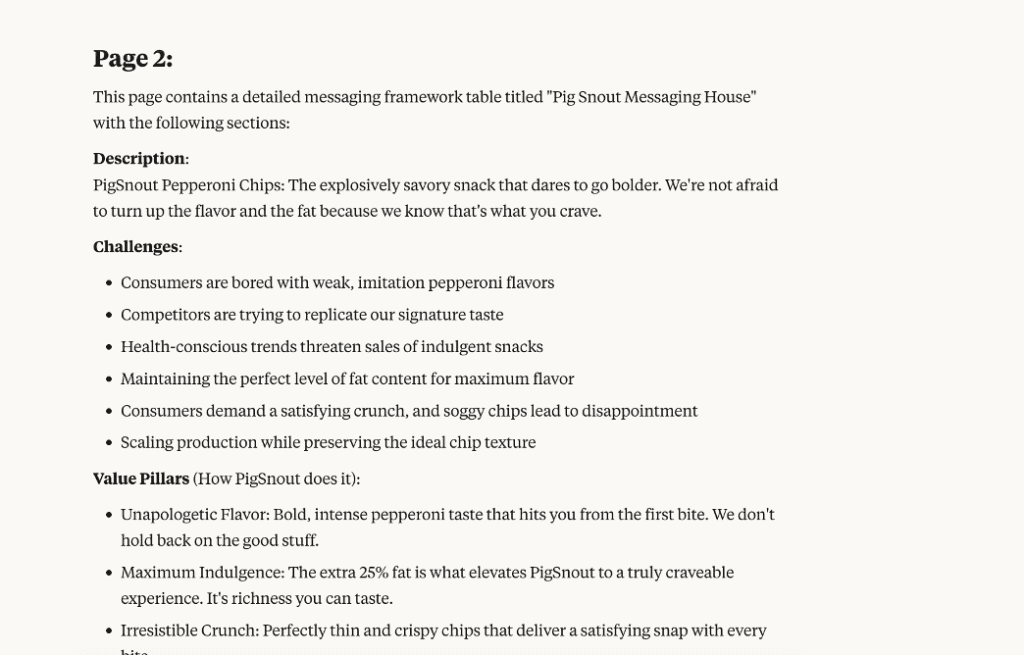
Impact on Product Marketing
When LLMs misinterpret your tables, the consequences for product marketing are significant. Here are the impacts, ranked from most to least critical:
Off-message content tops the list of problems. When LLMs can’t “see” messaging frameworks stored in tables, they create content that does not align with the approved positioning and messaging you worked so hard to create. Your carefully crafted talking points become invisible, and the AI starts making up its own narrative about your products.
It’s the digital equivalent of playing ‘telephone’ with your product or platform messaging—what comes out at the other end maintains the confidence of the original but bears little resemblance to your intended message.
Brand voice inconsistency creeps in as another concern. Those tone guidelines you organized in a neat table? The LLM can’t see them correctly, which leads to generic-sounding AI outputs that lack your brand’s distinctive voice.
Fabricated information starts appearing in your AI-generated content. LLMs may “hallucinate” case studies or testimonials when they can’t access your actual customer success data in tables. Suddenly, your content includes quotes from customers that don’t exist or product benefits you never claimed.
Competitive positioning errors round out the major problems, undermining your market differentiation. When your competitive analysis lives in tables, the LLM misses critical differences between your offering and the competition.
The real-world consequence? PMMs end up manually reviewing and correcting AI-generated content, negating the efficiency benefits of using LLMs in the first place. What should save you time ends up creating more work.
Take our fictional PigSnout Enterprises example. The carefully crafted messaging framework for pepperoni chips becomes inaccessible and completely invisible in table format. Instead of emphasizing their unique cooking process and premium ingredients, the LLM might focus on generic snack food benefits or even invent product features that don’t exist.
Solutions and Workarounds
The most effective solution I’ve found is straightforward: maintain parallel document versions of your key marketing assets specifically for LLM consumption. I call this the “Doc Approach,” which works remarkably well.
The Doc Approach isn’t about dumbing down your content—it’s about translating it into a language LLMs can understand. Think of it as creating a pepperoni pizza that everyone can enjoy.
Here’s how this looks in practice. When creating your messaging framework as a beautifully formatted table in your presentation deck, simultaneously create a Word or Google document version.
Pro tip:
LLMs do a better job of extracting information from PDF tables than PPTX tables.
Best format practices:
- Markdown (MD) format is one of the best options for LLM readability
- Be careful when exporting from PowerPoint or Google Slides – many export options (like RTF) drop tables entirely and don’t export as MD format.
- When exporting from presentation software, you’ll need to recreate the table structure in Markdown manually.
For practical implementation, create a “For LLM Use” folder in your marketing asset repository. When developing new frameworks, create both versions simultaneously. For existing assets, prioritize converting your most frequently referenced messaging frameworks first.
When formatting your LLM-friendly docs:
- Replace complex tables with bulleted hierarchies when possible
- If tables are necessary, use simple HTML tables in Google Docs or Markdown tables in text files
- Include text descriptions of relationships between concepts
- Label all sections clearly for easy reference by the LLM
Your workflow should look like this:
- Identify your critical marketing assets that contain tables
- Create document versions with the same information in LLM-friendly formats
- Test these documents with your preferred LLM
- Update your team’s workflow to maintain both versions going forward
Make sure to assign ownership to maintain these parallel document versions as part of your content governance process. Someone must be responsible for keeping both versions in sync when updates occur.
When developing brand guidelines for LLM use, create a separate text-focused version alongside your designed PDF. This ensures your AI tools can access your brand voice guidelines properly.
Testing Your Tables with LLMs
Before you invest time converting all your tables, it’s worth testing how well your current formats perform with LLMs. This lets you prioritize which documents need immediate attention.
Testing your tables with LLMs is like asking someone to repeat your directions back to you. If they get it wrong, you need to find a different way to explain.
Here’s a simple checklist to test your tables:
Verification Prompts:
- “Please extract all information from the table above and present it in bullet point format.”
- “How many rows and columns are in the table I shared?”
- “What is the content of row 3, column 2?”
Warning Signs to Watch For:
- Incorrect row or column counts
- Information from different cells combined or mixed up
- Missing rows or columns
- Invented information not present in your table
- Headers confused with content
Try an A/B testing approach with different formats:
- Test your original table format
- Convert to a simple HTML table and test again
- Try a Markdown format table
- Test a bulleted list version of the same information
Compare the results to determine which format your LLM processes most accurately. You’ll likely find significant differences in comprehension between formats.
For example, with our PigSnout Pepperoni Chips messaging framework, a bulleted hierarchy might perform better than the original table. Instead of rows and columns, organize by primary message, supporting points, and proof points in a nested list format that maintains the relationships between concepts while being more LLM-friendly.
By identifying which tables your LLM struggles with most, you can prioritize your conversion efforts and ensure your most critical marketing assets are accessible to your AI tools.
Don’t Let Your AI Miss the Pepperoni: Next Steps
LLMs currently have a significant blind spot when it comes to reading marketing tables, frameworks, and structured data. As a product marketer relying on AI tools, you must adapt your content formats to ensure your key messaging remains accessible.
The difference between an LLM that misses your messaging and one that nails it isn’t the AI—it’s how you package your information for consumption.
Your immediate action item: Test your top three most critical marketing frameworks with your preferred LLM today. See how well the AI interprets your tables, and if it struggles, apply the Doc Approach to create LLM-friendly versions.
Simple format changes can dramatically improve how well AI systems understand your structured marketing content. The small investment in creating LLM-friendly versions of your key marketing assets will pay off through more accurate, on-message content that truly represents your brand.
Just as PigSnout Enterprises wouldn’t hide their pepperoni chips in hard-to-open packaging, don’t hide your valuable marketing frameworks in formats LLMs can’t digest. When your AI assistant can clearly see all the pepperoni, it’ll create content that delivers the full flavor of your messaging.
About the Author
Books: Artificial Intelligence | Generative AI Business Applications | The Generative AI Practitioner’s Guide | The CIO’s Guide to Adopting Generative AI | Modern B2B Marketing
Founder of TinyTechGuides, David Sweenor is a top 25 analytics thought leader and influencer, international speaker, and acclaimed author with several patents. He is a marketing leader, analytics practitioner, and specialist in the business application of AI, ML, data science, IoT, and business intelligence.
With over 25 years of hands-on business analytics experience, Sweenor has supported organizations including Alation, Alteryx, TIBCO, SAS, IBM, Dell, and Quest, in advanced analytic roles.
Follow David on Twitter @DavidSweenor and connect with him on LinkedIn https://www.linkedin.com/in/davidsweenor/.
[1] Liu, Tianyang, Fei Wang, and Muhao Chen. 2024. “Rethinking Tabular Data Understanding with Large Language Models.” In Proceedings of the 2024 Conference of the North American Chapter of the Association for Computational Linguistics.
[2] Sui, Yuan, Mengyu Zhou, Mingjie Zhou, Shi Han, and Dongmei Zhang. 2024. “Table Meets LLM: Can Large Language Models Understand Structured Table Data? A Benchmark and Empirical Study.” Microsoft Research. March 4, 2024. https://www.microsoft.com/en-us/research/publication/table-meets-llm-can-large-language-models-understand-structured-table-data-a-benchmark-and-empirical-study/.