Exploring the current state of AI agents, their challenges, and how businesses can prepare for widespread adoption.
Listen now on YouTube | Spotify | Apple Podcasts
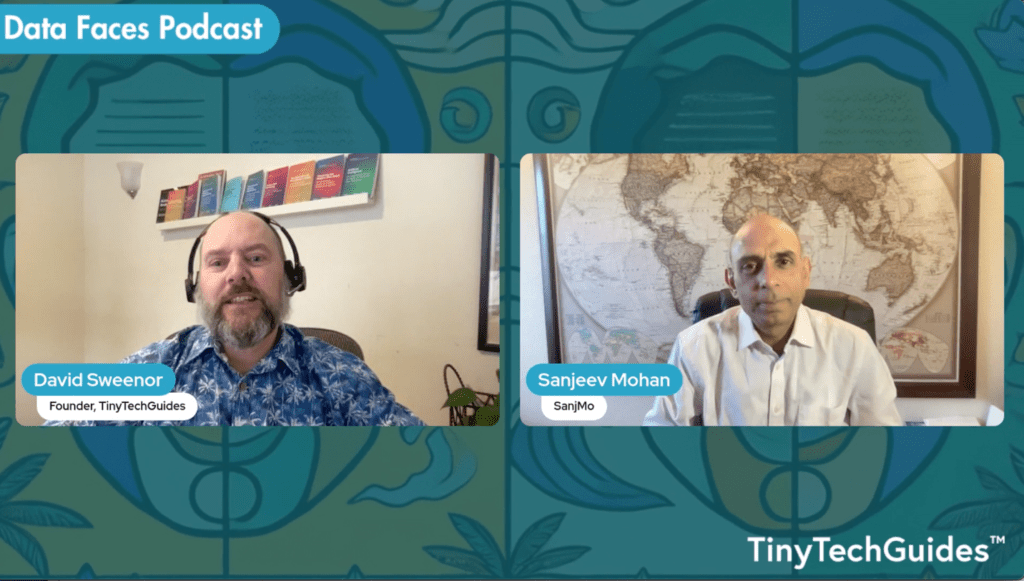
The journey from daemons to robotic process automation (RPA) to chatbots has been a steady march toward smarter, more autonomous systems. Today, we stand at the next frontier: AI agents. These systems don’t just follow rules or respond to queries—they understand context, reason through problems, and take action autonomously. In this episode of the Data Faces Podcast, we explore this evolution with Sanjeev Mohan, a leading expert in data and AI.
About the Speaker
Sanjeev Mohan is the Principal at SanjMo and a former Gartner Research VP, where he led the data and AI practice. With decades of experience helping organizations adopt data strategies, Sanjeev now focuses on researching and analyzing emerging technologies at the bleeding edge of AI. He is a prolific writer, speaker, and advisor, helping businesses navigate the rapidly evolving AI landscape.
In this episode, Sanjeev shares his perspective on the current state of AI agents, their challenges, and how businesses can prepare for their widespread adoption.
Understanding AI Agents
AI agents represent the next step in the evolution of automation. Early systems like daemons (background processes that perform specific tasks) and RPA were limited to predefined rules and workflows. Chatbots added a layer of interactivity, allowing users to ask questions and receive answers. But AI agents go further—they don’t just respond; they act.
What Are AI Agents?
AI agents are autonomous systems that understand context, reason through problems, and take actionable steps. Unlike chatbots or RPA, they don’t just follow rules—they make decisions and execute tasks on your behalf.
For example, imagine an AI agent that scans your inbox, identifies urgent client requests, and automatically reschedules meetings to accommodate them—all without human intervention. In supply chains, AI agents can monitor inventory levels, predict shortages, and reorder supplies autonomously, reducing delays and costs.
Unlike their predecessors, AI agents are designed to operate independently, making decisions and executing tasks on your behalf. This autonomy is what sets them apart and makes them so powerful.
Current State of AI Agents
AI agents are still in their early stages, but they’re already showing promise in specific use cases. However, they come with challenges—most notably, issues like hallucination (incorrect or nonsensical outputs) and reliability. For now, they’re best suited for tasks where errors are less critical.
Hallucination occurs when an AI agent generates incorrect or nonsensical outputs. For example, an AI agent might misinterpret a client email and take an inappropriate action, like rescheduling a meeting to the wrong time.
As Sanjeev Mohan explains, “AI agents are not ready to run an entire supply chain yet. They’re still dealing with problems like hallucinations, which can lead to costly mistakes. But everything is moving at warp speed—what’s a limitation today could be solved tomorrow.”
One area where AI agents are already making an impact is competitive intelligence. Companies are using AI agents to scan the web for market trends, analyze competitors’ websites, and summarize findings for executives. For instance, an AI agent might crawl through thousands of documents, extract key insights, and present them in a digestible format—saving hours of manual work.
Sanjeev shares an example: “Imagine an AI agent that acts as your research assistant. Every day, it goes out on the internet, crawls through competitor websites, summarizes their strengths and weaknesses, and presents you with a SWOT analysis. It’s a game-changer because it frees up your time to focus on strategy.”
Another promising use case is research assistance. AI agents can sift through vast amounts of data, identify patterns, and generate reports, freeing up human analysts to focus on strategic decision-making. For example, an AI agent could analyze customer feedback across multiple channels, identify common pain points, and recommend actionable improvements.
However, the rapid pace of AI development means that today’s limitations could be resolved quickly. What seems like a challenge today—such as reliability issues—might be addressed in months, not years. This makes it crucial for businesses to stay informed and adaptable.
Challenges Organizations Face with AI Agents
While AI agents hold immense potential, their adoption comes with significant challenges. Organizations must navigate issues like reliability, cost, data privacy, and governance to ensure these systems deliver value without unintended consequences.
Reliability is critical for AI agents. If they hallucinate or make incorrect decisions, the downstream impact can be severe—like a supply chain disruption or a damaged client relationship.
As Sanjeev Mohan points out, “Reliability is the biggest challenge. If an AI agent hallucinates or makes a wrong decision, it can screw up everything downstream. You can’t afford that in mission-critical tasks.”
One of the most pressing concerns is cost. An army of autonomous AI agents can quickly escalate expenses, especially if they execute tasks without proper oversight. For example, an AI agent might autonomously reorder supplies, leading to budget overruns if not carefully monitored. Sanjeev emphasizes this: “Cost is a huge factor. If an AI agent starts doing things that break your budget, that’s not a good thing. You need to balance automation with financial control.”
Data privacy and sovereignty are also major hurdles. AI agents trained on unvetted data sources may produce toxic or harmful outputs. Organizations must ensure their data is clean, secure, and compliant with regulations. Sanjeev notes, “Data privacy is non-negotiable. If your AI agent exposes sensitive information or mimics toxic behavior from unvetted data, it can lead to serious reputational and legal risks.”
Finally, monitoring and governance are essential to keep AI agents aligned with organizational goals. Continuous evaluation and feedback loops are necessary to catch and correct errors before they escalate. Sanjeev explains, “You can’t just deploy an AI agent and forget about it. You need constant monitoring, reinforcement learning, and human feedback to keep it on track.”
The Role of Data in AI Success
In the AI era, data is the ultimate differentiator. Organizations with high-quality, well-organized data can train AI agents to deliver more accurate and actionable insights. However, the challenge lies in balancing the urgency to adopt AI with the need to improve data quality.
Data is the foundation of AI success. Organizations with rich, high-quality data can train AI agents to deliver superior results, giving them a significant competitive advantage.”
As Sanjeev Mohan explains, “Data is the biggest moat for any organization. If you have high-quality data that’s unique to your business, no one can replicate that. It’s what gives you the edge in AI.”
One key consideration is the distinction between structured and unstructured data. While 80% of enterprise data is unstructured—like emails, PDFs, and Slack messages—structured data (e.g., databases) provides the critical linkages AI agents need to make informed decisions. Sanjeev highlights this: “Structured data is where you get the linkages. You can’t just rely on unstructured data like PDFs. You need to combine both to unlock the full potential of AI.”
However, organizations often struggle with data quality. Poor data can lead to inaccurate outputs and hallucinations, undermining the effectiveness of AI agents. Sanjeev advises, “You can’t wait for perfect data before adopting AI. Your competitors are already moving forward. The key is to recognize your data is imperfect but start experimenting anyway. Just be mindful of the risks.”
Human-AI Collaboration
AI agents are poised to take over routine tasks, freeing humans to focus on higher-value activities. Rather than replacing humans, AI agents will augment our capabilities, enabling us to focus on creativity, innovation, and strategic thinking.
AI agents are not here to replace humans—they’re here to augment our capabilities. By automating mundane tasks, they free us to focus on what we do best: creativity, innovation, and strategic thinking.
As Sanjeev Mohan explains, “AI is writing 25% of our code, but it gives us more time to think more strategically. All the time-consuming stuff—like checking syntax or debugging—is being automated, so we can focus on higher-value work.”
This shift mirrors past technological disruptions. For example, when ATMs were introduced, bank tellers didn’t disappear—they transitioned to roles like private client banking, where human interaction added value. Sanjeev draws a parallel: “When ATMs came, people worried about tellers losing their jobs. But tellers became private client bankers. The same will happen with AI—it will create new roles and opportunities.”
The future of work will likely involve a collaborative partnership between humans and AI agents. For instance, in research, AI agents can sift through vast amounts of data, identify patterns, and generate reports, freeing up human analysts to focus on strategic decision-making. Sanjeev emphasizes this: “The agent is my co-pilot, but I’m still the pilot. That may change in the future, but for now, humans are in control.”
Environmental and Regulatory Considerations
As AI agents become more widespread, organizations must address two critical issues: the environmental impact of AI systems and the evolving regulatory landscape.
Training and running AI models require significant energy, but advancements in efficiency—like smaller, more targeted models—are helping to reduce this footprint.
The energy demands of AI models and data centers are substantial. For example, training a single large language model can consume as much energy as hundreds of homes in a year. However, as Sanjeev Mohan notes, “The models are getting smarter and more efficient. We may not need tens of thousands of GPUs to train them in the future. But with more companies deploying AI agents, the overall energy usage will likely increase.”
On the regulatory front, AI-specific rules are still evolving, but existing data governance frameworks provide a solid foundation for responsible AI use. Sanjeev explains, “AI is not completely unregulated. There are already safeguards in place for data privacy and security. But as generative AI becomes more prevalent, we’ll see more specific regulations emerge.”
Organizations should focus on compliance with existing data privacy laws (e.g., GDPR) while staying adaptable to future AI regulations. Sanjeev advises, “Don’t wait for regulations to catch up. Start with the basics—clean your data, ensure privacy, and build a governance framework that can adapt to new rules.”
Key Recommendations for Business Leaders
Adopting AI agents requires a structured approach. Business leaders should focus on their unique data assets, experiment with AI in low-risk areas, and build a culture of curiosity and adaptability.
The best way to adopt AI agents is to start small. Identify a high-impact area, run a pilot, and measure the results before scaling up.
As Sanjeev Mohan advises, “Cut out the noise. Focus on your business imperatives and how AI can improve them. Don’t get bogged down by the latest trends or technologies.”
Here are four key recommendations for leaders:
- Focus on business objectives: Start by identifying one high-impact area, such as customer service, and pilot an AI agent to handle routine inquiries. Measure the impact on response times and customer satisfaction before scaling up.
- Experiment and iterate: Encourage a culture of experimentation. For example, set up a small team to explore AI agent frameworks and test them on low-risk tasks, like internal research or document summarization.
- Cut through the noise: Avoid getting distracted by the latest AI trends. Instead, focus on practical, data-driven solutions that align with your business goals.
- Invest in data quality: High-quality data is the foundation of effective AI agents. Invest in data governance and cleaning processes to ensure your AI systems have the inputs they need to deliver accurate results.
Sanjeev emphasizes the importance of a structured approach: “Don’t jump straight to technology. Start with your business objectives, do a cost-value analysis, and then decide if AI is the right solution.”
Conclusion
AI agents are transforming how businesses operate, but their adoption requires careful planning. From ensuring data quality to addressing reliability challenges, leaders must take a structured approach to unlock their full potential.
AI agents are not just a technological advancement—they’re a strategic opportunity. Leaders who embrace them thoughtfully will gain a significant competitive edge.
As Sanjeev Mohan reminds us, “AI is not a panacea for every problem. It’s a tool, and like any tool, it’s only as good as how you use it. Focus on your business objectives, experiment wisely, and invest in your data.”
The journey from daemons to RPA to chatbots has brought us to the next frontier: autonomous AI agents. By understanding their capabilities, addressing their challenges, and leveraging their potential, businesses can stay ahead in the AI era.
Call to Action:
“As AI agents continue to evolve, now is the time for leaders to act. Start small, experiment often, and focus on your unique data assets to drive competitive advantage in the AI era.”
Listen to the full conversation with Sanjeev Mohan on the
- Data Faces podcast: https://youtu.be/2fdMAsMQnP0
- Follow Sanjeev on LinkedIn: https://www.linkedin.com/in/sanjmo/
- Read more on his blog: https://medium.com/@sanjmo
Summarized Transcript – Data Faces Podcast with Sanjeev Mohan
[0:00] – Introduction
Host David Sweenor introduces the Data Faces Podcast and guest Sanjeev Mohan, Principal at SanjMo and former VP at Gartner. The discussion focuses on AI agents—their current landscape, challenges, and future.
[2:21] – Sanjeev’s Background
Sanjeev shares his journey from being a Gartner analyst to launching his own consultancy, SanjMo. He discusses his focus on emerging technologies and the rapid evolution of generative AI.
[4:08] – AI Agents Defined
Sanjeev explains AI agents as autonomous systems capable of understanding context, reasoning, planning, and executing tasks—far beyond simple chatbot interactions. He highlights their potential impact on automation and workflows.
[7:23] – AI Agents: Hype vs. Reality
AI agents are still in their early stages. While promising, they currently struggle with hallucinations and reliability. Enterprises should use AI agents for non-mission-critical tasks like research and competitive intelligence before scaling to larger use cases.
[10:33] – Key Challenges in AI Agent Adoption
- Reliability: Ensuring AI agents do not hallucinate or make faulty decisions.
- Cost: Managing expensive AI operations.
- Data Privacy & Compliance: Protecting sensitive information and ensuring responsible AI use.
[14:30] – The Role of Data in AI Agents
Sanjeev emphasizes that 80% of enterprise data is unstructured and often untapped. While structured data remains crucial, organizations must find ways to integrate and govern both data types effectively.
[17:50] – Governance and Guardrails for AI
Organizations must establish AI governance frameworks to mitigate risks and ensure compliance. Best practices include:
- Continuous monitoring of AI outputs.
- Implementing reinforcement learning with human feedback (RLHF).
- Evaluating multiple AI models for different use cases.
[22:22] – Human-AI Collaboration
AI will not replace humans but augment their capabilities. Just as past innovations (e.g., ATMs) reshaped job roles, AI will create new opportunities while automating repetitive tasks.
[27:14] – AI’s Environmental Impact
While AI efficiency is improving, demand is skyrocketing. The future of AI infrastructure may involve sustainable energy solutions like nuclear-powered data centers.
[29:13] – The Competitive Edge: Data is King
Since AI models are widely available, high-quality, proprietary data will be the ultimate differentiator for organizations.
[32:06] – Skills for the AI Era
Sanjeev stresses the importance of curiosity and adaptability over traditional hard skills. Organizations should prioritize hiring individuals who are eager to experiment and learn.
[35:30] – Advice for Business Leaders
Cut through the hype. Focus on business imperatives and evaluate AI use cases through structured cost-benefit analyses. AI should serve business goals, not vice versa.[38:14] – Closing Remarks
Sanjeev reflects on the evolving AI landscape and offers final insights for organizations looking to leverage AI agents effectively.