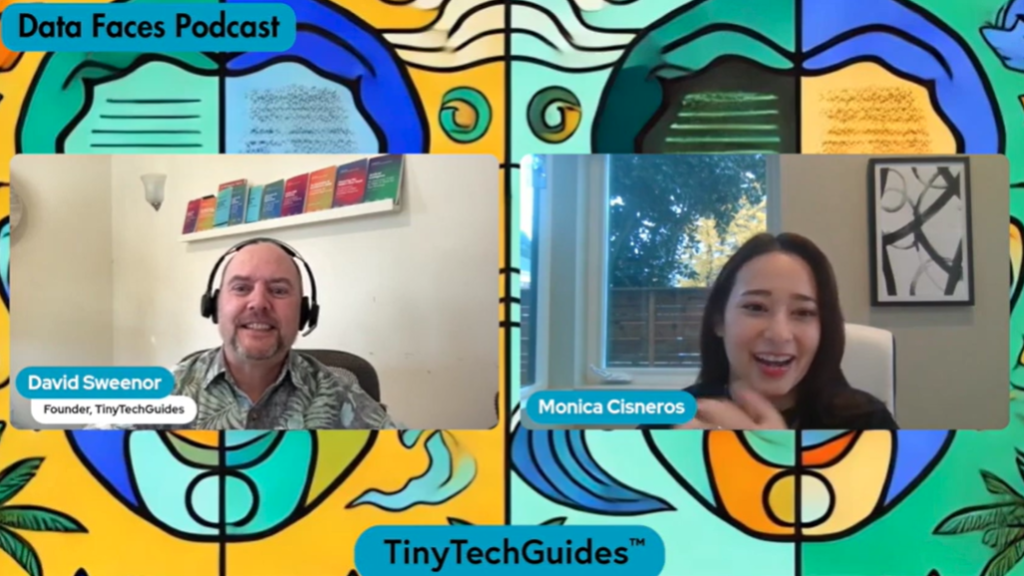
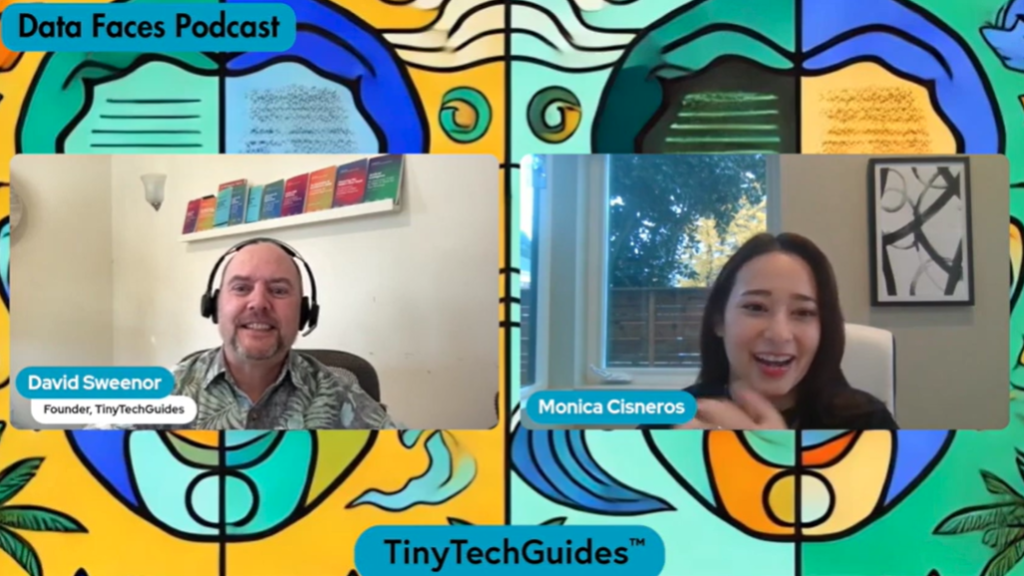
In the inaugural episode of Data Faces, I spoke with Monica Cisneros, Head of AI and Data Analytics at Fuerza Feminista. Monica has a unique background—from neuroscience research at Harvard to leading AI initiatives for analyzing sensitive data related to gender violence. Our discussion focused on pressing AI topics, particularly in the context of ethics, fairness, and leadership. Monica’s insights are invaluable for leaders navigating the complexities of AI.
The Complexity of AI Ethics
During the podcast, Monica reflected on a Stanford ethics course Ethics, Technology + Public Policy for Practitioners she recently finished. One of her key takeaways was recognizing that the ethical implications of AI are rarely black and white. For instance, AI can improve efficiency and decision-making (positive), displace jobs (negative), or have a mixed impact, such as automating mundane tasks but reducing certain skill development (neutral). This ambiguity reflects the nuanced nature of AI’s societal effects.
We also discussed a thought experiment from The Ones Who Walk Away from Omelas by Ursula K. Le Guin. In this story, the prosperity of a city relies on the suffering of a single child. Monica related this to the ethical complexities in AI: where do we draw the line between collective benefit and individual harm? How much are we willing to sacrifice for broader gains?
Our discussion highlighted critical takeaways for leaders—when it comes to AI ethics, decisions are rarely straightforward; there are varying shades of gray. Business leaders must recognize that ethical decision-making in AI involves trade-offs. Responsible AI leadership requires understanding these trade-offs and actively managing them while being transparent about the decisions made.
Fairness and the Leadership Dilemma
Monica and I explored the challenges of defining fairness in AI. There are 21 different mathematical definitions of fairness—each valid depending on the context. This raises a critical question: which definition should be used when deploying AI systems, and who decides? For leaders, navigating these fairness trade-offs involves concrete steps: clearly articulate why a particular fairness metric was chosen, communicate openly with stakeholders, and engage affected groups to mitigate negative impacts.
We also discussed the role of leadership evolution—how leaders start their careers with broader social good in mind but often become focused on business outcomes as they rise to senior positions. This evolution affects their decisions on fairness. The challenge lies in balancing these business metrics with social responsibility, and it is precisely within this tension that responsible leadership must operate.
Fairness in AI is not an endpoint—it’s an ongoing process of reflection, adjustment, and accountability.
The Evolution of Leadership in AI
Another significant theme was how leadership in AI evolves over time. Early-career professionals often enter the field driven by a desire to impact societal issues—such as climate change or ethical technology. As they rise through the ranks, their responsibilities expand, shifting their focus toward metrics tied to business health and operational success.
This shift is natural, shaped by the increasing scale of accountability that leaders face. Where early-career professionals work on projects, senior executives must ensure organizational stability. Responsible leadership in AI is about sustaining ethical choices while ensuring business success. Leaders must navigate pragmatism and purpose—recognizing opportunities for societal impact without compromising on the organization’s stability.
Lifting Underrepresented Voices
The final area we discussed was the importance of lifting underrepresented voices in tech. Monica highlighted that diverse leadership is crucial for shaping inclusive AI systems, as diverse teams bring richer experiences and perspectives to decision-making. We also discussed the different roles leaders can play—coaches, mentors, and sponsors:
- Coaches help individuals build skills, providing targeted feedback.
- Mentors guide individuals by sharing experiences.
- Sponsors actively advocate, using influence to open doors.
Monica emphasized that senior leaders must become sponsors for those often overlooked. Sponsorship goes beyond offering advice—it means leveraging influence to create real opportunities. Senior leaders should actively recommend diverse talent for key projects, provide visibility in critical meetings, and hold themselves accountable for promoting underrepresented voices.
A key takeaway from this part of the conversation was that fostering inclusivity in AI leadership is not only a matter of fairness; it is also a strategic advantage. By bringing diverse perspectives to the forefront, organizations can better anticipate risks, understand the full spectrum of their stakeholders, and develop AI systems that work equitably for everyone.
Conclusion
The conversation with Monica Cisneros highlighted some of the pressing challenges facing AI today—from ethical complexities to the evolving role of leadership and the importance of inclusivity. Leaders in AI must navigate ambiguity, balance business imperatives with societal good, and create opportunities for diverse voices. As a practical next step, consider initiating a fairness audit within your organization to understand how current AI practices align with ethical standards and what improvements can be made.
Call to Action
If these topics resonate with you, I encourage you to listen to the full podcast episode. Monica’s insights are not only thought-provoking but also actionable for anyone in a leadership position. How is your organization addressing the challenges of AI ethics, fairness, and inclusivity? Let’s continue the conversation on building ethical, fair, and inclusive AI. Listen here:
Transcript – Edited for Clarity
David Sweenor 0:01
Good morning, good afternoon, and good evening. Welcome to the Databases Podcast! I’m delighted to be joined by the magnificent Monica Cisneros. She’s a rising star in the data analytics and AI sphere and has extensive experience supporting clients around the world. Welcome to the show, Monica!
Monica Cisneros 0:18
Thank you, David. I’m super excited to be here, and I love the name Databases. It’s fantastic.
David Sweenor 0:24
Thank you, appreciate it. A quick introduction to Monica: she’s the Head of AI and Data Analytics at Forza Feminista—hope I pronounced that correctly. She’s also led product marketing for AI at Alteryx and served as a Solutions Consultant at TIBCO. We’ve had the chance to work together at some of these companies. A lesser-known fact about Monica is that she conducted neuroscience research at Harvard before transitioning to tech. Monica, tell us a bit more about your current role and the work you’re doing at Forza Feminista.
Monica Cisneros 0:59
Forza Feminista is an academic project led by two professors—one at UTSA and another at NMSU. They’re researching feminist sites in Ciudad Juárez, focusing on mothers who’ve lost their daughters to violence. The project explores how these traumatic events inspire advocacy and influence policy. My role involves transcription, sentiment analysis, and text analysis of interviews, with the goal of creating a digital exhibition to share these stories.
David Sweenor 1:49
That’s incredible. I commend you for applying your technical expertise to such meaningful work. The world needs more people using their skills for positive change. Now, Monica, let’s shift gears. We were texting recently about a class you took at Stanford on ethics, technology, and public policy. But before we get into that, I’d love to hear about your neuroscience research at Harvard. You once shared a funny story about the power going out. Can you share that with our listeners?
Monica Cisneros 2:34
Absolutely. I studied biology in college and was on track for a PhD—I even got into a program but didn’t go through with it. One reason was my love for animals. I hadn’t realized that basic research often involves working with animal tissue, especially mice, which are common models. That experience ultimately led me to leave academia.
One memorable (and terrifying) moment happened late one night in the lab. I was working in the animal facility, which was in the basement, and the lights suddenly went out. What many don’t know is that mice eyes reflect light in the dark. When I turned on my phone’s flashlight, I saw thousands of tiny, glowing eyes staring at me. It was terrifying! I was fumbling to get out, taking off protective equipment in total darkness, and I had to call for help. After that, I vowed never to stay in the lab past 7 PM.
Ultimately, the experience reinforced my decision to leave academia. It was also the beginning of my journey into tech, where my background in neuroscience became a foundation for my interest in AI, particularly neural networks.
David Sweenor 5:25
That’s both hilarious and horrifying. I’d have been scared out of my mind. I’m glad you’re now in a safer (and less creepy) environment! Let’s talk about the Stanford course. Ethics and AI is such a critical topic. What drew you to this class, and what was your experience like?
Monica Cisneros 5:50
I recently experienced a layoff, which gave me the opportunity to focus on learning and growth. I came across this course through a network of people in AI and public policy. The course, which is cohort-based, was about much more than just ethics—it centered around philosophy. It wasn’t about giving answers but about asking deeper questions.
For example, instead of just asking how to implement AI, we explored whether we should implement it in certain scenarios. What are the broader implications? What happens when decisions lead to unintended consequences? It challenged me to think beyond black-and-white outcomes and engage with the complexity of real-world issues.
David Sweenor 7:56
That’s fascinating. It sounds like the course pushed you to think critically and challenge assumptions. What were some of your key takeaways?
Monica Cisneros 8:09
One of the most surprising things I learned was that there are 21 mathematical definitions of fairness. As a technologist, this really struck me. For example, you can improve classification parity—equalizing error rates across groups—but that can negatively impact calibration, where the risk scores should be accurate across groups.
A case study we examined was COMPAS, a tool for predicting recidivism. While its predictions were 61% accurate overall, it was biased against Black defendants. It incorrectly labeled them as high-risk twice as often as white defendants and underestimated the risk for white defendants who did reoffend. This example highlighted the trade-offs between fairness metrics and the importance of scrutinizing AI systems in practice.
David Sweenor 10:10
It’s clear there’s no simple solution. Who do you think bears responsibility for ensuring fairness in AI—engineers, leaders, or everyone?
Monica Cisneros 10:37
It’s a shared responsibility. In the course, we heard from industry leaders, academics, and policymakers. Each emphasized the need for collaboration. Developers must critically evaluate their algorithms, but business leaders, NGOs, governments, and advocacy groups also play crucial roles in creating ethical frameworks and holding systems accountable.
David Sweenor 20:42
That’s such an important perspective. Let’s switch gears for a moment. In class, you also mentioned a utopian story called The Ones Who Walk Away from Omelas. What impact did that have on you?
Monica Cisneros 15:03
The story portrays a utopia where everyone lives in abundance, but their happiness depends on the suffering of one child. It raises questions about complicity and moral trade-offs. At the start of the course, I was struggling personally and felt I couldn’t take on more responsibility for addressing systemic issues. The story helped me recognize that sometimes we need to focus on self-care before we can help others. By the end of the course, I felt more hopeful and re-energized to contribute.
David Sweenor 48:46
Monica, it’s been an absolute pleasure having you on the podcast. Your insights on AI ethics and fairness have been thought-provoking. Thank you for sharing your journey and expertise with us.
Monica Cisneros 55:56
Thank you, David. I had a great time. This was such a fun and meaningful conversation!